Abstract
This study examines the impact of the mother wavelet, sensor selection, and machine learning (ML) models for smart fault diagnosis of rotating machines via discrete wavelet transform (DWT). The ability of Daubechies, Haar, Biorthogonal (Bior), Symlets (Sym), and Coiflets (Coif) wavelets is measured in terms of distinguishing imbalance, horizontal/vertical misalignment, and overhang/underhang bearing ball, cage, and outer race faults. For this purpose, single-step and two-step fault monitoring (SSFM and TSFM) approaches are proposed. In SSFM, the ML models detect the fault type by the healthy and faulty signals. In TSFM, the built models first determined whether the machine is faulty or not. If it is, then the models detect the fault type. As ML models, Random Forest (RF), AdaBoost with C4.5 (AB-C4.5), and two artificial neural network algorithms are trained by the features of DWT. Besides, the effect of the sensor type on the fault diagnosis is measured by considering the tachometer, microphone, and two accelerometers individually and combined. The results are interpreted regarding the evaluation metrics such as accuracy, precision, recall, confusion matrix, and model built time. It is concluded that Bior3.1 and Haar wavelets distinguish the fault type more accurately than other wavelets. Besides, the RF-Bior3.1 give the best results for SSFM and TSFM by accuracy values of 99.80% and 99.98%, respectively. It is also found that the sensor type is correlated with the selected mother wavelet.






















Similar content being viewed by others
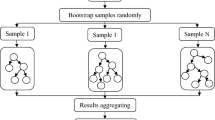
References
Mobley RK (2002) An introduction to predictive maintenance. Elsevier, Massachusetts, USA
Selcuk S (2017) Predictive maintenance, its implementation and latest trends. Proc Inst Mech Eng Part B J Eng Manuf 231:1670–1679. https://doi.org/10.1177/0954405415601640
Stenström C, Norrbin P, Parida A, Kumar U (2016) Preventive and corrective maintenance-cost comparison and cost-benefit analysis. Struct Infrastruct Eng 12:603–617. https://doi.org/10.1080/15732479.2015.1032983
Arunraj NS, Maiti J (2007) Risk-based maintenance-techniques and applications. J Hazard Mater 142:653–661. https://doi.org/10.1016/j.jhazmat.2006.06.069
Ahmad R, Kamaruddin S (2012) An overview of time-based and condition-based maintenance in industrial application. Comput Ind Eng 63:135–149. https://doi.org/10.1016/j.cie.2012.02.002
Jardine AKS, Lin D, Banjevic D (2006) A review on machinery diagnostics and prognostics implementing condition-based maintenance. Mech Syst Sig Process 20:1483–1510. https://doi.org/10.1016/j.ymssp.2005.09.012
Zonta T, da Costa CA, da RosaRighi R, de Lima MJ, da Trindade ES, Li GP (2020) Predictive maintenance in the Industry 4.0: a systematic literature review. Comput Ind Eng 150:106889. https://doi.org/10.1016/j.cie.2020.106889
Lee SM, Lee D, Kim YS (2019) The quality management ecosystem for predictive maintenance in the industry 4.0 era. Int J Qual Innov 5:4. https://doi.org/10.1186/s40887-019-0029-5
Ceruti A, Marzocca P, Liverani A, Bil C (2019) Maintenance in aeronautics in an industry 4.0 context: the role of augmented reality and additive manufacturing. J Comput Des Eng 6:516–526. https://doi.org/10.1016/j.jcde.2019.02.001
Li Z, Wang Y, Wang KS (2017) Intelligent predictive maintenance for fault diagnosis and prognosis in machine centers: industry 4.0 scenario. Adv Manuf 5:377–387. https://doi.org/10.1007/s40436-017-0203-8
Masoni R, Ferrise F, Bordegoni M, Gattullo M, Uva AE, Fiorentino M et al (2017) Supporting remote maintenance in industry 4.0 through augmented reality. Procedia Manuf 11:1296–1302. https://doi.org/10.1016/j.promfg.2017.07.257
