Abstract
Methotrexate (MTX) is a common first-line treatment for new-onset rheumatoid arthritis (RA). However, MTX is ineffective for 30–40% of patients and there is no way to know which patients might benefit. Here, we built statistical models based on serum lipid levels measured at two time-points (pre-treatment and following 4 weeks on-drug) to investigate if MTX response (by 6 months) could be predicted. Patients about to commence MTX treatment for the first time were selected from the Rheumatoid Arthritis Medication Study (RAMS). Patients were categorised as good or non-responders following 6 months on-drug using EULAR response criteria. Serum lipids were measured using ultra‐performance liquid chromatography–mass spectrometry and supervised machine learning methods (including regularized regression, support vector machine and random forest) were used to predict EULAR response. Models including lipid levels were compared to models including clinical covariates alone. The best performing classifier including lipid levels (assessed at 4 weeks) was constructed using regularized regression (ROC AUC 0.61 ± 0.02). However, the clinical covariate based model outperformed the classifier including lipid levels when either pre- or on-treatment time-points were investigated (ROC AUC 0.68 ± 0.02). Pre- or early-treatment serum lipid profiles are unlikely to inform classification of MTX response by 6 months with performance adequate for use in RA clinical management.
Similar content being viewed by others
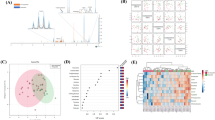
Introduction
Without early and effective treatment rheumatoid arthritis (RA) can lead to irreversible joint damage and poor long-term outcomes, including work disability and risk of premature mortality1. The treatment pathway for RA starts with synthetic disease-modifying anti-rheumatic drugs (scDMARDs), including methotrexate (MTX)2. If disease symptoms are not controlled effectively by MTX, patients are then escalated to more expensive biologic drugs or targeted small molecule inhibitors3,4. However, regardless of the class of treatment, each drug comes with a significant non-response rate and it is currently not possible to predict which patient will benefit from treatment with a particular drug5.
Although MTX has been licensed for use in RA for more than two decades, few demographic and clinical associations with MTX response have been established. A positive rheumatoid factor titre, high health assessment questionnaire (HAQ) score, a high number of tender joints and higher depression and anxiety before starting on MTX are predictive of non-response after 6 months on drug6. However, these factors do not predict MTX non-response perfectly and it is likely that other disease- or patient-related factors also influence treatment outcome. For example, the application of genetic, transcriptomic and proteomic technologies to RA has expanded our understanding of important pathophysiological processes underpinning disease development, progression and response to treatment5.
Metabolomics and lipidomics are emergent approaches for studying pathophysiology and patient stratification in RA. Such profiling of biofluids from RA patients has the potential to identify disease processes that underpin important clinical outcomes. For example, serum lipids have been reported to correlate with response to TNFi in RA7,8. However, metabolomics studies of MTX response in RA have so far been limited to fewer than 40 patients9 and, to our knowledge, no lipidomic specific study applying machine learning algorithms to predict MTX treatment response in RA exists in the literature. The aim of this study was thus to determine if future MTX response can be predicted from ultra-performance liquid chromatography–mass spectrometry (UPLC-MS) derived serum lipidomic data by applying state-of-the-art machine learning methods, under robust nested cross-validation.
Methods
Patients and methods
In total, 100 patients were recruited from the Rheumatoid Arthritis Medication Study (RAMS) cohort, a multi-centre 1-year prospective observational study investigating predictors of response to MTX in UK patients with RA. To be eligible for RAMS patients must be over the age of 18 years and have a physician diagnosis of RA or undifferentiated polyarthritis. Since 2009, patients who are about to start MTX for the first time are asked to provide demographic, clinical and psychological data as well as blood samples to permit DNA, RNA and serum-based biomarker studies. Once collected and processed, serum samples were stored in barcode-labelled tubes at − 80 °C and recorded on a fully integrated laboratory management system. Serum was collected from patients at the baseline (pre-treatment) visit and following 4 weeks on treatment. Ethical approval for this study was obtained from the Central Manchester NHS Research Ethics Committee (reference 08/H1008/25), all patients provided written informed consent and all methods were performed in accordance with relevant guidelines and regulations.
