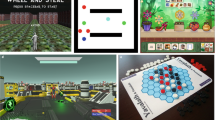
Overview
- Addresses the growing academic interest in PCGML
- Demonstrates common pitfalls in PCGML projects and how to avoid them
- Provides resources and guidance for starting new PCGML projects
Part of the book series: Synthesis Lectures on Games and Computational Intelligence (SLGCI)
Access this book
Tax calculation will be finalised at checkout
Other ways to access
About this book
Similar content being viewed by others
Keywords
Table of contents (13 chapters)
Authors and Affiliations
About the authors
Sam Snodgrass is an AI researcher at modl.ai, a game AI company focused on bringing state of the art game AI research from academia to the games industry. His research focuses on making PCGML more accessible to non-ML experts. This work includes making PCGML systems more adaptable and self-reliant, reducing the authorial burden of creating training data through domain blending, and building tools that allow for easier interactions with the underlying PCGML systems and their outputs. Through his work at modl.ai he has deployed several mixed-initiative PCGML tools into game studios to assist with level design and creation.
Adam Summerville is the lead AI engineer for Procedural Content Generation at The Molasses Flood, a CD Projekt studio. Prior to this, he was an assistant professor at California State Polytechnic University, Pomona. His research focuses on the intersection of artificial intelligence in games with a high-level goal of enabling experiences that would not be possible without artificial intelligence. This research ranges from procedural generation of levels, social simulation for games, and the use of natural language processing for gameplay. His work has been shown at the SF MoMA and SlamDance and won the audience choice award at IndieCade.
Bibliographic Information
Book Title: Procedural Content Generation via Machine Learning
Book Subtitle: An Overview
Authors: Matthew Guzdial, Sam Snodgrass, Adam J. Summerville
Series Title: Synthesis Lectures on Games and Computational Intelligence
DOI: https://doi.org/10.1007/978-3-031-16719-5
Publisher: Springer Cham
eBook Packages: Synthesis Collection of Technology (R0), eBColl Synthesis Collection 11
Copyright Information: The Editor(s) (if applicable) and The Author(s), under exclusive license to Springer Nature Switzerland AG 2022
Hardcover ISBN: 978-3-031-16718-8Published: 07 December 2022
Softcover ISBN: 978-3-031-16721-8Published: 07 December 2023
eBook ISBN: 978-3-031-16719-5Published: 06 December 2022
Series ISSN: 2573-6485
Series E-ISSN: 2573-6493
Edition Number: 1
Number of Pages: XIII, 238
Number of Illustrations: 19 b/w illustrations, 63 illustrations in colour
Topics: Machine Learning, Game Development, Artificial Intelligence, Computational Intelligence, Computer Science, general