Abstract
It is common for state-of-the-art research to demand higher granularity data to effectively model the atmospheric composition and personal exposure to air pollution. With the advent of Low-Cost Sensors (LCS) technology, the potential of increased spatiotemporal monitoring resolution arises, however, low cost comes with reduced measurement quality. On-site calibration via supervised machine learning (ML) is the most promising technique for the operational calibration of such devices. This study aims (a) to introduce the distribution guided neural disaggregation (DGND) method to increase the temporal resolution of air quality (AQ) low frequency data based on LCS high frequency readings and (b) simultaneously learn a calibration function with the ability to infer over the hourly resolution but with daily supervision. Towards this two-fold objective we propose an indirect training loss based on the first and second distribution moments errors to optimize a multi-layer perceptron (MLP). DGNDs generalization performance is compared against a traditionally trained MLP with the same architecture on a withheld test set in terms of errors and linearity. Furthermore, using the same metrics, the disaggregation results are evaluated on the original time series from which the reference moments originated. Results suggest that modeling the disaggregated (hourly) resolution of PM10 and O3 concentrations is feasible from aggregated (daily) information indicated by modest to high linearity with coefficient of determination R2 between 0.57–0.69 on the test set (except Sindos PM10 where R2 < 0), and 0.49–0.83 on the original time series accompanied by moderate to low errors.
Access this chapter
Tax calculation will be finalised at checkout
Purchases are for personal use only
Similar content being viewed by others
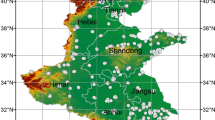
References
EUD (European Union Directive). Directive 2008/50/EC of the European Parliament and of the Council of 21 May 2008 on ambient air quality and cleaner air for Europe. Off. J. Eur. Union 2008, L152 (2008)
Castell, N.: Low-cost sensors and networks-Overview of current status by the Norwegian Reference Laboratory for Air Quality. NILU report 15/2020 (2020)
RIVM Data Portal. https://sensors.rivm.nl/dataportaal/. Accessed 05 Mar 2022
Peltier, R., et al.: An Update on Low-cost Sensors for the Measurement of Atmospheric Composition. WMO (2021)
Bigi, A., Mueller, M., Grange, S., Ghermandi, G., Hueglin, C.: Performance of NO, NO2 low cost sensors and three calibration approaches within a real world application. Atmosph. Measur. Techniq. 11(6) (2018)
Bagkis, E., Kassandros, T., Karteris, M., Karteris, A., Karatzas, K.: Analyzing and improving the performance of a particulate matter low cost air quality monitoring device. Atmosphere 12(2), 251 (2021)
Wapler, K., de Coning, E., Buzzi, M.: Nowcasting. Reference Module In Earth Systems And Environmental Sciences (2019)
Moauro, F., Savio, G.: Temporal disaggregation using multivariate structural time series models. Economet. J. 8(2), 214–234 (2005)
Banbura, M., Giannone, D., Modugno, M., Reichlin, L.: Now-casting and the real-time data flow. In: Elliott, G., Timmermann, A. (eds.) Handbook of Economic Forecasting, vol. 2 Part A, pp. 195–237. Elsevier (2013)
Samad, A., Obando Nuñez, D., Solis Castillo, G., Laquai, B., Vogt, U.: Effect of relative humidity and air temperature on the results obtained from low-cost gas sensors for ambient air quality measurements. Sensors 20(18), 5175 (2020)
Bagkis, E., Kassandros, T., Karatzas, K.: Learning calibration functions on the fly: hybrid batch online stacking ensembles for the calibration of low-cost air quality sensor networks in the presence of concept drift. Atmosphere 13(3), 416 (2022)
Yu, X., Shi, Y., Wang, T., Sun, X.: Dust-concentration measurement based on Mie scattering of a laser beam. PLoS ONE 12(8), e0181575 (2017)
Park, C., Fergus, J., Miura, N., Park, J., Choi, A.: Solid-state electrochemical gas sensors. Ionics 15(3), 261–284 (2009)