Clinical assessments
Patients were seen by a research nurse prior to commencement of MTX. The baseline clinical assessment included collection of patient demographics and a 28-joint count disease activity score (DAS28)10 was measured. Dose of MTX and MTX start date was also recorded. Patients complete a questionnaire including the HAQ. Similar patient-centred data were collected at 6 months following initiation of MTX therapy. The DAS28 was calculated again at 6 months and established EULAR response criteria11 were applied to categorize patients into good (n = 50) or non-responders (n = 50).
Lipid profiling
Serum samples were analysed using ultra-performance liquid chromatography–mass spectrometry (UPLC–MS) following previously described sample preparation, analytical, and quality control (QC) procedures12,13. Each set of sample analyses were performed in an order designed to be orthogonal to clinical and demographic data of potential significance with respect to the study design and outcome. Serum samples were prepared as previously described 12 for the separation of lipophilic analytes (e.g., complex and neutral lipids) by reversed-phase chromatography (lipid RPC). For quality control and pre-processing, a pooled QC sample was prepared by combining equal parts (25 μL) of each study sample. In brief, 50 μL aliquots were taken from each sample and the pooled QC and diluted 1:1 v/v with ultrapure water. Protein was removed by addition of organic solvent (diluted sample/isopropanol in 1:4 v/v ratio) and a mixture of method-specific authentic chemical standards were added at the protein precipitation step in order to monitor and evaluate data quality during and after the acquisition respectively.
Serum analyses were performed on ACQUITY UPLC instruments (Waters Corp., Milford, MA, USA) coupled to Xevo G2‐S Q-TOF mass spectrometers (Waters Corp., Manchester, UK) via a Z‐spray electrospray ionisation (ESI) source operating in both positive and negative ion modes to generate lipid positive (lipid RPC+) and negative (lipid RPC−) datasets. Lipid separation was conducted using a 2.1 × 100 mm BEH C8 column (Waters Corp., Milford, MA, USA) (further details on the UPLC method and MS parameters can be found in Izzi-Engbeaya et al.12). Pooled QC samples were acquired at 10 study sample intervals during sample analyses. An additional set of QC sample dilutions was created from the pooled QC (10 × 100%, 5 × 80%, 3 × 60%, 3 × 40%, 5 × 20%, 10 × 1%) and analysed at the start and end of each set of sample analyses for assessment of analyte response.
Raw data was converted to the mzML open-source format and signals below an absolute intensity threshold of 100 counts were removed using the MSConvert tool in ProteoWizard14.
Feature extraction was performed in XCMS15 and in-house scripts16 applied for elimination of potential run-order effects and feature filtering. Run-order correction was applied at the feature level by fitting a LOESS estimator across intensities of consecutive pooled QC samples and applying to intervening study samples, following an adapted version of the approach proposed by Dunn et al.17. Features were filtered to retain only those measured with high analytical quality (relative standard deviation (RSD) in pooled QC < 30%, pooled QC dilution series Pearson correlation to dilution factor > 0.7, RSD in study samples > 1.1* RSD in pooled QC) were retained and put forward for further statistical analysis.
Targeted interrogation of lipids of interest from previous publications7,8,9 was performed in both lipid RPC+ and lipid RPC− datasets. Cholesterol was annotated by matching both retention time and accurate mass to an authentic chemical standard and another four lipids (sn1-LPC 18:3/0:0, sn1-LPC 15:0/0:0, C24H52NO6P and C18H34O2) were tentatively annotated by matching the accurate mass and isotopic pattern/chemical formula of the annotation provided in the publication as no chemical reference standards were available.
Statistical analysis
Assessment of data complexity
Lipid levels were normalised using probabilistic quotient normalization (PQN) before hierarchical cluster analysis. Finite normal mixture modelling and heat maps were used to assess data complexity. The distance matrix required for hierarchical clustering was produced using the complete linkage method (furthest neighbour).The Pearson method was used to compute the correlation matrix required to produce the heat map. Gaussian mixture models, fitted by the expectation–maximization algorithm, were assessed using the Bayesian Information Criterion (BIC).
Regression analysis
Linear mixed effect models were developed to detect correlation between individual lipid levels and response to MTX, incorporating the pre-treatment and 4-week sample. Fixed effects included clinical and demographic parameters (i.e. patient sex, age-at-inclusion, MTX start dose, BMI and smoking history) and a patient identifier was included as a random effect. To determine if lipid effects on future treatment response were time-point dependent, an interaction term was specified between time-point and EULAR response. The false discovery rate (FDR) was used to control for Type 1 error at the 5% threshold.
