Abstract
This paper examines the role of outward foreign direct investment on energy consumption and environmental quality in BRICS from 1990 to 2019. Most of the previous research considered foreign direct investment mainly focused on the environmental impact on the host country effect and ignored the home country effect. Therefore, the current study examines the home country effect of environmental pollution. We use various methodologies like cross-sectional dependence and the Pesaran-Yamagata slope homogeneity for the diagnostic test. After confirming the diagnosis test, we employ second-generation panel unit root tests, which confirms that all elements are stationary at first difference. The pooled mean group (PMG), Westerlund cointegration, two-step generalised method of moments (GMM), panel fully modified ordinary least square (FMOLS), and dynamic ordinary least square (DOLS) model have been used to determine the short-term and long-term association among the variables. The cointegration and PMG results confirm that the short-run and long-run association exists among the considered variables. The results reveal that develo** countries produced environmental pollution at the early stage of development and checked in the long run. The study used the STIRPAT model to build an extended carbon dioxide emissions model by incorporating outward FDI, GDP per capita, and technology to achieve our objectives. The empirical results hold up the environmental Kuznets curve hypothesis, implying that outward FDI and energy consumption help expand greener technology to host countries’ environmental improvement in the long run and confirm that an inverted U-shaped linkage exists. Hence, the study suggests that develo** countries should pay more attention to sustainable development and technological development that encourages more eco-friendly and environment-friendly technology.
Similar content being viewed by others
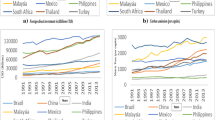
Avoid common mistakes on your manuscript.
Introduction
Particularly under liberalisation, globalisation, and privatisation policies, energy utilisation and environmental pollution have continuously increased and are predicted to increase more in the coming future (Pandey et al. 1999; Jun et al. 2021). According to the International Monetary Fund’s (IMF) report, the world’s total output increased by 3.7% in 2017 and 3.9% in 2018 (IMF 2018). Similarly, the US Energy Information Administration report reveals that the energy consumption is expected to increase by 736 quadrillions in BTUs (British thermal units) in 2040 compared to 575 quadrillions in BTUs in 2015 (US EAI 2017). This scenario creates a more significant challenge for develo** and developed countries. Another report revealed that the greenhouse gas (GHG) emissions might rise approximately up to 50% by 2050, and 70% of carbon emissions would be generated due to heavy energy consumption (OECD 2012).
To address certain pollution problems, nations need to give much more attention to their annual economic development. In develo** countries, foreign direct investment (FDI) creates a significant positive impact on economic growth and hampers both the home and host countries’ environment (Anyanwu 2012). Emerging economies strengthen their domestic and foreign investment for more economic development, which eventually create environmental problems. Outward FDI enhances industrialisation and advances technology (Buckley et al. 2020). According to WIR 2016, OFDI from develo** economics increased 91 billion to 400 billion dollars from 2000 to 2015. Develo** countries like China and India have created a better position in FDI, and they have produced high environmental pollution, especially water pollution and air pollution (Wang and Yang 2016; Li et al. 2016; Abdouli and Hammami 2017). Conclusively a sizeable emerging country generates high environmental pollution.
Most of the earlier studies considered the EKC hypothesis on developed countries (Salahuddin et al. 2018; Chandran and Tang 2013; Kivyiro and Arminen 2014). From the previous study, we do not find proper evidence to reduce pollution in emerging nations. Few studies suggest the policy measures to shifting the countries’ position haven hypothesis to halo hypothesis, particularly considering developed counties as their study area (Li et al. 2015; Sinha et al. 2017). However, very few studies focused on the essential factor affecting the environment in develo** countries, but their findings did not help reduce pollution in develo** countries like India and China. According to the International Energy Agency 2018 report, China is the most polluting country and produces 10.06 gigatons of carbon emissions in 2017–2018. Surprisingly, India and Russia were in the 3rd and 4th rank and produced approximately 3 gigatons of carbon emissions per year. Likewise, Brazil and South Africa were in the 13th and 14th globally and produced almost 2 gigatons of CO2 emissions in 2017–2018. An increase in carbon emissions is a severe concern for the BRICS union to achieve sustainable goals. Therefore, this paper attempts to solve the problem by adopting basic and advanced techniques to identify the association between OFDI, energy consumption, and carbon emissions in develo** countries like BRICS and provide proper policy and regulation to reduce environmental degradation.
The BRICS countries differ on the basis that their language, culture, background, and structure of their economies are not similar. However, they have a common denominator; i.e. they all are located in the mid-way of development. After the economic crisis, these unions continued the best performance as compared to others. In 2009 large economies like Japan and Germany shrunk as much as 6%, where Brazil stayed steady, China was 8.1%, and India was 5.9% (Zakarya et al. 2015). The present study covers the five fastest emerging economies, viz., Brazil, Russia, India, China, and South Africa. A considerable increase in economic growth has created transition and industrialised economies like the BRICS union for the last decades. BRICS nations are fast-growing develo** countries and generate more impact in the environment (Akpan et al. 2014). Among the BRICS countries, India and China have a significant role in global production and Brazil and Russia are experts in energy resources and raw materials (Kobayashi-Hillary 2007). The probationary period of the study will be 1990–2019. We proposed this period by considering the post-liberalisation, post-privatisation, and post-globalisation policies of the BRICS countries.
BRICS union is considered as a significant source of outward FDI. The BRICS accounted for 9% of the world’s FDI outflows in 2012 (Calderón-Zaks 2014). The past studies reported that FDI outflow from emerging countries like BRICS is quite impressive and has shown a significant growth in the last few years, which gives a positive picture towards economic development (Sachs 2003; WIR 2017). The BRICS union is considered with almost 40% of the global population, contributes 21% of the GDP, consumes almost 40% of the world’s energy, and generates a considerable part of global carbon emissions (WIR 2018 & Wang 2019). Due to better performance in growth and development, a negative externality generates on the environment, which will lead to climate change. The continuous climate change is now a significant concern for emerging nation as the BRICS. As the international mitigation efforts (IME) members, the BRICS nation registered with the United Nations Framework Convention on Climate Change and made initiatives to reduce the carbon emission intensity by 2020. The BRICS nations need the right policy to use alternative energy solutions for the future. BRICS countries prefer more biomass energy to control environmental pollution (Shahbaz et al. 2018). The BRICS union is at risk of environmental degradation, so it is necessary to pay more attention to this field. Therefore, the study presents a graphical overview of CO2 emissions in BRICS countries over the last 25 years.
Figure 1 shows the per capita carbon emission at an increasing rate which eventually deteriorates the environment quality in BRICS. Heavy pollutions are ultimately affected by the global greenhouse and world economic development. In 2013, the fifth BRICS summit sealed a “multilateral agreement on climate cooperation and the green economy” to control climate change and pollution in emerging countries (Liu et al. 2020). BRICS countries are recently located downstream, which means that the environmental costs are relatively high in BRICS. The International Energy Agency reported that in 2018 China was in the 1st rank, India was in the 3rd rank, and Russia was in the 4th rank. China produced 10.06 gigatons of carbon emission in 2017–2018, a massive problem for the environment. The heavy industrial revolution in India and China eventually generated high air pollution and environmental degradation in the twenty-first century (Zhu et al. 2007). Hence, to maintain substantial economic growth, it is not easy to achieve a sustainable environment.
