Abstract
To supplement the research on the evaluation method of asphalt pavement texture, novel three-dimensional (3D) methods are proposed. First, pavement textures were measured in laboratory from asphalt mixture specimens using laser texture scanner (LTS), and the macro-texture and micro-texture were extracted based on spectrum analysis techniques. Then, macro-texture level evaluation indices f8mac and f9mac together with micro-texture level evaluation indices f8mic and f9mic were proposed based on the gray level co-occurrence matrix (GLCM) method, and the hyperparameters existing in GLCM were discussed. Through the correlation analysis with mean texture depth (MTD) measured by sand patch method (SPM) and friction coefficient µ measured by walking friction tester (WFT), the optimum pavement texture level evaluation indices were determined. Additionally, the evaluation index σ of distribution uniformity of pavement texture (DUPT) was proposed based on the uniformity of deviations between sub-surfaces and the average surface of pavement texture. Finally, the correlations of σ with texture profiles were studied. The results show that f8mac and f8mic are the optimum indices for pavement texture level. MTD has significant correlation with f8mac, and the correlation coefficient R is 0.9348; friction coefficient µ has significant correlation with f8mic, and the R is 0.8030. The hyperparameters of GLCM selected in this study were proved effective. Moreover, the effectiveness of σ is also validated by calibrating with standard grooved surface. It can be concluded that the proposed indices in this study are suitable to the evaluation of pavement texture level and pavement texture distribution.
Access this chapter
Tax calculation will be finalised at checkout
Purchases are for personal use only
Similar content being viewed by others
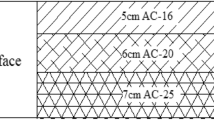
References
Fwa TF (2017) Skid resistance determination for pavement management and wet-weather road safety. Int J Transp Sci Technol 6(3):217–227
Chen D (2015) Study on image-based texture analysis method and prediction of skid-resistance & tire/pavement noise reduction of HMA. Chang’an University (In Chinese)
Del Pizzo A, Teti L, Moro A, Bianco F, Fredianelli L, Licitra G (2020) Influence of texture on tyre road noise spectra in rubberized pavements. Appl Acoust 159
ISO13473-1 (2019) Characterization of pavement texture by use of surface profiles—Part 1: determination of mean profile depth
Alhasan A, White DJ, De Brabanter K (2016) Wavelet filter design for pavement roughness analysis. Comput-Aided Civ Infrastruct Eng 31(12):907–920
Sohaney RC, Rasmussen RO (2013) Pavement texture evaluation and relationships to rolling resistance at MnROAD. Department of Transportation, Research Services Section
Šernas O, Zofka A, Vaitkus A, Gražulytė J (2020) The effect of exposed aggregate concrete gradation on the texture characteristics and durability. Constr Build Mater 261:119921
Liu C, Li J, Gao J, Yuan D, Gao Z, Chen Z (2021) Three-dimensional texture measurement using deep learning and multi-view pavement images. Measurement 172:108828
Puzzo L, Loprencipe G, Tozzo C, D’Andrea A (2017) Three-dimensional survey method of pavement texture using photographic equipment. Measurement 111:146–157
Dong N, Prozzi JA, Ni F (2019) Reconstruction of 3D pavement texture on handling dropouts and spikes using multiple data processing methods. Sensors 19(2):278
Li W, Huyan J, Tighe SL, Shao N-N, Sun Z-Y (2019) An innovative primary surface profile-based three-dimensional pavement distress data filtering approach for optical instruments and tilted pavement model-related noise reduction. Road Mater Pavement Des 20(1):132–150