Dalzochio J, Kunst R, Pignaton E, Binotto A, Sanyal S, Favilla J et al (2020) Machine learning and reasoning for predictive maintenance in industry 4.0: current status and challenges. Comput Ind 123:103298. https://doi.org/10.1016/j.compind.2020.103298
Paolanti M, Romeo L, Felicetti A, Mancini A, Frontoni E, Loncarski J (2018) Machine Learning approach for predictive maintenance in industry 4.0. IEEE, pp 1–6
Franciosi C, Iung B, Miranda S, Riemma S (2018) Maintenance for sustainability in the industry 4.0 context: a sco** literature review. IFAC-PapersOnLine 51:903–908. https://doi.org/10.1016/j.ifacol.2018.08.459
Rai VK, Mohanty AR (2007) Bearing fault diagnosis using FFT of intrinsic mode functions in Hilbert–Huang transform. Mech Syst Sig Process 21(6):2607–2615. https://doi.org/10.1016/j.ymssp.2006.12.004
Alameh K, Cité N, Hoblos G, Barakat G (2015) Vibration-based fault diagnosis approach for permanent magnet synchronous motors. IFAC-PapersOnLine 48(21):1444–1450. https://doi.org/10.1016/j.ifacol.2015.09.728. (9th IFAC Symposium on Fault Detection, Supervision andSafety for Technical Processes SAFEPROCESS 2015)
Janssens O, Schulz R, Slavkovikj V, Stockman K, Loccufier M, Van de Walle R et al (2015) Thermal image based fault diagnosis for rotating machinery. Infrared Phys Technol 73:78–87. https://doi.org/10.1016/j.infrared.2015.09.004
He D, Cao H, Wang S, Chen X (2019) Time-reassigned synchrosqueezing transform: the algorithm and its applications in mechanical signal processing. Mech Syst Sig Process 117:255–279. https://doi.org/10.1016/j.ymssp.2018.08.004
Bao W, Li F, Tu X, Hu Y, He Z (2020) Second-order synchroextracting transform with application to fault diagnosis. IEEE Trans Instrum Meas. https://doi.org/10.1109/TIM.2020.3045841
Yu K, Ma H, Han H, Zeng J, Li H, Li X et al (2019) Second order multi-synchrosqueezing transform for rub-impact detection of rotor systems. Mech Mach Theory 140:321–349. https://doi.org/10.1016/j.mechmachtheory.2019.06.007
Li Y, Wang Z, Zhao T, Song W (2021) An improved multi-ridge extraction method based on differential synchro-squeezing wavelet transform. IEEE Access 9:96763–96774. https://doi.org/10.1109/ACCESS.2021.3095054
Alpaydin E (2020) Introduction to machine learning. The Mit Press
Daş DB, Birant D (2021) Ordered physical human activity recognition based on ordinal classification. Turk J Electr Eng Comput Sci 29:2416–2436. https://doi.org/10.3906/elk-2010-75
Flah M, Nunez I, Chaabene WB, Nehdi ML (2021) Machine learning algorithms in civil structural health monitoring: a systematic review. Arch Comput Methods Eng 28:2621–2643. https://doi.org/10.1007/s11831-020-09471-9
Sajedi SO, Liang X (2021) Uncertainty-assisted deep vision structural health monitoring. Computer-Aided Civ Infrastruct Eng 36:126–142. https://doi.org/10.1111/mice.12580
Borzì L, Mazzetta I, Zampogna A, Suppa A, Olmo G, Irrera F (2021) Prediction of freezing of gait in Parkinson’s disease using wearables and machine learning. Sensors 21:614. https://doi.org/10.3390/s21020614
Jourdan T, Debs N, Frindel C (2021) The contribution of machine learning in the validation of commercial wearable sensors for gait monitoring in patients: a systematic review. Sensors 21:4808. https://doi.org/10.3390/s21144808
Chen W, Wang Q, Hesthaven JS, Zhang C (2021) Physics-informed machine learning for reduced-order modeling of nonlinear problems. J Comput Phys 446:110666. https://doi.org/10.1016/j.jcp.2021.110666
Faulon JL, Faure L (2021) In silico, in vitro, and in vivo machine learning in synthetic biology and metabolic engineering. Curr Opin Chem Biol 65:85–92. https://doi.org/10.1016/j.cbpa.2021.06.002