Several studies have analysed the effects of different macroeconomic variables on environmental pollution. Most of the literature has considered carbon dioxide emissions for their analysis as a proxy variable for environmental pollution (Arouri et al. 2012; He et al. 2017; & Wang et al. 2018; Ahmad and Du 2017). However, very few studies empirically examine the nexus among quality of environment, OFDI, population, and energy utilisation. Therefore, the primary focus of the study is to associate the link among outward FDI, energy consumption, innovation, economic growth, and pollution in emerging economics like BRICS. The novelty of this study includes innovation, human capital, and outward FDI as new explanatory variables in the EKC framework. This study is quite different from other studies because it examines the dynamic relationship and identifies the EKC hypothesis by employing advanced panel techniques to estimate the results. The study applies a cross-sectional dependency test and slopes homogeneity test to check cross-section’s heterogeneity. The study uses Westerlund cointegration and pooled mean group (PMG) to identify the short-run and long-run relationship for the cointegration analysis. We also use two-step GMM, FMOLS, and DOLS to check the long-run robustness and avoid endogeneity and serial correlations problems. The rest of the article is organised as follows. The “Theoretical foundation” section presents the theoretical framework. The “Literature review” section presents the related literature review. The “Data and methodology” section discusses the data and methodology. The “Empirical results and analysis” section discusses the empirical results and discussions. The “Conclusion and policy implications” section presents the conclusion and policy implications.
Theoretical foundation
Numerous studies have been proved reverse technological transformation from OFDI (Hao et al. 2020; ** countries enhance their overseas investment, they generate environmental degradation (Christoforidis and Katrakilidis 2021); however, developed nations are more concerned about pollution and emphasise green investment (Sulemana et al. 2016).
Ehrlich and Holdren (1971) developed the IPAT model to examine the effect of human activities on the environment. The model is as follows:
where I = impact, P = population, A = wealth, T = technology. Due to restrictions on some factors and determinants, Dietz and Rosa (1997) developed a random version of the IPAT model to estimate the impact of GDP, FDI, and innovation on carbon dioxide emissions.
where I stands for carbon emissions, P stands for per capita GDP, A stands for FDI, and T stands for innovation and technology (Commoner et al. 1971; Raskin 1995). The limitation of the IPAT model is some values are missing and not determined in the model to avoid the non-proportional and non-monotonic effects. Therefore, Rose and Dietz and Rosa (1997) suggest the STIRPAT model for stochastic impacts by applying the regression model on population, affluence, and technology. Thus, the STIRPAT model has been significantly used to see the impact of GDP, outward FDI, and research and development on carbon dioxide emissions (Carmer 1998; DeHart & Soule 2000; York et al., 2003; Cai et al. 2021; Chandio et al. 2021a).
Grossman and Krueger (1995) developed the theoretical framework of environmental economics, popularly known as the environmental Kuznets curve or EKC hypothesis. EKC hypothesis explains that the level of environmental pollution increases as nations develop; after a particular point of development, the level of pollution will decrease with a further increase in the per capita income. This hypothesis is imitated as an inverted “U”-shaped curve that explains the link between pollution and GDP per capita income. According to the EKC theory, the scale effect can be measured by taking the country’s economic growth (Hao et al. 2020). The scale effect is generally accepted when a country mainly depends on primary and secondary sectors. The scale effect will increase the income level, and a transformation occurs in the secondary industry, considered a composition effect. When manufacturing industries are in the take-off stage, it encourages eco-friendly technologies to control environmental destruction. This stage increases the demand for a pollution-free environment, further encouraging more research and developmental activity and enhancing economic growth. This process is known as the technique effect. Therefore, a country needs to encourage green development by promoting eco-friendly technologies that eventually improve the environment and increase economic growth for proper development.
Literature review
Numerous researches have investigated the impact of OFDI and energy consumption on environmental degradation. In addition to the existing literature, the study added different macroeconomic variables, which also play an essential role in environmental degradations. This study investigates OFDI, energy consumption, economic growth, R&D activities, and secondary industries on environmental pollution. The recent studies on OFDI and its impact on environmental quality is presented in Table 1.
Further, the review of literature section has been classified into different sections to clarify the association among the selected variables used in the study.
Environmental pollution and outward FDI nexus
The globe is currently in a conundrum because of drastic changes in climate and environment, which eventually destroys our natural environment and ecological system (Bonan 2015; Goldstone 2018; Wang et al. 2017). Salahuddin et al. (2018) explore the impact of growth, financial development, foreign direct investment (FDI), and electricity consumption on Kuwait’s environment. They employ time series data from 1980 through 2013 and use CO2 emissions as a proxy for environmental quality. For the practical analysis, they apply the ARDL bound testing method and conclude that economic growth, FDI, and electricity consumption stimulate carbon emissions (CO2) in Kuwait; thus, these three factors deteriorate the environmental quality. Outward FDI promotes national economic growth and development. The nexus between OFDI and pollution is “inverted U-shaped”, which implies that OFDI will increase environmental pollution in the short run. Still, in the long run, OFDI reduces pollution (Hao et al. 2018).
The research on the effect of environmental pollution on OFDI is still in its infancy. Many researchers found that OFDI harms the environment (** economies. International Journal of Finance & Economics" href="/article/10.1007/s11356-021-17180-4#ref-CR119" id="ref-link-section-d267934796e1413">2020; Sahoo and Sethi 2021a). By employing the ARDL model, Jalil and Mahmud (2009) examined the EKC hypothesis between carbon emissions and per capita GDP in China. Similarly, (Wang et al. 2011; Jayanthakumaran et al. 2012; Yin et al. 2015; Kang et al. 2016; Dong et al. 2017) empirical studies support an inverse relationship between pollution and growth. Likewise, Dinda’s (2004) study was examined by considering OECD and non-OECD nations. His result deviates from other works of literature; i.e. CO2 emissions do not hamper economic growth in non-OECD regions. In contrast, carbon emissions badly hindered economic growth in OECD countries.
Environmental pollution and other macro-economic variables
Many previous works of literature have attempted to use eco-innovation to reduce carbon emissions, encouraging governments to promote R&D activity (Gu and Wang 2018; Long et al. 2017). Chen et al.’s (2018) research revealed that energy intensity and GDP would increase carbon emissions in OECD countries. Likewise, Ito (2017) considered the impact of energy structure on CO2 emissions in 42 advanced countries. In the last decades, research and development (R&D) has increased significantly over the world. Many research pieces focus on the role of R&D performance on carbon emissions in developed countries (Wang and Wang 2019; Greaker and Pade 2009). Su and Moaniba (2017) used the GMM method to examine the nexus between innovation and environmental pollution and conclude that technical innovations in liquid fuels like petroleum and natural gas significantly reduce carbon emissions. Álvarez-Herránz et al. (2017) investigated that energy-centric innovation significantly reduced greenhouse gas emissions in OECD countries. Santra (2017) revealed that innovation enhanced energy use and increased carbon emissions in the BRICS. Likewise, Yii and Geetha (2017) employed the VECM model to support that R&D and innovation reduce environmental pollution in the short run but not in the long run. To examine the macro-level analysis, the relationship between human capital and carbon emissions is not well identified in the previous studies. According to the endogenous growth model, human capital is a crucial driver of technical progress and creates a better investment environment by promoting more research and development (R&D) activities (Romer 1990; Vandenbussche et al. 2006). Hence, technological improvement provides efficiency at the production level and help to reduce carbon dioxide emissions (Cagno and Trianni 2013; Churchill et al. 2019).
Carbon emissions are among the most widely used indicators of environmental pollution. A large amount of CO2 emissions is associated with energy consumption, energy source, manufacturing industries, and the population (Li et al. 2015; Zhang et al. 2017; Sinha et al. 2017; Chandio et al. 2020a). Xu and Lin (2017) examined that the manufacturing industry heavily affects carbon emissions in China. At the same time, Li et al. (2015) find that 75% of the total emissions are generated from chemical production and ferrous metal. Environmental pollution problems are now a matter of concern, attracting governments’ and policymakers’ attention, specifically in develo** countries (Lu et al. 2018). Therefore, develo** nations need to promote sustainable growth, encourage sustainable energy consumption, and encourage more environmental-friendly technology and eco-friendly innovation (Liu and Bae 2018). Duerksen and Leonard (1980) investigate trade and domestic investment data to examine a pollution haven effect in the USA and found that FDI in pollution-intensive industries has not significantly increased in develo** countries than developed countries. Moreover, Wagner and Timmins (2009) examine the impact of environmental regulations across many host nations on the OFDI of German industries from 1996 to 2003. This study concludes that FDI creates negative externalities in both home and hosts countries and suggest effective policy needs to frame in such a way that help to control the environmental damages.
