Responsible artificial intelligence for measuring efficiency: a neural production specification
Authors (first, second and last of 5)
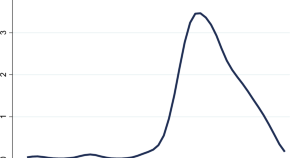
Collection
Artificial Intelligence (AI) is one of the most disruptive technologies in this decade that has started to transform both business organisations and societies in ways we could not have envisaged a few years ago. AI has become the key source of business model innovation, process transformation, re-engineering practices for gaining competitive advantage in organisations embracing data-centric, analytics, and digital culture. The impact of AI in transforming both businesses and societies is comparable to that of the Internet and World Wide Web (1990–2000) that led to the emergence of ecommerce, consume-centric practices, sharing economy, gig economy, digital manufacturing, and data-driven operations.
Despite the benefits offered by AI, machine learning (ML) algorithms currently lack transparency and explainability that makes it difficult for managers (or any other concerned user) to trust the output generated by these algorithms. In the context of transparency, it is a form of ideal that should facilitate revealing how data is integrated into an algorithm, processed by the algorithm and the knowledge that is gained using that data. The issue with explainability is that business managers do not usually understand how AI-based ML algorithms generate the outputs after processing the input data because the algorithm is either proprietary or that the mathematical computational models used in the algorithm are very complex to understand (even with technical expertise). In this context, literature has discussed that the time, effort, and resources invested by the organisations in AI systems has not fully translated into business value and productivity in majority of cases. Limited transparency and explainability of output responses generated by the AI systems has emerged as a key barrier to experiencing anticipated benefits by confidently turning data-centric decisions into effective actionable strategies.
The primary goal of embedding transparency within AI-based ML and deep learning models is to help the decision-making authorities understand what the AI system is doing, how it is generating the output responses, and why a particular response is generated. This will help these business users to confidently understand and assess the accuracy of the responses based on their own tacit domain expertise that will increase trust in these systems. The ability to get explanations for the output responses generated by AI algorithms can also potentially reduce biases in business processes, operations, and decision-making, thus enhancing both accountability and fairness. For example, gender discrimination in hiring employees and setting up credit card borrowing limits stemming from AI systems has led to mistrust among both businesses and their consumers, demonstrating the need for AI transparency. Furthermore, AI transparency can also aid in identifying and resolving flaws within ML models stemming from improper training datasets (input issues), wrong settings, configurations and hyperparameters (algorithmic issues), and overfitting or underfitting models that can help evolve these systems and therefore enhance the value offered.
While research into AI transparency, interpretability, and trust mechanisms is nascent (within Operations Research [OR]), following streams of research are gradually emerging in the literature: (1) techniques and mechanisms to embed transparency in AI models that can potentially alleviate concerns about the negative impact of AI such as bad decision-making, discrimination, bias, inaccurate recommendations; (2) impact/implications of embedding transparency to data-driven decision making and accountability of people/organisational body making these operational decisions; (3) what are the skills, operational strategies, changes and policies required to facilitate responsible usage of AI applications in operational decision-making; (4) what are the drivers and barriers to embed transparency within AI models and applications from different perspectives (businesses, consumers, employees, supply chain partners and other stakeholders involved, e.g., how enhancing AI transparency can impact supply chain culture and relationships).
There is limited consensus on the best practices, tools, and mechanisms to embed transparency in AI-based systems, and the impact of introducing such mechanisms on organisational operational processes, dynamics, supply chain partnership, supply chain culture, multi-stake holder collaboration in supply chain, and gaining competitive advantage). Embedding transparency in AI algorithms (such as machine learning and deep learning) to enhance interpretability, accountability, and robustness of data-driven decision-making (leveraging AI and analytics) represents an unknown and unexplored territory for both researchers and practitioners. This presents an important challenge and opportunity for the community to advance scholarship of AI transparency and explainability in OR. However, research reported in the field of OR (and wider business and operations management literature) on this theme (conceptual, theoretical, and empirical) is extremely limited.
Considering these knowledge gaps, we invite high-quality submissions addressing theoretical and algorithmic developments, providing evidence-based empirical cases and insights, advancing theory, practice, and methodology of AI transparency scholarship in operations research. The special issue will also consider real-world innovative implementation of tools, techniques, and mechanisms to enhance interpretability of AI outputs for both business organisations and society in areas such as supply chain management, operations management, service operations, transformative marketing operations, industrial management and production systems, supply chain finance, reverse logistics, and sustainability. Review papers and conceptual papers without any empirical evidence are beyond the scope of this special issue. However, the special issue will consider demonstrators relevant to OR (corresponding to the theme) making considerable contributions to both theory and practice. Topics of contributions corresponding to the theme of the special issue, AI Transparency, include (but are not limited to):
• Transparency in various stages of data analytics
• Mechanisms to AI transparency (e.g., model-agnostic interpretability methods)
• Tools for AI transparency (e.g., LIME, SHAP)
• Optimising AI algorithms by embedding transparency
• Models for accountability in AI-based data-driven decision-making
• Evaluating the performance of AI algorithms through transparency
• Methods for model interpretability in machine learning
• Implications of AI transparency on data-driven decision-making
• Implications of AI transparency on supply chain relationships and culture
• Implications of AI transparency on supply chain culture
• AI transparency and social sustainability
• AI transparency and supply chain resilience, supply chain diligence
• Role of visualisation in AI interpretability
• Role of AI transparency to algorithmic fairness and robustness
• Dark side of AI transparency for OR and OM
Please note: Manuscripts on the above topics should make a clear and unique contribution to OR through relevant references to OR literature.
University of Surrey, UK
TBS Business School, France