Di Antonio, A., Popoola, O., Ouyang, B., Saffell, J., Jones, R.: Develo** a relative humidity correction for low-cost sensors measuring ambient particulate matter. Sensors 18(9), 2790 (2018)
Perone, C., Calabrese, E., Cohen-Adad, J.: Spinal cord gray matter segmentation using deep dilated convolutions. Sci. Rep. 8(1) (2018)
Han, J., Liu, H., **ong, H., Yang, J.: Semi-supervised air quality forecasting via self-supervised hierarchical graph neural network. In: IEEE Transactions on Knowledge and Data Engineering, vol. 5966 (2022)
Jiang, X., Luo, Y., Zhang, B.: Prediction of PM2.5 concentration based on the LSTM-TSLightGBM variable weight combination model. Atmosphere, 12(9), 1211 (2021)
Elsayed, S., Thyssens, D., Rashed, A., Jomaa, H.S., Schmidt-Thieme, L.: Do We Really Need Deep Learning Models for Time Series Forecasting? https://arxiv.org/abs/2101.02118. Accessed 05 Mar 2022
Stats Exchange. https://stats.stackexchange.com/questions/213464/on-the-importance-of-the-i-i-d-assumption-in-statistical-learning. Accessed 05 Mar 2022
Hounmenou, C., Gneyou, K., Glele Kakaï, R: A Formalism of the General Mathematical Expression of Multilayer Perceptron Neural Networks. Preprint (2021)
Glorot, X., Bengio, Y.: Understanding the difficulty of training deep feedforward neural networks. In: Proceedings of the Thirteenth International Conference on Artificial Intelligence and Statistics, PMLR, vol. 9, pp. 249–256 (2010)
Ge, R., Huang, F., **, C., Yuan, Y.: Esca** From Saddle Points --- Online Stochastic Gradient for Tensor Decomposition. https://arxiv.org/abs/1503.02101. Accessed 05 Mar 2022
Maas, A.L.: Rectifier Nonlinearities Improve Neural Network Acoustic Models (2013)
Kingma, D.P., Ba, J.: Adam: A Method for Stochastic Optimization. https://arxiv.org/abs/1412.6980. Accessed 05 Mar 2022
Maleki, F., Muthukrishnan, N., Ovens, K., Reinhold, C., Forghani, R.: Machine learning algorithm validation. Neuroimag. Clin. N. Am. 30(4), 433–445 (2020)
Fan, K., Dhammapala, R., Harrington, K., Lamastro, R., Lamb, B., Lee, Y.: Development of a Machine Learning Approach for Local-Scale Ozone Forecasting: Application to Kennewick, WA. Frontiers In Big Data, vol. 5 (2022)
Giannakidou, P.A.: Study on aerosols PM10 concentrations in the region of Thessaloniki, M.Sc. Thesis, in Greek (2021). https://doi.org/10.26262/heal.auth.ir.335294
Athanasakis, E., Kassandros, Th., Karatzas, K.: Investigation of traffic and air pollution in Thessaloniki, Greece, under ordinary and COVID-19 pandemic conditions. In: Kamilaris, A., Wohlgemuth, V., Karatzas, K., Athanasiadis, I. (eds.) Environmental Informatics. New perspectives in Environmental Information Systems: Transport, Sensors, Recycling. Adjunct Proceedings of the 34th Edition of the EnviroInfo, Shaker Verlang, Kassel, Germany, pp. 84–92 (2020). ISBN:978-3-8440-7628-8
Acknowledgments
This research has been co-financed by the European Union and Greek national funds through the Operational Program Competitiveness, Entrepreneurship and Innovation, under the call RESEARCH—CREATE—INNOVATE. Project code Τ1ΕDΚ-01697; project name Innovative system for air quality monitoring and forecasting (KASTOM, www.air4me.eu, accessed on 28 January 2022).
Author information
Authors and Affiliations
Corresponding author
Editor information
Editors and Affiliations
Rights and permissions
Copyright information
© 2022 IFIP International Federation for Information Processing
About this paper
Cite this paper
Bagkis, E., Kassandros, T., Karatzas, K. (2022). Distribution Guided Neural Disaggregation of PM10 and O3 Hourly Concentrations from Daily Statistics and Low-Cost Sensors. In: Maglogiannis, I., Iliadis, L., Macintyre, J., Cortez, P. (eds) Artificial Intelligence Applications and Innovations. AIAI 2022. IFIP Advances in Information and Communication Technology, vol 647. Springer, Cham. https://doi.org/10.1007/978-3-031-08337-2_16
Download citation
DOI: https://doi.org/10.1007/978-3-031-08337-2_16
Published:
Publisher Name: Springer, Cham
Print ISBN: 978-3-031-08336-5
Online ISBN: 978-3-031-08337-2
eBook Packages: Computer ScienceComputer Science (R0)