From the above literature reviews, we found that, firstly, past studies considered the impact of FDI on environmental pollution, and very few studies focus on outward FDI. Secondly, we identify that most studies explain the association between economic growth and environmental degradation; few studies examined the link at the macro-level. Thirdly, most of the researches have used time series analysis and microeconomic approaches, and a few kinds of research also consider country-level analysis from China (Hao et al. 2020). Fourthly, most of the previous academic studies considered foreign direct investment mainly focuses on the environmental impact on the host country effect and ignored the home country effect. Therefore, the present study is different from the previous studies in several aspects. Firstly, the study considers BRICS countries as the sample for panel analysis. Secondly, the study focuses on how OFDI affects the environment of the home country. Thirdly, the study examines the dynamic impact of considered variables on environmental pollution using rigorous econometric models.
Data and methodology
To investigate the effect of outward FDI and energy consumption on environmental pollution, we consider the strongly balanced panel data for BRICS from 1990 to 2019. The panel data estimation is examined to capture the behaviour of dependent and independent variables and provide a more efficient estimation for further research. The study uses panel analysis to identify more accurate inference than time series and provide better socioeconomic policies (Heckman et al. 1998; Hsiao 2007; Hsiao 2010). The data are obtained from secondary data sources such as UNCTAD (2020) statistics and World Development Indicators (WDI) of the World Bank (2020). The STIRPAT model mainly was used to analyse the impact of the explanatory variables on the environment (Bargaoui et al. 2014). From the previous study (Wang and Liu 2008; Cantwell 2009; Grossman and Krueger 1991), the present study develops the functional equation to investigate the effect of OFDI and the other explanatory variables on carbon dioxide emissions in BRICS. Equation (3) is formulated as follows.
where ENV is denoted as environmental pollution, which is a function of outward FDI (OFDI), per capita gross domestic product (GDP), energy consumption (EC), labour force (LAB), physical capital (GCF), secondary industry (SI), research and development (R&D), and human capital (HC). This study also considered the geographical distance as a dummy variable, i.e., denoted as X. Taking geographical distance as a variable is essential to measure the pollutions like air pollution and water pollution (Zhao et al. 2015; Hao et al. 2020). The considered variables’ summary is signed positive and described in Table 2. By considering Eq. (3), our model specified to analyse the effect of energy consumption and outward FDI on the environment in BRICS.
All the variables are considered in natural logarithm form, where t = 1, 2… T refers to the time period and I = 1, 2 … N refers to the cross-section data. The parameters β1, β2,..,and β9 represent the long-run elasticity estimators of dependent and independent variables and uit is the white noise error term.
By adopting dynamic and static panel models, Zhu and Ye (2018) examined that OFDI has not significantly improved the Chinese environment. However, Eskeland and Harrison’s (1997) study analysed that outward FDI helps improve the environmental quality of the host and home countries by encouraging more pollution avoidance effects and green investment. Yang and Liu’s (2013) research focused on OFDI and its effect on home country carbon emissions in Japan and revealed that OFDI could effectively reduce carbon emissions. Ouyang et al. (2020) used the panel data analyses by considering listed Chinese companies found that the OFDI effectively improves regional environmental pollution. Many studies reported unidirectional causality from energy consumption to carbon emissions (Apergis and Payne 2009; Ozturk 2010; Pao and Tsai 2011). Likewise, some studies confirmed bidirectional causality between energy consumption and carbon emissions in the long-run and no short-run relations (Dogan and Aslan 2017; Cai et al. 2018). From the above evidence, the present study identified that the associations between outward FDI, energy consumption, and pollution are providing a mixed result; subsequently, the study develops the null hypothesis that:
-
H1a: There are no long-run and short-run causal relations that exist between outward FDI and carbon emissions in BRICS.
-
H1b: There are no long-run and short-run associations that exist between energy consumptions and carbon emissions in BRICS.
Extensive research on the environmental Kuznets curve (EKC) explains the relationship between GDP and carbon emissions (Grossman and Krueger 1995; Dinda 2004; Nahman and Antrobus 2005). Since then, many studies have confirmed the EKC hypothesis exists in developed countries (Mielnik and Goldemberg 2002; Ahmad et al. 2021). For our dataset of five develo** countries with data from 1990 to 2019, we test the second null hypothesis of this study, which is the EKC hypothesis.
H 2: The EKC hypothesis does not exist in BRICS.
Cross-sectional dependence (CSD) test
The first-generation unit root tests have some disadvantages: the cross-sections in the panel data are independent. However, the presence of the CSD assumption is not appropriate for empirical investigation. The panel analysis’s cross-sectional dependence (CSD) test is considered the most critical test because CSD might create biased and inconsistent results (Phillips and Sul 2003). This study uses the CSD test developed by Chudik and Pesaran (2015) to identify cross-sectional dependence (CSD) exists within our model or not. Pesaran’s CSD test using the following equation is based on the correlations between the disturbances in different cross-section units.
where T represents the period, N indicates the cross-sections in the panel, ∂ijj is correlation coefficients of I and j units. The null hypothesis of the cross-section is weak the CSD, and the statistic is asymptotically distributed.
Second-generation panel unit-root test
Since CSD among countries, we apply the second-generation unit root tests for stationarity of the considered variables. Therefore, this study employs the CADF panel second-generation unit root test for the preliminary test. CADF test is an extended version of the augmented Dickey-Fuller (ADF) test; these tests take the following equation form:
where xit is considered as an analysed variable, ϵit is the white noise error term, Δ is the difference, and T and α are time trends and individual intercepts. The appropriate and optimal lag lengths are based on the Akaike information criterion (AIC).
Westerlund (2007) panel cointegration test
The study employs Westerlund’s (2007) dynamic panel cointegration method to examine the selected variables’ heterogeneity and cross-sectional dependence test. The null hypothesis of this approach is no cointegration exists in the error-correction term (ECT). To check the cointegrating association between dependent and independent variables, hence, we have employed the following error-correction model:
where dt contains the deterministic element and cointegration is expressed by Yit − 1 − βiXit − 1 = 0. αi measures the velocity of adjustment, and cointegration is assured by αi < 0, whereas αi= 0 falsifies the presence of cointegration.
Generalised method of moments test
The generalised method of moments test can apply from both level and difference equations. GMM work better for panel with large sample size. The GMM estimator gives more robustness results as compared to the other econometrics model. By considering various weight matrixes, GMM approach is generally divided into one-step GMM estimators and two-step GMM estimators. The two-step GMM is comparably a better instrument than the conventional one-step GMM because it helps to remove simultaneity from the regressor set by including instrumental variables. Ullah et al. (2018) developed some generic STATA codes for using GMM estimator to control three sources of endogeneity problem better, i.e. (i) unobserved heterogeneity, (ii) simultaneity, and (iii) dynamic heterogeneity. Arellano and Bond (1991) and Blundell and Bond (1998) included lagged dependent variable as a regressor in the model to reduce possible endogeneity; thus, they developed a GMM estimator for dynamic panel modelling. Windmeijer (2005) explained that biases could be rectified by giving more attention to small samples’ variation. A null hypothesis of the Sargan test is the instruments are exogenous. Therefore, the higher the p value of the Sargan test, the better. The Arellano-Bond two-step difference GMM test for autocorrelation has a null hypothesis of no autocorrelation. This test generally applied to differenced residuals. The AR (2) process in the first differences generally rejects the null hypothesis. Therefore, the AR (2) test is more critical to define autocorrelation in the regression models.
Panel FMOLS and panel DOLS
This is essential to check the robustness of the long-run connection among the elements in our model. Hence, this is necessary to examine the elasticity of dependent variables with respect to considered independent elements. Pedroni (2001) employed the fully modified ordinary least square (FMOLS) technique to solve the problem related to endogeneity between regressors. This test corrected the stochastic regressor and the correlation between the cointegrating equations in the long run. Likewise, the dynamic ordinary least square (DOLS) test was propounded by Kao and Chiang (2000) to identify and remove the endogeneity and autocorrelation problems using a parametric approach in the panel data set. DOLS estimators are applied for both homogeneous and heterogeneous panels. This test is better than FMOLS because it removes endogeneity and autocorrelation problems by adopting a parametric approach.