Hwang DH, Youn YW, Sun JH, Choi KH, Lee JH, Kim YH (2015) Support vector machine based bearing fault diagnosis for induction motors using vibration signals. J Electr Eng Technol 10:1558–1565. https://doi.org/10.5370/JEET.2015.10.4.1558
Tian J, Morillo C, Azarian MH, Pecht M (2016) Motor bearing fault detection using spectral kurtosis-based feature extraction coupled with K-nearest neighbor distance analysis. IEEE Trans Ind Electr 63:1793–1803. https://doi.org/10.1109/TIE.2015.2509913
Sánchez RV, Lucero P, Vásquez RE, Cerrada M, Macancela JC, Cabrera D (2018) Feature ranking for multi-fault diagnosis of rotating machinery by using random forest and KNN. J Intell Fuzzy Syst 34:3463–3473. https://doi.org/10.3233/JIFS-169526
Shah AK, Yadav A, Malik H (2018) EMD and ANN based intelligent model for bearing fault diagnosis. J Intell Fuzzy Syst 35:5391–5402. https://doi.org/10.3233/JIFS-169821
Zheng J, Pan H, Cheng J (2017) Rolling bearing fault detection and diagnosis based on composite multiscale fuzzy entropy and ensemble support vector machines. Mech Syst Sig Process 85:746–759. https://doi.org/10.1016/j.ymssp.2016.09.010
Liu Z, Guo W, Hu J, Ma W (2017) A hybrid intelligent multi-fault detection method for rotating machinery based on RSGWPT, KPCA and Twin SVM. ISA Trans 66:249–261. https://doi.org/10.1016/j.isatra.2016.11.001
Wei Y, Li Y, Xu M, Huang W (2019) A review of early fault diagnosis approaches and their applications in rotating machinery. Entropy. https://doi.org/10.3390/e21040409
de Jesus Romero-Troncoso R (2017) Multirate signal processing to improve FFT-based analysis for detecting faults in induction motors. IEEE Tran Ind Inform 13:1291–1300. https://doi.org/10.1109/TII.2016.2603968
Dhamande LS, Chaudhari MB (2018) Compound gear-bearing fault feature extraction using statistical features based on time-frequency method. Measurement 125:63–77. https://doi.org/10.1016/j.measurement.2018.04.059
** X, Fan J, Chow TWS (2019) Fault detection for rolling-element bearings using multivariate statistical process control methods. IEEE Trans Instrum Meas 68:3128–3136. https://doi.org/10.1109/TIM.2018.2872610
Kumar P, Hati AS (2020) Review on machine learning algorithm based fault detection in induction motors. Arch Comput Methods Eng 28(3):1929–1940. https://doi.org/10.1007/s11831-020-09446-w
Jian Y, Qing X, He L, Zhao Y, Qi X, Du M (2019) Fault diagnosis of motor bearing based on deep learning. Adv Mech Eng. https://doi.org/10.1177/1687814019875620
Liu R, Yang B, Zio E, Chen X (2018) Artificial intelligence for fault diagnosis of rotating machinery: a review. Mech Syst Sig Process 108:33–47. https://doi.org/10.1016/j.ymssp.2018.02.016
Katsifarakis N, Riga M, Voukantsis D, Karatzas K (2016) Computational intelligence methods for rolling bearing fault detection. J Braz Soc Mech Sci Eng 38:1565–1574. https://doi.org/10.1007/s40430-015-0458-6
Hu Q, Qin A, Zhang Q, He J, Sun G (2018) Fault diagnosis based on weighted extreme learning machine with wavelet packet decomposition and KPCA. IEEE Sens J 18:8472–8483. https://doi.org/10.1109/JSEN.2018.2866708
Guo MF, Zeng XD, Chen DY, Yang NC (2018) Deep-learning-based earth fault detection using continuous wavelet transform and convolutional neural network in resonant grounding distribution systems. IEEE Sens J 18:1291–1300. https://doi.org/10.1109/JSEN.2017.2776238
Gangsar P, Tiwari R (2019) Diagnostics of mechanical and electrical faults in induction motors using wavelet-based features of vibration and current through support vector machine algorithms for various operating conditions. J Braz Soc Mech Sci Eng 41:71. https://doi.org/10.1007/s40430-019-1574-5
