Abstract
Estimation errors or uncertainities in expected return and risk measures create difficulties for portfolio optimization. The literature deals with the uncertainty using stochastic, fuzzy or probability programming. This paper proposes a new approach to treating uncertainty. By assuming that the expected return and risk vary within a bounded interval, this paper uses interval analysis to extend the classical mean-variance portfolio optimization problem to the cases with bounded uncertainty. To solve the interval quadratic programming problem, the paper adopts order relations to transform the uncertain programme into a deterministic programme, and includes the investors’ risk preference into the model. Numerical analysis illustrates the advantage of this new approach against conventional methods.


Similar content being viewed by others
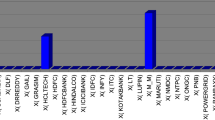
References
Alolyan I (2011). A new method for comparing closed intervals. The Australian Journal of Mathematical Analysis and Application 8 (1): 1–6.
Ammar EE (2008). On solutions of fuzzy random multiobjective quadratic programming with applications in portfolio problem. Information Science 178 (2): 468–484.
Aouni B and Torre DL (2010). A generalized stochastic goal programming model. Applied Mathematics and Computation 215 (12): 4347–4357.
Bhattacharyya R, Kar S and Majumder DD (2011). Fuzzy mean-variance-skewness portfolio selection models by interval analysis. Computers & Mathematics with Application 61 (1): 126–137.
Charnes A and Cooper WW (1959). Chance-constrained programming. Management Science 6 (1): 73–79.
Chinneck JW and Ramadan K (2000). Linear programming with interval coefficients. Journal of Operational Research Society 51 (2): 209–220.
Delgado M, Verdegay JL and Vila MA (1989). A general model for fuzzy linear programming. Fuzzy Sets and Systems 29 (1): 21–29.
Fang Y, Lai KK and Wang SY (2006). Portfolio rebalancing model with transaction costs based on fuzzy decision theory. European Journal of Operational Research 175 (2): 879–893.
Giove S, Funari S and Nardelli C (2006). An interval portfolio problem based on regret function. European Journal of Operational Research 170 (1): 253–264.
Hladík M (2008). Tolerances in portfolio selection via interval linear programming. In: Rehorova P, Marsikova K and Hubinka Z (eds). CD-ROM Proceedings 26-th Int. Conf. Mathematical Methods in Economics MME08; Liberec, Czech Republic, pp 185–191.
Hladík M (2011). Optimal value bounds in nonlinear programming with interval data. TOP 19 (1): 93–106.
Hladík M (2012). Interval linear programming: A survey. In: Mann ZA (ed). Linear Programming-New Frontiers in Theory and Applications. Nova Science Publishers: New York, pp 85–120.
Huang GH and Cao MF (2011). Analysis of solution methods for interval linear programming. Journal of Environmental Informatics 17 (2): 54–64.
Ida M (2003). Portfolio selection problem with interval coefficients. Applied Mathematics Letters 16 (3): 709–713.
Ida M (2004). Solutions for the portfolio selection problem with interval and fuzzy coefficients. Reliable Computing 10 (5): 389–400.
Inuiguchi M and Sakawa M (1995). Minimax regret solution to linear programming problems with an interval objective function. European Journal of Operational Research 86 (3): 526–536.
Ishibuchi H and Tanaka H (1990). Multiobjective programming in optimization of the interval objective function. European Journal of Operational Research 48 (2): 219–225.
Kall P (1982). Stochastic programming. European Journal of Operational Research 10 (2): 125–130.
Karmakar S and Bhunia AK (2012). A comparative study of different order relations of intervals. Reliable Computing 16: 38–72.
Karmakar S and Bhunia AK (2014). Uncertain constrained optimization by interval-oriented algorithm. Journal of the Operational Research Society 65 (1): 73–877.
Karmakar S, Mahato SK and Bhunia AK (2009). Interval oriented multi-section techniques for global optimization. Journal of Computation and Applied Mathematics 224 (2): 476–491.
Lai KK, Wang SY, Xu JP, Zhu SS and Fang Y (2002). A class of linear interval programming problems and its application to portfolio selection. IEEE Transactions on Fuzzy Systems 10 (6): 698–704.
Li J and Xu JP (2009). A novel portfolio selection model in a hybrid uncertain environment. Omega 37 (2): 439–449.
Li W and Tian XL (2008). Numerical solution method for general interval quadratic programming. Applied Mathematics and Computation 202 (2): 589–595.
Liu ST (2011). The mean-absolute deviation portfolio selection problem with interval-valued returns. Journal of Computational and Applied Mathematics 235 (14): 4149–4157.
Liu S-T and Wang R-T (2007). A numerical solution method to interval quadratic programming. Applied Mathematics and Computation 189 (2): 1274–1281.
Liu XW and Da QL (1999). A satisfactory solution for interval number linear programming. Journal of Systems Engineering 14 (2): 123–128.
Liu YJ, Zhang WG and Zhang P (2013). A multi-period portfolio optimization model by using interval analysis. Economic Modelling 33: 113–119.
Moore RE (1979). Method and Application of Interval Analysis. SIAM: Philadelphia.
Ratschek H and Rokne J (1988). New Computer Methods for Global Optimization. Ellis Horwood Ltd.: Chichester.
Rohn J (2006). Interval linear programming. In: Fiedler M, Rohn J, Nedoma J, Ramík J and Zimmermann K (eds). Linear Optimization Problems with Inexact Data. Springer: New York, pp 79–100.
Rommelfanger H (1989). Interactive decision making in fuzzy linear optimization problems. European Journal of Operational Research 41 (2): 210–217.
Sahoo L, Bhunia AK and Kapur PK (2012). Genetic algorithm based multi-objective reliability optimization in interval environment. Computers & Industrial Engineering 62 (1): 152–160.
Sengupta A and Pal TK (2000). On comparing interval numbers. European Journal of Operational Research 127 (1): 28–43.
Sengupta A and Pal TK (2009). Fuzzy Preference Ordering of Interval Numbers in Decision Problems. Springer: India.
Sengupta A, Pal TK and Chakraborty D (2001). Interpretation of inequality constraints involving interval coefficients and a solution to interval linear programming. Fuzzy Sets and Systems 119 (1): 129–138.
Shi Y, Wu F, Chu LK, Sculli D and Xu YH (2010). A portfolio approach to managing procurement risk using multi-stage stochastic programming. Journal of the Operational Research Society 62 (11): 1958–1970.
Tong SC (1994). Interval number and fuzzy number linear programmings. Fuzzy Sets and Systems 66 (3): 301–306.
Vajda S (1972). Probabilistic Programming. Academic Press: New York.
Acknowledgements
We are thankful for support from the No.71272160 and No.71172011 project of National Natural Science Foundation of China, No. NCET-12-0772 Program for New Century Excellent Talents in University of Ministry of Education of China and No. FRF-TP-09-022. A project of the Fundamental Research Funds for the Central Universities, this paper is finished as expected. We are also grateful to the anonymous referees for their professional comments and suggestions, which have greatly improved upon the earlier version of this paper.
Author information
Authors and Affiliations
Rights and permissions
About this article
Cite this article
Xu, X., He, F., Chen, R. et al. Solving non-linear portfolio optimization problems with interval analysis. J Oper Res Soc 66, 885–893 (2015). https://doi.org/10.1057/jors.2014.31
Received:
Accepted:
Published:
Issue Date:
DOI: https://doi.org/10.1057/jors.2014.31