Prediction
Machine learning models were built to classify MTX response at 6 months using serum lipid profiles at pre-treatment, following 4 weeks on drug and by taking the difference in serum lipid levels between the 4-week and pre-treatment sample. Models including metabolite levels were compared to models including the following clinical parameters: MTX start dose, steroid use at inclusion, BMI, number of swollen joints, number of tender joints, CRP levels, patients’ assessment of their overall wellbeing, sex, age-at-inclusion, age-at-onset, disease duration, HAQ score and pre-treatment smoking habits. For each contrast, we employed three state-of-the-art machine learning methods: random forest, a kernel-based approach (i.e. radial kernel support vector machine) and regularized logistic regression, a strategy that uses a loss function designed to drop the features that are not predictive. Each model was run under a fivefold nested cross-validation scheme (where hyper-parameters were computed in each of the strata using an inner fivefold cross validation loop, with the default tuneGrid parameters in the caret library18, and a tuneLength of 100) to give accurate estimates of predicted performance. Model performance is reported using receiver operating characteristic (ROC) curves; the average ROC area under the curve (AUC) is reported along with the standard errors of the mean (± SE). All ROC AUCs reported throughout were calculated using the out-of-sample cross-validation folds.
Results
Samples
Following QC, 3,366 features (1060 in negatively-charged mode and 2306 in positive mode) were available for analysis at pre-treatment and 4 weeks from 100 RA patients categorised as good- (GR, n = 50) or non- (NR, n = 50) responders to MTX following 6 months on drug (Table 1).
Data complexity and linear mixed models
No evidence of patient clustering was observed in either the PCA analysis (Supplementary Figure S1), by assessing pair-wise correlation, or using a model-based clustering approach (Supplementary Figures S2, S3). Hierarchical cluster analysis revealed less dissimilarity in within-patient samples (across time-points) compared to between-patient samples (Supplementary Figure S4); median within-patient Pearson correlation = 0.58 (25th and 75th percentile = 0.37 and 0.68, respectively). Linear mixed effects models tested the association between individual lipid levels and EULAR response measured at 6 months. No lipid tested was individually associated with response to treatment (at FDR < 5%). An interaction was specified between sampling time-point and EULAR response to test if the difference in lipid level between responders depended on time-point. No evidence for interaction was observed (at FDR < 5%).
Classifier performance
In the models based on lipidomic data very limited predictive utility was observed in either the pre-treatment or 4-week sample or by taking the difference in lipid levels between 4-week and pre-treatment; best performance was observed at the post-treatment time-point for L1/L2-regularised regression models (linear method: ROC AUC 0.61 ± 0.02, Fig. 1). Limited predictive utility was observed in the models based on the clinical data alone, at either baseline or 3 months (Fig. 1). However, the clinical models performed better than the models based on lipid levels.
Average ROC AUCs across the cross-validation runs from three machine learning methods including: regularised regression, random forest and a pathway‐supported approach described previously (15). The three methods are labelled linear, non-linear and kernel-based, respectively. Clinical only: performance of models based on clinical data only, recorded at baseline and following 3 months on drug. Metabolomics with clinical: performance of models based on clinical variables plus lipid levels measured at baseline following 4 weeks on drug, and using the ratio of lipid levels between 4 weeks and baseline. Metabolomics without clinical: performance of models based on lipid levels only.
Discussion
In this study, one of the largest lipidomic studies of MTX response in RA to date, we applied advanced statistical methods to serum lipidomic profiling data generated at pre-treatment and following 4 weeks on drug in patients receiving MTX for their RA. By assessing the various methods used, we conclude that serum lipid levels present at pre-treatment or during early treatment are unlikely to be useful for classifying future response to MTX in the setting of routine clinical care. None of the metabolites tested were associated with MTX response when tested individually or when considered together by taking a machine learning approach.