Islam MMM, Kim JM (2019) Automated bearing fault diagnosis scheme using 2D representation of wavelet packet transform and deep convolutional neural network. Comput Ind 106:142–153. https://doi.org/10.1016/j.compind.2019.01.008
Yang L, Chen H (2019) Fault diagnosis of gearbox based on RBF-PF and particle swarm optimization wavelet neural network. Neural Comput Appl 31:4463–4478. https://doi.org/10.1007/s00521-018-3525-y
Zhang Y, Zhu D, Zhao L (2021) Fault diagnosis of rolling element bearing using ACYCBD based cross correlation spectrum. J Braz Soc Mech Sci Eng 43:447. https://doi.org/10.1007/s40430-021-02955-0
Gangsar P, Tiwari R (2020) Signal based condition monitoring techniques for fault detection and diagnosis of induction motors: a state-of-the-art review. Mech Syst Sig Process. https://doi.org/10.1016/j.ymssp.2020.106908
Shi Z, Yang X, Li Y, Yu G (2021) Wavelet-based synchroextracting transform: an effective TFA tool for machinery fault diagnosis. Control Eng Pract. https://doi.org/10.1016/j.conengprac.2021.104884
Han B, Zhou Y, Yu G (2021) Second-order synchroextracting wavelet transform for nonstationary signal analysis of rotating machinery. Sig Process. https://doi.org/10.1016/j.sigpro.2021.108123
Varanis M, Pederiva R (2017) The influence of the wavelet filter in the parameters extraction for signal classification: an experimental study. Proc Ser Braz Soc Comput Appl Math. https://doi.org/10.5540/03.2017.005.01.0501
Defdaf M, Berrabah F, Chebabhi A, Cherif BDE (2021) A new transform discrete wavelet technique based on artificial neural network for induction motor broken rotor bar faults diagnosis. Int Trans Electr Energy Syst. https://doi.org/10.1002/2050-7038.12807
Varanis M, Pederiva R (2018) Statements on wavelet packet energy-entropy signatures and filter influence in fault diagnosis of induction motor in non-stationary operations. J Braz Soc Mech Sci Eng. https://doi.org/10.1007/s40430-018-1025-8
Liang P, Deng C, Wu J, Yang Z (2020) Intelligent fault diagnosis of rotating machinery via wavelet transform, generative adversarial nets and convolutional neural network. Measurement 159:107768. https://doi.org/10.1016/j.measurement.2020.107768
Shukla R, Kankar PK, Pachori RB (2021) Automated bearing fault classification based on discrete wavelet transform method. Life Cycle Reliab Saf Eng 10:99–111. https://doi.org/10.1007/S41872-020-00151-Y
Lee KM, Vununu C, Moon KS, Lee SH, Kwon KR (2017) Automatic machine fault diagnosis system using discrete wavelet transform and machine learning. J Korea Multimed Soc 20:1299–1311
Malla C, Rai A, Kaul V, Panigrahi I (2019) Rolling element bearing fault detection based on the complex Morlet wavelet transform and performance evaluation using artificial neural network and support vector machine. Noise Vib Worldw 50:313–327. https://doi.org/10.1177/0957456519883280
Sharma S, Tiwari SK, Singh S (2021) Integrated approach based on flexible analytical wavelet transform and permutation entropy for fault detection in rotary machines. Measurement 169:108389. https://doi.org/10.1016/j.measurement.2020.108389
Tao X, Ren C, Wu Y, Li Q, Guo W, Liu R et al (2020) Bearings fault detection using wavelet transform and generalized Gaussian density modeling. Measurement 155:107557. https://doi.org/10.1016/j.measurement.2020.107557
Ding J, Ding C (2019) Automatic detection of a wheelset bearing fault using a multi-level empirical wavelet transform. Measurement 134:179–192. https://doi.org/10.1016/j.measurement.2018.10.064
Jha RK, Swami PD (2022) Failure prognosis of rolling bearings using maximum variance wavelet subband selection and support vector regression. J Braz Soc Mech Sci Eng 44:49. https://doi.org/10.1007/s40430-021-03345-2
