Geometric deep learning for enhanced quantitative analysis of microstructures in X-ray computed tomography data
Authors (first, second and last of 6)
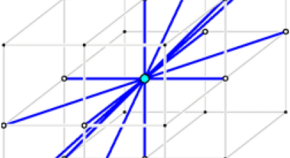
Collection
As manufacturing continues to digitize, machine learning and deep learning techniques have the potential to revolutionize quality assurance and control by leveraging seamlessly integrated data from production processes and quality assessments. Collectively known as “predictive quality”, manufacturing companies have the tools to make informed, data-driven predictions about product quality by leveraging the wealth of process data available to them. Thereby, the current state of research on predictive quality includes a variety of approaches suitable for different use cases and industries. These approaches range from inline real-time quality prediction using sensor data to automated downstream quality assessment using extensive measurement data. The range of data modalities used for these purposes is equally diverse and includes complex time series data as well as high-dimensional image and video data, possibly enriched with process-specific as well as domain knowledge. The goal of this collection is to bring together the latest research, methodologies, and outstanding applications in the field of data-driven AI-enabled quality prediction. Academics, industry experts, researchers, and practitioners alike are invited to submit state-of-the-art techniques, novel algorithms, theoretical insights, and practical applications in the form of original research papers, reviews, and case studies.
We welcome submissions addressing the following scopes, although submissions on related topics are also welcome: “Advanced machine learning and deep learning models for predictive quality”, “Integrating diverse data modalities into predictive quality systems”, “Challenges, opportunities, and future trends in data-driven AI-enabled predictive quality”, “Industry case studies demonstrating the impact of predictive quality on product quality, cost, and efficiency”, and “Ethical considerations and privacy in the context of data-driven AI-enabled predictive quality”.
Professor Tobias Meisen, University of Wuppertal, Germany. He is professor at the Institute for Technologies and Management of Digital Transformation at the University of Wuppertal since September 2018. Further, he is the director of the Interdisciplinary Centre for Data Analytics and Machine Learning. He has studied computer science and a Ph.D. in engineering. His research focuses on autonomous technical systems in industry, with an emphasis on deep and machine learning. He has authored/co-authored over a hundred scientific publications and is a regular reviewer for conferences and journals.
Dr Hasan Tercan, University of Wuppertal, Germany. He is a researcher and group leader in applied artificial intelligence at the Institute for Technologies and Management of Digital Transformation at the University of Wuppertal since December 2018. He has studied computer science at the Technical University in Darmstadt. His research deals with the development and realization of machine learning and artificial intelligence methods for industry, with a focus on machine learning-based predictive quality, intelligent scheduling and planning using reinforcement learning, and data-efficient AI using transfer learning and continual learning.