Abstract
The quality of stored minced pork meat was monitored using a rapid multispectral imaging device to quantify the degree of spoilage. Bacterial counts of a total of 155 meat samples stored for up to 580 h have been measured using conventional laboratory methods. Meat samples were maintained under two different storage conditions: aerobic and modified atmosphere packages as well as under different temperatures. Besides bacterial counts, a sensory panel has judged the spoilage degree of all meat samples into one of three classes. Results showed that the multispectral imaging device was able to classify 76.13 % of the meat samples correctly according to the defined sensory scale. Furthermore, the multispectral camera device was able to predict total viable counts with a standard error of prediction of 7.47 %. It is concluded that there is a good possibility that a setup like the one investigated will be successful for the detection of spoilage degree in minced pork meat.






Similar content being viewed by others
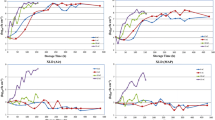
References
Ammor, S. A., Argyri, A., & Nychas, G.-J. E. (2009). Rapid monitoring of the spoilage of minced beef stored under conventionally and active packaging conditions using Fourier transform infrared spectroscopy in tandem with chemometrics. Meat Science, 81, 507–514.
Argyri, A. A., Panagou, E. Z., Tarantilis, P. A., Polysiou, M., & Nychas, G.-J. E. (2010). Rapid qualitative and quantitative detection of beef fillets spoilage based on Fourier transform infrared spectroscopy data and artificial neural networks. Sensors an Actuators B: Chemical, 145, 146–154.
Baranyi, J., & Roberts, T. A. (1994). A dynamic approach to predicting bacterial growth in food. International Journal of Food Microbiology, 23, 277–294.
Brooks, J. C., Alvarado, M., Stephens, T. P., Kellermeier, J. D., Tittor, A. W., & Miller, M. F. (2008). Spoilage and safety characteristics of ground beef packaged in traditional and modified atmosphere packages. Journal of Food Protection, 71, 293–301.
Byun, J. S., Min, J. S., Kim, I. S., Kim, J. W., Chung, M. S., & Lee, M. (2003). Comparison of indicators of microbial quality of meat during aerobic cold storage. Journal of Food Protection, 66, 1733–1737.
Carstensen, J. M., & Hansen, J. F. (2003). An apparatus and a method of recording an image of an object. Patent family EP1051660, Issued in 2003.
Carstensen, J. M., Hansen, M. E., Lassen, N. K., & Hansen, P. W. (2006). Creating surface chemistry maps using multispectral vision technology. In B. Ersbøll, T. M. Jørgensen (Eds.), 9th medical image computing and computer assisted intervention (MICCAI)—workshop on biophotonics imaging for diagnostics and treatment, Institute of Mathematical Modelling. Technical Report-2006-17. Kgs. Lyngby: IMM.
Chevallier, S., Bertrand, D., Kohler, A., & Courcoux, P. (2006). Application of PLS-DA in multivariate image analysis. Journal of Chemometrics, 20, 221–229.
Clemmensen, L. H., Hansen, M. E., Ersbøll, B. K., & Frisvad, J. C. (2007). A method for comparison of growth media in objective identification of Penicillium based on multi-spectral imaging. Journal of Microbiological Methods, 69, 249–255.
Cohen, J. (1960). A coefficient of agreement for nominal scales. Educational and Psychological Measurement, 20, 37.
Dainty, R. H. (1996). Chemical/biochemical detection of spoilage. International Journal of Food Microbiology, 33, 19–34.
Daugaard, S. B., Adler-Nissen, J., & Carstensen, J. M. (2010). New vision technology for multidimensional quality monitoring of continuous frying of meat. Food Control, 21, 626–632.
Dissing, B. S., Clemmesen, L. H., Løje, H., Ersbøll, B. K., &Adler-Nissen, J. (2009). Temporal reflectance changes in vegetables. Proceedings of Institute of Electrical and Electronics Engineers (IEEE) color and reflectance in imaging and computer vision workshop, Kyoto, Japan.
Doulgeraki, A. I., Paramithiotis, S., Kagkli, D. M., & Nychas, G.-J. E. (2010). Lactic acid bacteria population dynamics during minced beef storage under aerobic or modified atmosphere packaging conditions. Food Microbiology, 27, 1028–1034.
Ellis, D. I., & Goodacre, R. (2001). Rapid and quantitative detection of the microbial spoilage of muscle foods: current status and future trends. Trends in Food Science and Technology, 12, 414–424.
Ellis, D. I., Broadhurst, D., Kell, D. B., Rowland, J. J., & Goodacre, R. (2002). Rapid and quantitative detection of the microbial spoilage of meat by Fourier transform infrared spectroscopy and machine learning. Applied and Environmental Microbiology, 68, 2822–2828.
Ellis, D. I., Broadhurst, D., & Goodacre, R. (2004). Rapid and quantitative detection of the microbialspoilage of beef by Fourier transforminfraredspectroscopy and machine learning. Analytica Chimica Acta, 514, 193–201.
Ercolini, D., Russo, F., Torrieri, E., Masi, P., & Villani, F. (2006). Changes in the spoilage related microbiota of beef during refrigerated storage under different packaging conditions. Applied and Environmental Microbiology, 72, 4663–4671.
Folm- Hansen, J. (1999). On chromatic and geometrical calibration. Ph.D. thesis, Technical University of Denmark, Kgs. Lyngby.