Peng J, Chu L, Fwa T (2020) Determination of safe vehicle speeds on wet horizontal pavement curves. Road Mater Pavement Des 1–13
Zhang Z, Luan B, Liu X, Zhang M (2020) Effects of surface texture on tire-pavement noise and skid resistance in long freeway tunnels: from field investigation to technical practice. Appl Acoust 160:107120
Chen D, Ling C, Wang T, Su Q, Ye A (2018) Prediction of tire-pavement noise of porous asphalt mixture based on mixture surface texture level and distributions. Constr Build Mater 173:801–810
ISO13473-4 (2008) Characterization of pavement texture by use of surface profiles—Part 4: spectral analysis of texture profiles
Chen D (2020) Evaluating asphalt pavement surface texture using 3D digital imaging. Int J Pavement Eng 21(4):416–427
Ren WY (2019) Study on the abrasion characteristic of surface texture and its effect on noise for asphalt pavements (PhD). Chang’an University (In Chinese)
Zhang K, Zhang Z, Luo Y, Huang S (2017) Accurate detection and evaluation method for aggregate distribution uniformity of asphalt pavement. Constr Build Mater 152:715–730
Lan ZZ (2017) Study on surface texture structure and anti-skid performance of asphalt pavement based on digital image technology. Chongqing Jiaotong University, Chongqing
Song ZY, Yan GX, Sui YQ, Li FC (2014) Texture structure distribution of asphalt pavement surface based on digital image processing technology. J Central South Univ (Sci Technol) 45(11):4075–4080
Liu Q, Shalaby A (2015) Relating concrete pavement noise and friction to three-dimensional texture parameters. Int J Pavement Eng 18(5–6):450–458
Miao Y, Wang L, Wang X, Gong X (2015) Characterizing asphalt pavement 3-D macrotexture using features of co-occurrence matrix. Int J Pavement Res Technol
Miao Y, Wu J, Hou Y, Wang L, Yu W, Wang S (2019) Study on asphalt pavement surface texture degradation using 3-D image processing techniques and entropy theory. Entropy 21(2)
Haralick RM, Shanmugam K, Dinstein IH (1973) Textural features for image classification. IEEE Trans Syst Man Cybernet SMC-3(6):610–621
Jain S, Gruteser M (2019) Recognizing Textures with Mobile Cameras for Pedestrian Safety Applications. IEEE Trans Mob Comput 18(8):1911–1923
Katicha SW, Mogrovejo DE, Flintsch GW, de León Izeppi ED (2015) Adaptive spike removal method for high-speed pavement macrotexture measurements by controlling the false discovery rate. Transp Res Rec 2525(1):100–110
Clausi DA (2002) An analysis of co-occurrence texture statistics as a function of grey level quantization. Can J Remote Sens 28(1):45–62
Eleyan A, Demirel H (2011) Co-occurrence matrix and its statistical features as a new approach for face recognition. Turkish J Electr Eng Comput Sci 19(1):97–107
ISO13473–2 (2002) Characterization of pavement texture by use of surface profiles—Part 2: terminology and basic requirements related to pavement texture profile analysis
Han S, Liu M, Fwa TF (2018) Testing for low-speed skid resistance of road pavements. Road Mater Pavement Des 1–14
Author information
Authors and Affiliations
Corresponding author
Editor information
Editors and Affiliations
Rights and permissions
Copyright information
© 2022 The Author(s), under exclusive license to Springer Nature Switzerland AG
About this paper
Cite this paper
Dong, S., Han, S. (2022). Asphalt Pavement Texture Level and Distribution Uniformity Evaluation Using Three-Dimensional Method. In: Pasindu, H.R., Bandara, S., Mampearachchi, W.K., Fwa, T.F. (eds) Road and Airfield Pavement Technology. Lecture Notes in Civil Engineering, vol 193. Springer, Cham. https://doi.org/10.1007/978-3-030-87379-0_3
Download citation
DOI: https://doi.org/10.1007/978-3-030-87379-0_3
Published:
Publisher Name: Springer, Cham
Print ISBN: 978-3-030-87378-3
Online ISBN: 978-3-030-87379-0
eBook Packages: EngineeringEngineering (R0)