Empirical results and analysis
We employ panel data techniques to estimate the dynamic behaviour of the dependent variable on independent variables in BRICS countries. The primary motivation of summary statistics is to analyse all sample summaries and selected elements of the study. The correlation matrixes provide a podium for regression to verify the association of dependent and independent variables. Hence, descriptive statistics and correlation analysis have been carried out before estimating advanced tests for panel analysis.
Table 3 presents the summary statistics results of dependent and independent variables. It observed that outward FDI and carbon emissions have the highest standard deviation than other independent variables with 2.15 and 5.4 mean. This result implies the flow of outward FDI and carbon emissions are relatively high in BRICS. The volatility of carbon emissions is less than the volatility of OFDI. Pearson pair-wise correlation matrix explains the strength and nature of the correlation among the considered variables. The variables outward FDI, GDP, E.C., R&D, and HC positively correlate with environmental pollution (ENV). The results from the correlation matrix explain that the model is free from the multicollinearity problem as the element does not exceed more than 0.85, leading to VIF values of less than 5 (Gujarati and Porter 2009).
Table 4 represents the slope homogeneity test. As per the CSD and slope homogeneity results, the study has considered employing heterogeneous panel models that control the cross-sectional dependency among the variables. Before adopting the panel methodologies, it is necessary to examine the existence of cross-sectional dependency in the model. Thus, the study uses the Pesaran (2007) CD test, as it provides the confirmations result about the use of panel unit root test for further explanations. The null hypothesis of the CSD test is there is cross-sectional independence among cross countries, against the alternative hypothesis is cross dependency among the sample size.
The study assumes there is a high probability of the existence of cross-sectional dependence among considered variables as the BRICS countries are interlinked internationally and based on a different type of cultural and economic background. We employ Breusch and Pagan (1980) L.M. test, Pesaran (2004) CD test, and Pesaran (2004) Scaled L.M. for testing the cross-dependencies. The study rejects the null hypothesis of no cross-sectional dependencies at 1% significance level. The results reveal that the selected variables exhibit the presence of heterogeneity across the cross-sections significantly. Therefore, this confirms the presence of cross-sectional dependence among the variables.
In the presence of CSD, the study employs second-generation panel unit root tests CADF and CIPS to examine the stationary properties of the considered variables in Table 5. We find that some variables like human capital, labour force, and manufacturing industries and R&D are stationary at their levels. However, this result cannot be generalised to the stationarity at level; subsequently, we employ the first difference where all the elements are stationary at 95% and 99% significance level. The panel data further employs an advance econometric test for long-run analysis of the parameters.
Many panel cointegration approaches are available to identify the long-run cointegration among the variables developed by Pedroni (2001) and Westerlund (2007). Westerlund (2007) cointegration approach generally employs for CDS and heterogeneity analyses for the dynamic cointegration relationship among variables. The Westerlund panel cointegration test reveals a more robust and consistent result for dependent and independent variables. The results are divided into panel statistics (Pt, Pa) and group statistics (Gt, Ga). The panel statistics method focuses on the error correction mechanism and includes the cross-sectional units, where the group statistics method does not consider the information from the error term (Latif et al. 2021b; Haldar and Sethi 2021). The findings also explain that some independent variables are positive nexus with carbon emissions, and some are not statistically significant. However, the variables like OFDI and HC are negative and statistically significant, indicating an indirect relationship with dependent variables in the short run. The long-run equations confirm that there is a long-run association present among the variables. That means outward FDI and energy utilisation will help to reduce BRICS’ pollution in the long run.
The robustness of long-run results is discussed in Table 8 and Table 9. We estimate generalised methods of moments (GMM) to ignore the endogeneity and serial correlation problem. For a more convenient result, this study also considers the panel fully modified ordinary least square (FMOLS) and the panel dynamic ordinary least square (DOLS), which considered by many researchers (Apergis and Payne 2010; Jebli et al. 2016; Sahoo and Sethi 2021a) for robustness test of EKC hypothesis.
The study employs both system and difference two-step GMM. The result shows that energy consumption directly affects the environmental quality in BRICS in different GMM, not in system GMM. This also shows that a decrease in CO2 emissions by − 0.64% is impacted by a 1% rise in the outward FDI coefficient, thereby validating the higher levels of outward FDI in BRICS reduce carbon emissions, improving environmental quality (Braconier et al. 2001). In this study, Sargant test statistics’ application fails to reject the null hypothesis, implying that our instrument set is robust. The AR (2) coefficient of each dynamic panel model elaborates that the study accepts the alternative hypothesis. This result reveals an upturn U-shaped link between outward foreign investment and CO2 emissions and confirms the existence of PHH in BRICS. This study finding verifies the existence EKC hypothesis exists in develo** nations like BRICS (Wang et al. 2011; Jayanthakumaran et al. 2012; Bakirtas and Cetin 2017; Hao et al. 2020; li et al. 2020; Haldar and Sethi 2021). However, some studies found EKC hypothesis does not exist in underdeveloped and develo** countries (Salahuddin and Gow 2016; Lee and Brahmasrene 2014).
The panel FMOLS and the DOLS methods are effective in eliminating the endogeneity problems and serial correlation problems. First, the study reports the estimators obtained by dynamic least squares (DOLS) for the countries individually with effects fixed time. The estimators can be computed as elasticities directly. The positive coefficient elements explain the ratio between pollution and each explanatory variable, and some variables’ strength of the cointegration vector is overwhelming. Results from both panel estimators (Table 10) indicate that some variables like R&D and EC are positive and statistically significant on carbon emissions.
Meanwhile, GDP per capita and outward FDI is negatively statistically significant at the 1% and 5% level on carbon dioxide emissions. Thus, the connection between environmental pollution, outward FDI, and economic growth confirms an inverted U-shaped curve exists. Therefore, the results of FMOLS and DOLS validate the EKC hypothesis that exists in BRICS countries. These results resemble previous studies by Heidari et al. (2015) and Apergis and Ozturk (2015), Chandio et al. (2020a) and Sahoo and Sethi (2021b) that found that the EKC hypothesis is validated in develo** countries in the long run and contradictory with previous studies by Boluk and Mert (2014) and Liu and Bae (2018) found that EKC hypothesis is invalid in develo** nations. The indicator like human capital reveals a negative and insignificant value that explains human capital is insufficient in reducing the pollution in BRICS countries in the long run.
Conclusion and policy implications
This paper investigates the effects of outward FDI and energy utilisation on environmental pollution in BRICS from 1990 to 2019. The study first employed the second-generation unit root tests to satisfy the selected determinants’ stationarity properties. The Westerlund (2007) cointegration approach is used to scrutinise the long-run cointegrating relationship among the variables. The study also employed robust two-step difference GMM, panel FMOLS and DOLS, to avoid endogeneity problems and verify the connection among the macro-economic variables. The PMG results confirm a short-run nexus, and the ECT value revealed that the short-run disturbances would be corrected at − 0.38% to achieve the equilibrium level in the long run. PMG results confirm that OFDI and HC are negative and significant, which means an inverse relationship exists with ENV and explains there is a long-run association present among the variables. The study used advanced models like GMM and DOLS for the robustness check, which reject the study’s null hypothesis and confirms that both short-run and long-run associations exist between OFDI, energy consumption, and carbon dioxide emissions (Cole 2006; Anyanwu 2012).