Gomez, D. D., Clemmensen, L. H., Ersbøll, B. K., & Carstensen, J. M. (2007). Precise acquisition and unsupervised segmentation of multi-spectral images. Computer Vision and Image Understanding, 106, 183–193.
Gowen, A., O’Donnell, C., Cullen, P., Downey, G., & Frias, J. (2007). Hyperspectral imaging—an emerging process analytical tool for food quality and safety control. Trends in Food Science and Technology, 18, 590–598.
Green, A. A., Berman, M., Switzer, P., & Craig, M. D. (1988). A transformation for ordering multispectral data in terms of image quality with implications for noise removal. IEEE Transactions on Geoscience and Remote Sensing, 26, 65–74.
Hansen, M. E., Ersbøll, B. K., Carstensen, J. M., & Nielsen, A. A. (2005). Estimation of critical parameters in concrete production using multispectral vision technology. In: Lecture notes in Computer Science, LNCS3540. Kgs. Lyngby: Informatics and Mathematical Modelling, Technical University of Denmark, DTU. pp. 1228–1237.
Hastie, T., Tibshirani, R., & Friedman, J. (2009). Elements of statistical learning: data mining, inference and prediction (2nd ed.). New York: Springer.
Koutsoumanis, K., & Taoukis, P. (2006). Meat safety, refrigerated storage and transport: modelling and management. In J. Sofos (Ed.), Improving the safety of fresh meat (pp. 503–561). Cambridge: Woodhead.
Koutsoumanis, K. A., Stamatiou, A. P., Skandamis, P., & Nychas, G. J. E. (2006). Development of a microbial model for the combined effect of temperature and pH on spoilage of ground meat and validation of the model under dynamic temperature conditions. Applied and Environmental Microbiology, 71, 124–134.
Koutsoumanis, K. A., Stamatiou, A. P., Drosinos, E. H., & Nychas, G. J. E. (2008). Control of spoilage microorganisms in minced pork by a self-developed modified atmosphere induced by the respiratory activity of meat microflora. Food Microbiology, 25, 915–921.
Nielsen, A. A. (1999). An extension to a filter implementation of a local quadratic surface for image noise estimation. In: 10th international conference on image analysis and processing, ICIAP’99, 119–124.
Nychas, G. J. E., Drosinos, E. H., & Board, R. G. (1998). Chemical changes in stored meat. In R. G. Board & A. R. Davies (Eds.), The microbiology of meat and poultry (pp. 288–326). London: Blackie.
Nychas, G. J. E., Skandamis, P. N., Tassou, C. C., & Koutsoumanis, K. P. (2008). Meat spoilage during distribution. Meat Science, 78, 77–89.
Otsu, N. (1979). A threshold selection method from gray-level histograms. IEEE Transactions on Systems, Man, and Cybernetics, 9, 62–66.
Palanichamy, A., Jayas, D., & Holley, R. (2008). Predicting survival of Escherichia coli O157:H7 in dry fermented sausage using artificial neural networks. Journal of Food Protection, 71, 6–12.
Papadopoulou, O., Panagou, E. Z., Tassou, C. C., & Nychas, G.-J. E. (2011). Contribution of Fourier transform infrared (FTIR) spectroscopy data on the quantitative determination of minced pork meat spoilage. Food Research International, 44, 3264–3271.
Ross, T. (1996). Indices for performance evaluation of predictive models in food microbiology. Letters in Applied Bacteriology, 81, 501–508.
Sánchez, A. J., Albarracin, W., Grau, R., Ricolfe, C., & Barat, J. M. (2008). Control of ham salting by using image segmentation. Food Control, 19, 135–142.
Skandamis, P. N., & Nychas, G.-J. E. (2002). Preservation of fresh meat with active and modified atmosphere packaging conditions. International Journal of Food Microbiology, 79, 35–45.
Stien, L. H., Kiessling, A., & Manne, F. (2007). Rapid estimation of fat content in salmon fillets by colour image analysis. Journal of Food Composition and Analysis, 20, 73–79.
Taghizadeh, M., Gowen, A., Ward, P., & O’Donnell, C. P. (2010). Use of hyperspectral imaging for evaluation of the shelf-life of fresh white button mushrooms (Agaricusbisporus) stored in different packaging films. Innovative Food Science & Emerging Technologies, 11, 423–431.
Tran, T. N., Wehrens, R., & Buydens, L. M. (2005). Clustering multispectral images: a tutorial. Chemometrics and Intelligent Laboratory Systems, 77, 3–17.
Videometer (2009). Testimonial: Kopenhagen Fur, April 19, 2007. <http:// www.videometer.com/news/artikler2007/Testimonial_Kopenhagen_Fur.html> Accessed 01.09.09.
Acknowledgments
The authors acknowledge the Symbiosis-EU (www.symbiosis-eu.net) project (no. 211638) financed by the European Commission under the 7th Framework Programme for RTD. The information in this document reflects only the authors’ views, and the Community is not liable for any use that may be made of the information contained therein.
Author information
Authors and Affiliations
Corresponding author
Rights and permissions
About this article
Cite this article
Dissing, B.S., Papadopoulou, O.S., Tassou, C. et al. Using Multispectral Imaging for Spoilage Detection of Pork Meat. Food Bioprocess Technol 6, 2268–2279 (2013). https://doi.org/10.1007/s11947-012-0886-6
Received:
Accepted:
Published:
Issue Date:
DOI: https://doi.org/10.1007/s11947-012-0886-6