To our knowledge, the previous largest metabolomics study of MTX response included 38 patients (25 good-responders and 13 non-responders)9. In that small study, on-treatment serum levels of 11 metabolites were found to co-vary with MTX response, measured at the same time-point (24 weeks). Larger and more recent metabolomics study, which included a targeted lipidomic approach, have focussed on TNF-inhibitor (TNFi) treatment response in RA. For example, Cuppen et al.7 investigated pre-treatment serum metabolites as potential predictors of TNFi response in 105 RA patients (55 good-responders and 50 non-responders at 6-months). In that study, four metabolites, selected from 139 analysed, improved classification of response (average ROC-AUC 0.84), compared to models including clinical data only (average ROC-AUC 0.72). A study by Tatar et al.8 analysed pre-treatment plasma samples from 140 RA patients (100 good-responders and 40 non-responders at 6-months). In our lipid-focussed study we were able to extract peaks for one of the 11 serum metabolites in the study by Wang (cholesterol); two of the four metabolites found to improve classification of response to TNFi in the Cuppen et al. study (sn1-LPC 18:3/0:0 and sn1-LPC 15:0/0:0), and two metabolites detected in the study by Tatar et al. (C24H52NO6P and C18H34O2). None of these metabolites were associated with MTX response in the current study (Supplementary Table S1). Although these findings require replication in independent samples, the fact that these were not found to improve classification of response to MTX in our study implies either a context (i.e. assessment of a later time-point is needed e.g. 24-weeks) or drug specific (i.e. TNFi rather than MTX) utility.
The strengths of the current study include a large sample size (n = 100), robust acquisition of lipidomic data at two time-points (pre-treatment and also during early treatment), rigorous internal model validation by nested cross-validation, a focus on a single drug and profiling of a large number of features (n = 3,366). A limitation to the current study is that modest differences in MTX start dose were observed between responder groups at baseline. However, MTX start dose, along with other important clinical features, was adjusted for in the regression analysis to minimise any potential effects. We do not think modest differences in start dose resulted in altered lipid profiles. If this were the case we would have observed differences when classifying response using models that were built using lipid levels as input. A further limitation is random sampling. Serum samples were taken as part of routine clinical care; therefore, trough sampling was not possible and patients were not asked to fast prior to sample collection. A further limitation was that the on-treatment sampling time-point was arbitrarily decided. As discussed above, serum metabolites have been observed to co-vary with MTX response when later time-points are assessed (i.e. 24-weeks)9. It could be that a later time-point may have revealed greater differences than the 4-week sample assessed here. However, the sampling strategy in the current study is in kee** with that performed as part of routine clinical care and the 4-week time-point was chosen because early classification of likely response to MTX was a study aim. Nonetheless, we cannot exclude that better performance may have been achieved if samples were taken at a different time or preprandial following overnight fast. Alternatively, it could be that the lipidome is simply not substantially altered during early MTX therapy for RA. A more broad-scale metabolomics approach might be necessary to improve prediction performance, or a different approach, for example targeting proteomic biomarkers, may be warranted.
Conclusions
In conclusion, these data do not support the utility of early treatment lipidomic monitoring in routine clinical practice in patients started on MTX for their RA.
Data availability
De-identified lipidomics data presented in this manuscript are available via Mendeley data using the following link: https://data.mendeley.com/datasets/732n8426y5/1.
References
Plant, D., Wilson, A. G. & Barton, A. Genetic and epigenetic predictors of responsiveness to treatment in RA. Nat. Rev. Rheumatol. 10, 329–337 (2014).
Barrera, P. et al. Drug survival, efficacy and toxicity of monotherapy with a fully human anti-tumour necrosis factor-alpha antibody compared with methotrexate in long-standing rheumatoid arthritis. Rheumatology (Oxford) 41, 430–439 (2002).
Singh, J. A. et al. 2015 American College of Rheumatology Guideline for the treatment of rheumatoid arthritis. Arthritis Rheumatol. 68, 1–26 (2016).
Smolen, J. S. et al. EULAR recommendations for the management of rheumatoid arthritis with synthetic and biological disease-modifying antirheumatic drugs: 2016 update. Ann. Rheum. Dis. 76, 960–977 (2017).
Plant, D. & Barton, A. Adding value to real-world data: The role of biomarkers. Rheumatology 59(1), 31–38 (2020).
Sergeant, J. C. et al. Prediction of primary non-response to methotrexate therapy using demographic, clinical and psychosocial variables: Results from the UK Rheumatoid Arthritis Medication Study (RAMS). Arthritis Res. Ther. 20, 147 (2018).
Cuppen, B. V. J. et al. Exploring the inflammatory metabolomic profile to predict response to TNF-α inhibitors in rheumatoid arthritis. PLoS One 11(9), e0163087 (2016).
Tatar, Z. et al. Variations in the metabolome in response to disease activity of rheumatoid arthritis. BMC Musculoskelet. Disord. 17, 20 (2016).