Therefore, at the early stage of development, develo** countries produce environmental pollution and will be checked in the long run. It can be concluded from empirical results domestic investment (OFDI) and more use of energy increase carbon emissions in BRICS in the short run and will be checked in the long run. Hence, the most popular EKC hypothesis exist in develo** nations like BRICS (Braconier et al. 2001; Pao and Tsai 2011) and also confirms that an inverted U-shaped connection exists between outward FDI and carbon emissions in BRICS (Hao et al. 2018; Jena and Sethi 2019). In GMM, the GDP per capita is positive and significant, indicating that an increase in the growth rate of countries will lead to an increase in the number of carbon emissions in the long run. This evidence is consistent with the studies of (Jayanthakumaran et al. 2012; Wu et al. 2015; Mahalik et al. 2018). This study is the first empirical analysis of most emerging countries taking as pollution, energy consumption, OFDI in a single lens. However, most previous studies provide a mixed result about the association between OFDI and carbon emissions (Boluk and Mert 2014; Liu and Bae 2018). From a policy point of view, the study suggests that generating a sustainable healthy environment from the future fear of climate change and global warming should reduce excessive energy consumption without hampering rising growth. By focusing on theoretical contribution, this study will help for future study.
The study used the STIRPAT model to build an extended carbon dioxide emissions model by incorporating outward FDI, GDP per capita, and technology to achieve our objectives. The idea of the IPAT and STIRPAT model explains that a statistical and conceptual framework for assessing human impacts on the environment. The goal of STIRPAT theory is to provide an analytic strategy for testing. The underlying goal is to identify the primary drivers of environmental harm and to uncover lever points for ameliorating that harm. Hence, we hope that the STIRPAT model will contribute to the fundamental understanding of both human and natural systems and provide results that help policymakers and decision-makers.
This study also highlighted economic and environmental pollution in the context of extreme events. According to the Energy Gap Report, 2020, greenhouse gas (GHG) emissions continued to increase for the last couple of years, particularly in China and India. However, due to the COVID-19 pandemic from the end of 2019, the global CO2 emissions have been reduced by about 7%. Global pandemic and environmental degradation is now a matter of concern that what will be the future situations? After the normalisation, the GHGs will be predicted to increase more than before to achieve the market demand-supply equilibrium positions. Therefore, the study suggests that develo** countries should give more attention to sustainable development. Energy consumption in emerging economies is relatively high than in advanced countries because of the initial development stage; therefore, it is a concern. So, policymakers and governments should give more attention to alternate energy solutions. Therefore, the COVID-19 outbreak in 2019 is a global emergency event for public health. The policy needs to frame systematically by focusing on the relationship between economic growth and environmental pollution by encouraging eco-friendly vehicles, eco-friendly technology, pollution-free machines, etc.
Develo** countries should encourage more sustainable energy technologies. Develo** economies like BRICS enhance their domestic as well as foreign investment by adopting advanced technology. This transfer should be continued until the emerging economies can achieve proper economic progression. The technological development in emerging economies should encourage more eco-friendly and environment-friendly technology to achieve a sustainable natural environment. Another crucial implication is also needed to design environmental policies focused on green innovation. Being a desirable market, the BRICS union should boost green innovation drive by using market-oriented policies. Develo** countries need to open and strengthen their market by providing incentives for technology developers, spending more on R&D activities, and encouraging governments to venture capital. The government also needs to frame the profitable strategies, inspire PPP, i.e. public-private partnerships, to circulate the environmental consciousness, guideline for energy efficiency, and generate a pollution-free environment.
The current research has certain limitations: Firstly, the study considered BRICS countries over 1990–2019 for panel analysis. Secondly, due to the lack of data, the analysis is focused on specific determinants and ignores many crucial factors that influence the quality of the environment. However, the current study provides a fruitful direction for further future research. The researcher can use both time series and extensive panel framework to identify the link between outward FDI and pollution concerning each of the variables. The research can be expanded by considering develo** countries and a comparative analysis on developed and develo** countries by employing advanced methods. By covering up these research gaps, the present study would be pretty practical from a policy aspect for other emerging and develo** economies.
Abbreviations
- CSD:
-
Cross-sectional dependence
- CIPS:
-
Cross-sectionally augmented IPS
- CADF:
-
Cross-sectionally augmented ADF
- OFDI:
-
Outward foreign direct investment
- BRICS:
-
Brazil, Russia, India, China, South Africa
- EKC:
-
Environmental Kuznets curve
- FMOLS:
-
Fully modified ordinary least square
- DOLS:
-
Dynamic ordinary least square
- GMM:
-
Generalised method of moments
- PMG:
-
Pooled mean group
- OLS:
-
Ordinary least squares
- GHG:
-
Greenhouse gases
- IMF:
-
International monetary funds
- WIR:
-
World Investment Report
- BTUs:
-
British thermal units
- GDP:
-
Gross domestic product
- IPAT:
-
Impact = population + affluence + technology
- STIRPAT:
-
Stochastic impacts by regression on population, affluence, and technology
- UNCTAD:
-
United Nations Conference on Trade and Development
- WDI:
-
World development indicators
- ECM:
-
Error correction model
- ECT:
-
Error correction term
- PHH:
-
Pollution haven hypothesis
- R&D:
-
Research and development
- FDI:
-
Foreign direct investment
- ARDL:
-
Autoregressive distributed lags
- VECM:
-
Vector error correction model
- OECD:
-
Organisation for Economic Co-operation and Development
- CO2:
-
Carbon emissions
References
Abdouli M, Hammami S (2017) The impact of FDI inflows and environmental quality on economic growth: an empirical study for the MENA countries. Journal of the Knowledge Economy 8(1):254–278
Ahmad M, Muslija A, Satrovic E (2021) Does economic prosperity lead to environmental sustainability in develo** economies? Environmental Kuznets curve theory. Environmental Science and Pollution Research 28(18):22588–22601
Ahmad N, Du L (2017) Effects of energy production and CO2 emissions on economic growth in Iran: ARDL approach. Energy 123:521–537
Akalpler E, Hove S (2019) Carbon emissions, energy use, real GDP per capita and trade matrix in the Indian economy-an ARDL approach. Energy 168:1081–1093
Akpan, U., Isihak, S., & Asongu, S. (2014). Determinants of foreign direct investment in fast-growing economies: a study of BRICS and MINT. African Governance and Development Institute W.P./14/002.