Wang, Z. et al. 1H NMR-based metabolomic analysis for identifying serum biomarkers to evaluate methotrexate treatment in patients with early rheumatoid arthritis. Exp. Ther. Med. 4, 165–171 (2012).
Home of the Disease activity score and DAS28. https://www.das-score.nl/das28/en/.
DAS28: EULAR response criteria. (2017). http://www.das-score.nl/das28/en/difference-between-the-das-and-das28/importance-of-das28-and-tight-control/eular-response-criteria.html.
Izzi-Engbeaya, C. et al. The effects of kisspeptin on β-cell function, serum metabolites and appetite in humans. Diabetes. Obes. Metab. 20, 2800–2810 (2018).
Lewis, M. R. et al. Development and application of ultra-performance liquid chromatography-TOF MS for precision large scale urinary metabolic phenoty**. Anal. Chem. 88, 9004–9013 (2016).
Chambers, M. C. et al. A cross-platform toolkit for mass spectrometry and proteomics. Nat. Biotechnol. 30, 918–920 (2012).
Smith, C. A., Want, E. J., O’Maille, G., Abagyan, R. & Siuzdak, G. XCMS: Processing mass spectrometry data for metabolite profiling using nonlinear peak alignment, matching, and identification. Anal. Chem. 78, 779–787 (2006).
Sands, C. J. et al. The nPYc-Toolbox, a Python module for the pre-processing, quality-control and analysis of metabolic profiling datasets. Bioinformatics 35, 5359–5360 (2019).
Dunn, W. B. et al. Procedures for large-scale metabolic profiling of serum and plasma using gas chromatography and liquid chromatography coupled to mass spectrometry. Nat. Protoc. 6, 1060–1083 (2011).
Kuhn, M. Building predictive models in R using the caret package. J. Stat. Softw. 28, 1–26 (2008).
Acknowledgements
This work was supported by the Medical Research Council (MRC) and Versus Arthritis (Grant codes 20385 and 20380), which jointly funded MATURA (Grant codes MR/K015346/1 and 20670, respectively). We thank MATURA consortium members for their input and feedback during the design of this project. A full list of RAMS and MATURA co-investigators can be found in the supplementary information. This research was supported by the NIHR Manchester Biomedical Research Centre. This work was further supported by the Medical Research Council and National Institute for Health Research [grant number MC_PC_12025] and infrastructure support was provided by the National Institute for Health Research (NIHR) Imperial Biomedical Research Centre (BRC). The views expressed are those of the author(s) and not necessarily those of the NHS, the NIHR or the Department of Health.
Author information
Authors and Affiliations
Contributions
C.S. and M.L.—acquisition of data, analysis, interpretation of data and drafting manuscript. M.M. and D.Z.—analysis, interpretation of data and drafting manuscript. S.L. and N.N.—acquisition of data, interpretation of data and drafting manuscript. S.V., K.H. and A.B. —design of study, interpretation of data and drafting manuscript. D.P.—design of study, analysis, interpretation of data and drafting manuscript.
Corresponding author
Ethics declarations
Competing interests
Drs. Maciejewski and Ziemek are full‐time employees of Pfizer and own stock or stock options in Pfizer. Drs Plant, Sands, Nair, Ling, Verstappen, Lewis and Professors Hyrich and Barton declare no potential competing interest.
Additional information
Publisher's note
Springer Nature remains neutral with regard to jurisdictional claims in published maps and institutional affiliations.
Supplementary Information
Rights and permissions
Open Access This article is licensed under a Creative Commons Attribution 4.0 International License, which permits use, sharing, adaptation, distribution and reproduction in any medium or format, as long as you give appropriate credit to the original author(s) and the source, provide a link to the Creative Commons licence, and indicate if changes were made. The images or other third party material in this article are included in the article's Creative Commons licence, unless indicated otherwise in a credit line to the material. If material is not included in the article's Creative Commons licence and your intended use is not permitted by statutory regulation or exceeds the permitted use, you will need to obtain permission directly from the copyright holder. To view a copy of this licence, visit http://creativecommons.org/licenses/by/4.0/.
About this article
Cite this article
Maciejewski, M., Sands, C., Nair, N. et al. Prediction of response of methotrexate in patients with rheumatoid arthritis using serum lipidomics. Sci Rep 11, 7266 (2021). https://doi.org/10.1038/s41598-021-86729-7
Received:
Accepted:
Published:
DOI: https://doi.org/10.1038/s41598-021-86729-7
- Springer Nature Limited