Alshehry AS, Belloumi M (2015) Energy consumption, carbon dioxide emissions and economic growth: the case of Saudi Arabia. Renewable and Sustainable Energy Reviews 41:237–247
Álvarez-Herránz A, Balsalobre D, Cantos JM, Shahbaz M (2017) Energy innovations-GHG emissions nexus: fresh empirical evidence from OECD countries. Energy Policy 101:90–100
Anyanwu JC (2012). Why does foreign direct investment go where it goes? New Evidence from African Countries. Annals of Economics & Finance, 13(2)
Apergis N, Ozturk I (2015) Testing environmental Kuznets curve hypothesis in Asian countries. Ecological Indicators 52:16–22
Apergis N, Payne JE (2009) CO2 emissions, energy usage, and output in Central America. Energy Policy 37(8):3282–3286
Apergis N, Payne JE (2010) Renewable energy consumption and economic growth: evidence from a panel of OECD countries. Energy policy 38(1):656–660
Arellano M, Bond S (1991) Some tests of specification for panel data: Monte Carlo evidence and an application to employment equations. The review of economic studies 58(2):277–297
Arouri MEH, Youssef AB, M'henni H, Rault C (2012) Energy consumption, economic growth and CO2 emissions in Middle East and North African countries. Energy policy, 45, 342-349
Bakirtas I, Cetin MA (2017) Revisiting the environmental Kuznets curve and pollution haven hypotheses: MIKTA sample. Environmental Science and Pollution Research 24(22):18273–18283
Bargaoui SA, Liouane N, Nouri FZ (2014) Environmental impact determinants: an empirical analysis based on the STIRPAT model. Procedia-Social and Behavioral Sciences 109:449–458
Bhujabal P, Sethi N, Padhan PC (2021) ICT, Foreign direct investment and environmental pollution in major Asia Pacific countries. Environmental Sciences and Pollution Research 28(31):42649–42669. https://doi.org/10.1007/s11356-021-13619-w
Blundell R, Bond S (1998) Initial conditions and moment restrictions in dynamic panel data models. Journal of econometrics 87(1):115–143
Bonan G (2015) Ecological climatology: concepts and applications. Cambridge University Press
Braconier H, Ekholm K, Knarvik KHM (2001) In search of FDI-transmitted R&D spillovers: a study based on Swedish data. Review of World Economics 137(4):644–665
Breusch TS, Pagan AR (1980) The Lagrange multiplier test and its applications to model specification in econometrics. The review of economic studies 47(1):239–253
Buckley PJ, Chen L, Clegg LJ, Voss H (2020) The role of endogenous and exogenous risk in FDI entry choices. Journal of World Business 55(1):101040
Cagno E, Trianni A (2013) Exploring drivers for energy efficiency within small-and medium-sized enterprises: first evidences from Italian manufacturing enterprises. Applied Energy 104:276–285
Calderón-Zaks M (2014) Are the BRICS a viable alternative to the West? A succinct analysis. Perspectives on Global Development and Technology 13(1-2):61–69
Cantwell J (2009) Location and the multinational enterprise. Journal of international business studies 40(1):35–41
Chandio AA, Akram W, Ahmad F, Ahmad M (2020a) Dynamic relationship among agriculture-energy-forestry and carbon dioxide (CO 2) emissions: empirical evidence from China. Environmental Science and Pollution Research 27(27):34078–34089
Chandio AA, Akram W, Ozturk I, Ahmad M, Ahmad F (2021a). Towards long-term sustainable environment: does agriculture and renewable energy consumption matter?. Environmental Science and Pollution Research, 1-20
Chandio AA, Jiang Y, Ahmad F, Akram W, Ali S, Rauf A (2020b) Investigating the long-run interaction between electricity consumption, foreign investment, and economic progress in Pakistan: evidence from VECM approach. Environmental Science and Pollution Research 27(20):25664–25674
Chandio AA, Shah MI, Sethi N, Mushtaq Z (2021b) Assessing the effect of climate change and financial development on agricultural production in ASEAN-4: the role of renewable energy, institutional quality, and human capital as moderators. Environmental Science and Pollution Research:1–15
Chandio AA, Shah MI, Sethi N, Mushtaq Z (2021c) Assessing the effect of climate change and financial development on agricultural production in ASEAN-4: the role of renewable energy, institutional quality, and human capital as moderators. Environmental Sciences and Pollution Research. https://doi.org/10.1007/s11356-021-16670-9
Chandran VGR, Tang CF (2013) The impacts of transport energy consumption, foreign direct investment and income on CO2 emissions in ASEAN-5 economies. Renewable and Sustainable Energy Reviews 24:445–453
Chen C (2018) Impact of China's outward foreign direct investment on its regional economic growth. China & World Economy 26(3):1–21
Chen J, Wang P, Cui L, Huang S, Song M (2018) Decomposition and decoupling analysis of CO2 emissions in OECD. Applied energy 231:937–950
Chen-chen ZLP (2013). Home country environmental effects of China’s foreign direct investment: based on the perspective of regional differences [J]. China Population, Resources and Environment, 8
Christoforidis T, Katrakilidis C (2021). Does foreign direct investment matter for environmental degradation? Empirical Evidence from Central–Eastern European Countries. Journal of the Knowledge Economy, 1-30
Chudik A, Pesaran MH (2015) Common correlated effects estimation of heterogeneous dynamic panel data models with weakly exogenous regressors. Journal of Econometrics 188(2):393–420
Churchill SA, Inekwe J, Smyth R, Zhang X (2019) R&D intensity and carbon emissions in the G7: 1870–2014. Energy Economics 80:30–37
Cole MA (2006) Does trade liberalisation increase national energy use? Economics Letters 92(1):108–112
Commoner B, Corr M, Stamler PJ (1971) The causes of pollution. Environment: Science and Policy for Sustainable Development 13(3):2–19
Dietz T, Rosa EA (1997) Effects of population and affluence on CO2 emissions. Proceedings of the National Academy of Sciences 94(1):175–179
Dinda S (2004) Environmental Kuznets curve hypothesis: a survey. Ecological economics 49(4):431–455
Dogan E, Aslan A (2017) Exploring the relationship among CO2 emissions, real GDP, energy consumption and tourism in the E.U. and candidate countries: evidence from panel models robust to heterogeneity and cross-sectional dependence. Renewable and Sustainable Energy Reviews 77:239–245
Dong K, Sun R, Hochman G (2017) Do natural gas and renewable energy consumption lead to less CO2 emission? Empirical evidence from a panel of BRICS countries. Energy 141:1466–1478
Duerksen C, Leonard HJ (1980) Environmental regulations and the location of industries: An international perspective. Columbia Journal of World Business 15(2):52–58
Dunning JH (1991). The eclectic paradigm of international production. The nature of the transnational firm, 121
Ehrlich PR, Holdren JP (1971) Impact of population growth. Science 171(3977):1212–1217
Eskeland GS, Harrison AE (1997). Moving to Greener Pastures?: Multinationals and the Pollution-Haven Hypothesis. World Bank Publications
Galeotti M, Lanza A (2005) Desperately seeking environmental Kuznets. Environmental Modelling & Software 20(11):1379–1388
Goldstone JA (2018). Demography, environment, and security. In Environmental conflict (pp. 84-108). Routledge
Greaker M, Pade LL (2009) Optimal carbon dioxide abatement and technological change: should emission taxes start high in order to spur R&D? Climatic Change 96(3):335–355
Grossman GM, Krueger AB (1991). Environmental impacts of a North American free trade agreement
Grossman GM, Krueger AB (1995) Economic growth and the environment. The quarterly journal of economics 110(2):353–377
Gu G, Wang Z (2018) Research on global carbon abatement driven by R&D investment in the context of INDCs. Energy 148:662–675
Gujarati DN, Porter DC (2009). Basic econometrics (international edition). New York: McGraw-Hills Inc.
Haldar A, Sethi N (2021) Effect of institutional quality and renewable energy consumption on co2 emissions - an empirical investigation for develo** countries. Environmental Science and Pollution Research 28(12):15485–15503. https://doi.org/10.1007/s11356-020-11532
Halliru AM, Loganathan N, Sethi N, Hassan AAG (2020) FDI inflows, energy consumption and economic growth: testing the pollution haven hypothesis for ECOWAS countries. Int. J. Green Economics 14(4):327–348. https://doi.org/10.1504/IJGE.2020.10034342
Han B (2021). Does China’s OFDI successfully promote environmental technology innovation?. Complexity, 2021
Hao Y, Deng Y, Lu ZN, Chen H (2018) Is environmental regulation effective in China? Evidence from city-level panel data. Journal of Cleaner Production 188:966–976
Hao Y, Guo Y, Guo Y, Wu H, Ren S (2020) Does outward foreign direct investment (OFDI) affect the home country’s environmental quality? The case of China. Structural Change and Economic Dynamics 52:109–119
He J, Richard P (2010) Environmental Kuznets curve for CO2 in Canada. Ecological economics 69(5):1083–1093
He Z, Xu S, Shen W, Long R, Chen H (2017) Impact of urbanisation on energy related CO2 emission at different development levels: regional difference in China based on panel estimation. Journal of cleaner production 140:1719–1730
Heckman JJ, Ichimura H, Smith JA, Todd PE (1998). Characterising selection bias using experimental data
Heidari H, Katircioğlu ST, Saeidpour L (2015) Economic growth, CO2 emissions, and energy consumption in the five ASEAN countries. International Journal of Electrical Power & Energy Systems 64:785–791
Hsiao C (2007) Panel data analysis—advantages and challenges. Test 16(1):1–22
Hsiao C (2010). Longitudinal data analysis. In Microeconometrics (pp. 89-107). Palgrave Macmillan, London
IEA 2018 CO2 Emissions from Fossil Fuel Combustion 2018 (International Energy Agency) (https://webstore.iea.org/ co2-emissions-from-fuel-combustion-2018).
IMF. (2018). World Economic Outlook Update, January 2018
Isik C, Dogru T, Turk ES (2018) A nexus of linear and non-linear relationships between tourism demand, renewable energy consumption, and economic growth: theory and evidence. International Journal of Tourism Research 20(1):38–49
Ito K (2017) CO2 emissions, renewable and non-renewable energy consumption, and economic growth: evidence from panel data for develo** countries. International Economics 151:1–6
Jalil A, Mahmud SF (2009) Environment Kuznets curve for CO2 emissions: a cointegration analysis for China. Energy policy 37(12):5167–5172
Jayanthakumaran K, Verma R, Liu Y (2012) CO2 emissions, energy consumption, trade and income: a comparative analysis of China and India. Energy Policy 42:450–460
Jebli MB, Belloumi M (2017) Investigation of the causal relationships between combustible renewables and waste consumption and CO2 emissions in the case of Tunisian maritime and rail transport. Renewable and Sustainable Energy Reviews 71:820–829
Jebli MB, Youssef SB, Ozturk I (2016) Testing environmental Kuznets curve hypothesis: the role of renewable and non-renewable energy consumption and trade in OECD countries. Ecological Indicators 60:824–831
Jena NR, Sethi N (2019) Foreign aid and economic growth in Sub-Saharan Africa. African Journal of Economic and Management Studies. 11(1):147–168. https://doi.org/10.1108/AJEMS-08-2019-0305
Jun W, Mughal N, Zhao J, Shabbir MS, Niedbała G, Jain V, Anwar A (2021) Does globalisation matter for environmental degradation? Nexus among energy consumption, economic growth, and carbon dioxide emission. Energy Policy 153:112230
Kang YQ, Zhao T, Yang YY (2016) Environmental Kuznets curve for CO2 emissions in China: a spatial panel data approach. Ecological Indicators 63:231–239
Kao C, Chiang MH (2000) On the estimation and inference of a cointegrated regression in panel data. Nonstationary Panels, Panels Cointegration and Dynamic Panels. Advances in Econometrics, 15
Khan MI, Teng JZ, Khan MK (2020) The impact of macroeconomic and financial development on carbon dioxide emissions in Pakistan: evidence with a novel dynamic simulated ARDL approach. Environmental Science and Pollution Research 27(31):39560–39571
Kivyiro P, Arminen H (2014) Carbon dioxide emissions, energy consumption, economic growth, and foreign direct investment: causality analysis for Sub-Saharan Africa. Energy 74:595–606
Kobayashi-Hillary M (Ed.). (2007). Building a future with Brics: the next decade for offshoring (Vol. 4643). Springer Science & Business Media
Latif Z, Latif S, **mei L, Pathan ZH, Salam S, Jianqiu Z (2018) The dynamics of ICT, foreign direct investment, globalisation and economic growth: panel estimation robust to heterogeneity and cross-sectional dependence. Telematics and Informatics 35(2):318–328
Lee JW, Brahmasrene T (2014) ICT, CO2 emissions and economic growth: evidence from a panel of ASEAN. Global Economic Review 43(2):93–109
Li H, Wei YM, Mi Z (2015) China's carbon flow: 2008–2012. Energy Policy 80:45–53
Li J, Chandio AA, Liu Y (2020) Trade impacts on embodied carbon emissions—evidence from the bilateral trade between China and Germany. International Journal of Environmental Research and Public Health 17(14):5076
Li X, Song J, Lin T, Dixon J, Zhang G, Ye H (2016) Urbanisation and health in China, thinking at the national, local and individual levels. Environmental Health 15(S1):S32
Liu JL, Ma CQ, Ren YS, Zhao XW (2020) Do real output and renewable energy consumption affect CO2 emissions? Evidence for selected BRICS countries. Energies 13(4):960
Liu X, Bae J (2018) Urbanisation and industrialisation impact of CO2 emissions in China. Journal of cleaner production 172:178–186
Long X, Chen Y, Du J, Oh K, Han I (2017) Environmental innovation and its impact on economic and environmental performance: evidence from Korean-owned firms in China. Energy Policy 107:131–137
Lu Y, Wang Y, Zuo J, Jiang H, Huang D, Rameezdeen R (2018) Characteristics of public concern on haze in China and its relationship with air quality in urban areas. Science of the Total Environment 637:1597–1606
Mahalik MK, Mallick H, Padhan H, Sahoo B (2018) Is skewed income distribution good for environmental quality? A comparative analysis among selected BRICS countries. Environmental Science and Pollution Research 25(23):23170–23194
Mielnik O, Goldemberg J (2002) Foreign direct investment and decoupling between energy and gross domestic product in develo** countries. Energy policy 30(2):87–89
Mirza FM, Kanwal A (2017) Energy consumption, carbon emissions and economic growth in Pakistan: dynamic causality analysis. Renewable and Sustainable Energy Reviews 72:1233–1240
Mohanty S, Sethi N (2019) Outward FDI, human capital and economic growth in BRICS countries: an empirical insight. Transnational Corporations Review 11(3):235–249
Nahman A, Antrobus G (2005) The environmental Kuznets curve: a literature survey. South African Journal of Economics 73(1):105–120
NCTAD, U. (2018). UNCTAD world investment report. Geneva: UNCTAD 1-213
OECD (2012), OECD Environmental Outlook to 2050: The consequences of inaction, OECD Publishing, Paris, available at: doi: 10.1787/9789264122246-en
Outlook AE (2017) U.S. Energy Information Administration, 2017. Source: https://www. eia. gov/outlooks/steo
Ouyang Y, Huang X, Zhong L (2020) The impact of outward foreign direct investment on environment pollution in home country: local and spatial spillover effects. China Industrial Economics 2:98–121
Ozturk I (2010) A literature survey on energy–growth nexus. Energy policy 38(1):340–349
Pandey M, Gupta S, Banerjee S, Hazra AK, Kumar A, Bahari A (1999). Capacity building for Integrating Environmental Considerations in Development Planning and Decision Making. National Council of Applied Academic Research. New Delhi, India
Pao HT, Tsai CM (2011) Modeling and forecasting the CO2 emissions, energy consumption, and economic growth in Brazil. Energy 36(5):2450–2458
Pedroni P (2001) Purchasing power parity tests in cointegrated panels. Review of Economics and statistics 83(4):727–731
Pesaran M (2004) General diagnostic test for cross sectional independence in panel. Journal of Econometrics 68(1):79–113
Pesaran MH (2007) A simple panel unit root test in the presence of cross-section dependence. Journal of applied econometrics 22(2):265–312
Pesaran MH, Yamagata T (2008) Testing slope homogeneity in large panels. Journal of econometrics 142(1):50–93
Phillips PC, Sul D (2003) Dynamic panel estimation and homogeneity testing under cross section dependence. The Econometrics Journal 6(1):217–259
Pradhan JP, Singh N (2008) Outward FDI and knowledge flows: a study of the Indian automotive sector. International Journal of Institutions and Economies 1(1):155–186
Raskin PD (1995) Methods for estimating the population contribution to environmental change. Ecological economics 15(3):225–233
Romer PM (1990). Endogenous technological change. Journal of political Economy, 98(5, Part 2), S71-S102
Rosa EA, Dietz T (1998) Climate change and society: speculation, construction and scientific investigation. International sociology 13(4):421–455
Sachs G (2003). Dreaming with BRICs: the path to 2050. New York, Global Economics Paper No. 99.
Sahoo M, Sethi N (2021a) The dynamic impact of urbanisation, structural transformation, and technological innovation on ecological footprint and PM2.5: evidence from newly industrialised countries, Environment, Development and Sustainability, doi: 10.1007/s10668-021-01614-7
Sahoo M, Sethi N (2021b) The intermittent effects of renewable energy on ecological footprint: evidence from develo** countries. Environmental Sciences and Pollution Research. https://doi.org/10.1007/s11356-021-14600-3
Sahoo M, Sethi N (2020) Impact of industrialization, urbanization and financial development on energy consumption: empirical evidence from India. J Public Affairs. 20(3). https://doi.org/10.1002/pa.2089
Salahuddin M, Gow J (2016) The effects of Internet usage, financial development and trade openness on economic growth in South Africa: a time series analysis. Telematics and Informatics 33(4):1141–1154
Salahuddin M, Alam K, Ozturk I, Sohag K (2018) The effects of electricity consumption, economic growth, financial development and foreign direct investment on CO2 emissions in Kuwait. Renewable and Sustainable Energy Reviews 81:2002–2010
Samah IHA, Abd Rashid IM, Husain WAFW, Lskandar S, Abdullah MFS, Amlus MH (2021) Government expenditure, manufacturing growth, C02 emission: a causality analysis in Malaysia. International Journal of Energy Economics and Policy 11(1):373
Santra S (2017) The effect of technological innovation on production-based energy and CO2 emission productivity: evidence from BRICS countries. African Journal of Science, Technology, Innovation and Development 9(5):503–512
Shahbaz M, Khraief N, Uddin GS, Ozturk I (2014) Environmental Kuznets curve in an open economy: a bounds testing and causality analysis for Tunisia. Renewable and Sustainable Energy Reviews 34:325–336
Shahbaz M, Sinha A, Kontoleon A (2020). Decomposing scale and technique effects of economic growth on energy consumption: Fresh evidence from develo** economies. International Journal of Finance & Economics
Shahbaz M, Zakaria M, Shahzad SJH, Mahalik MK (2018) The energy consumption and economic growth nexus in top ten energy-consuming countries: fresh evidence from using the quantile-on-quantile approach. Energy Economics 71:282–301
Sinha A, Shahbaz M, Balsalobre D (2017) Exploring the relationship between energy usage segregation and environmental degradation in N-11 countries. Journal of Cleaner Production 168:1217–1229
Su HN, Moaniba IM (2017) Does innovation respond to climate change? Empirical evidence from patents and greenhouse gas emissions. Technological Forecasting and Social Change 122:49–62
Sulemana I, James HS Jr, Valdivia CB (2016) Perceived socioeconomic status as a predictor of environmental concern in African and developed countries. Journal of Environmental Psychology 46:83–95
Tiwari AK, Shahbaz M, Hye QMA (2013) The environmental Kuznets curve and the role of coal consumption in India: cointegration and causality analysis in an open economy. Renewable and Sustainable Energy Reviews 18:519–527
Topcu M, Payne JE (2018) Further evidence on the trade-energy consumption nexus in OECD countries. Energy Policy 117:160–165
Ullah S, Akhtar P, Zaefarian G (2018) Dealing with endogeneity bias: the generalised method of moments (GMM) for panel data. Industrial Marketing Management 71:69–78
UNCTAD, G. (2016). World Investment Report: Investor nationality: policy challenges. Geneva: UNCTAD, 1-232
UNCTAD, U. (2017). World Investment Report 2017: investment and the digital economy. In United Nations Conference on Trade and Development, United Nations, Geneva, 1-56
Vandenbussche J, Aghion P, Meghir C (2006) Growth, distance to frontier and composition of human capital. Journal of economic growth 11(2):97–127
Wang Q, Wang S (2019) Decoupling economic growth from carbon emissions growth in the United States: the role of research and development. Journal of Cleaner Production 234:702–713
Wang Q, Yang Z (2016) Industrial water pollution, water environment treatment, and health risks in China. Environmental Pollution 218:358–365
Wang X, Jiang D, Lang X (2017) Future extreme climate changes linked to global warming intensity. Science Bulletin 62(24):1673–1680
Wang X, Jiang D, Lang X (2018) Climate change of 4 C global warming above pre-industrial levels. Advances in Atmospheric Sciences 35(7):757–770
Wang Y, Liu SF (2008) The impact of OFDI on China's industrial structure--based on grey relational analysis. World Economic Research 4:61–62
Wang Y, Wang Y, Zhou J, Zhu X, Lu G (2011). Energy consumption and economic growth in China: A multivariate causality test. Energy Policy, 39(7), 4399-4406.a
Wang Z (2019) Does biomass energy consumption help to control environmental pollution? Evidence from BRICS countries. Science of the total environment 670:1075–1083
Westerlund J (2007) Testing for error correction in panel data. Oxford Bulletin of Economics and statistics 69(6):709–748
Windmeijer F (2005) A finite sample correction for the variance of linear efficient two-step GMM estimators. Journal of econometrics 126(1):25–51
Wu L, Liu S, Liu D, Fang Z, Xu H (2015) Modelling and forecasting CO2 emissions in the BRICS (Brazil, Russia, India, China, and South Africa) countries using a novel multi-variable grey model. Energy 79:489–495
**a Y, Zhang M, Song Z, Li J, Wu W (2020). The impact of OFDI reverse technology spillover effect on industrial structure upgrading. In IEIS2019 (pp. 367-380). Springer, Singapore
**e F, Zhang B (2021) Impact of China's outward foreign direct investment on green total factor productivity in "Belt and Road" participating countries: a perspective of institutional distance. Environmental Science and Pollution Research 28(4):4704–4715
**n D, Zhang Y (2020) Threshold effect of OFDI on China’s provincial environmental pollution. Journal of Cleaner Production 258:120608
Yang LG, Liu YN (2013). Can Japan's Outwards FDI Reduce its CO2 Emissions?: A new thought on polluter haven hypothesis. In Advanced Materials Research (Vol. 807, pp. 830-834). Trans Tech Publications Ltd
Yii KJ, Geetha C (2017) The nexus between technology innovation and CO2 emissions in Malaysia: evidence from granger causality test. Energy Procedia 105:3118–3124
Yin J, Zheng M, Chen J (2015) The effects of environmental regulation and technical progress on CO2 Kuznets curve: an evidence from China. Energy Policy 77:97–108
Zakarya GY, Mostefa BELMOKADDEM, Abbes SM, Seghir GM (2015) Factors affecting CO2 emissions in the BRICS countries: a panel data analysis. Procedia Economics and Finance 26:114–125
Zhang N, Yu K, Chen Z (2017) How does urbanisation affect carbon dioxide emissions? A cross-country panel data analysis. Energy Policy 107:678–687
Zhao D, Fan F, Cheng J, Zhang Y, Wong KS, Chigrinov VG et al (2015) Light-emitting liquid crystal displays based on an aggregation-induced emission luminogen. Advanced Optical Materials 3(2):199–202
Zhu Q, Sarkis J, Lai KH (2007) Initiatives and outcomes of green supply chain management implementation by Chinese manufacturers. Journal of environmental management 85(1):179–189
Zhu S, Ye A (2018) Does the impact of China’s outward foreign direct investment on reverse green technology process differ across countries? Sustainability 10(11):3841
Acknowledgements
The authors are grateful to the editor in chief, Prof Roula Inglesi-Lotz, editor, and anonymous referees of the journal for their extremely useful suggestions for the improvement of this paper. The usual disclaimers apply.
Availability of data and materials
The datasets generated and/or analysed during the current study are available in the World Development Indicators (2019) and UNCTAD. Links: https://databank.worldbank.org/source/world-development-indicators#
Author information
Authors and Affiliations
Contributions
All the authors contributed to the study conception and design. Material preparation, data collection, and analysis were performed by S.M. and N.S. The first draft of the manuscript was written by S.M., and N.S. commented on previous versions of the same. All authors have read and approved the final manuscript.
Corresponding author
Ethics declarations
Ethics approval and consent to participate
Not applicable.
Consent for publication
Not applicable.
Competing interests
The authors declare that they have no competing interests.
Additional information
Responsible Editor: Roula Inglesi-Lotz
Publisher’s note
Springer Nature remains neutral with regard to jurisdictional claims in published maps and institutional affiliations.
Rights and permissions
About this article
Cite this article
Mohanty, S., Sethi, N. The energy consumption-environmental quality nexus in BRICS countries: the role of outward foreign direct investment. Environ Sci Pollut Res 29, 19714–19730 (2022). https://doi.org/10.1007/s11356-021-17180-4
Received:
Accepted:
Published:
Issue Date:
DOI: https://doi.org/10.1007/s11356-021-17180-4