Li G, Deng C, Wu J, Chen Z, Xu X (2020) Rolling bearing fault diagnosis based on wavelet packet transform and convolutional neural network. Appl Sci 10:770. https://doi.org/10.3390/app10030770
Narendiranath BT, Himamshu HS, Prabin KN, Rama PD, Nishant C (2017) Journal bearing fault detection based on Daubechies wavelet. Arch Acoust 42:401–414. https://doi.org/10.1515/aoa-2017-0042
Chen B, Shen B, Chen F, Tian H, **ao W, Zhang F et al (2019) Fault diagnosis method based on integration of RSSD and wavelet transform to rolling bearing. Measurement 131:400–411. https://doi.org/10.1016/j.measurement.2018.07.043
Wang Z, Zhang Q, **ong J, **ao M, Sun G, He J (2017) Fault diagnosis of a rolling bearing using wavelet packet denoising and random forests. IEEE Sens J 17(17):5581–5588. https://doi.org/10.1109/JSEN.2017.2726011
Saari J, Lundberg J, Odelius J, Rantatalo M (2018) Selection of features for fault diagnosis on rotating machines using random forest and wavelet analysis. Insight Non-Destr Test Cond Monit 60:434–442. https://doi.org/10.1784/insi.2018.60.8.434
Strömbergsson D, Marklund P, Berglund K, Saari J, Thomson A (2019) Mother wavelet selection in the discrete wavelet transform for condition monitoring of wind turbine drivetrain bearings. Wind Energy 22:1581–1592. https://doi.org/10.1002/WE.2390
Toma RN, Kim JM (2020) Article bearing fault classification of induction motors using discrete wavelet transform and ensemble machine learning algorithms. Appl Sci (Switz). https://doi.org/10.3390/APP10155251
Rafiee J, Rafiee MA, Tse PW (2010) Application of mother wavelet functions for automatic gear and bearing fault diagnosis. Expert Syst Appl 37:4568–4579. https://doi.org/10.1016/j.eswa.2009.12.051
Sharma A, Amarnath M, Kankar P (2014) Feature extraction and fault severity classification in ball bearings. J Vib Control 22:1–17. https://doi.org/10.1177/1077546314528021
Nikravesh SMY, Rezaie H, Kilpatrik M, Taheri H (2019) Intelligent fault diagnosis of bearings based on energy levels in frequency bands using wavelet and support vector machines (SVM). J Manuf Mater Process. https://doi.org/10.3390/jmmp3010011
Nath AG, Udmale SS, Singh SK (2021) Role of artificial intelligence in rotor fault diagnosis: a comprehensive review. Artif Intell Rev 54:2609–2668. https://doi.org/10.1007/s10462-020-09910-w
Chen R, Huang X, Yang L, Xu X, Zhang X, Zhang Y (2019) Intelligent fault diagnosis method of planetary gearboxes based on convolution neural network and discrete wavelet transform. Comput Ind 106:48–59. https://doi.org/10.1016/j.compind.2018.11.003
Yan J, Laflamme S, Singh P, Sadhu A, Dodson J (2020) A comparison of time-frequency methods for real-time application to high-rate dynamic systems. Vibration 3:204–216. https://doi.org/10.3390/vibration3030016
Tran H, Noori M, Altabey WA, Wu X (2017) Fault diagnosis of rotating machinery using wavelet-based feature extraction and support vector machine classifier. High Speed Mach. https://doi.org/10.1515/hsm-2017-0003
Burrus CS, Gopinath RA, Guo H (1998) Introduction to wavelets and wavelet transforms: a primer. Prentice Hall, New Jersey, USA
Minsky M, Papert S, Léon B (2017) Perceptrons: an introduction to computational geometry. The MIT Press, Massachusetts, USA
Breiman L (2001) Random forests. Mach Learn 45:5–32. https://doi.org/10.1023/A:1010933404324
Schapire RE (2013) Explaining adaboost. Empirical Inference: Festschrift in Honor of Vladimir N Vapnik, Heidelberg, Germany, pp 37–52. https://doi.org/10.1007/978-3-642-41136-6_5
Riberio FML.: MaFaulDa-machinery fault database. http://www02.smt.ufrj.br/~offshore/mfs/page_01.html#SEC1
Marins MA, Ribeiro FML, Netto SL, da Silva EAB (2018) Improved similarity-based modeling for the classification of rotating-machine failures. J Frankl Inst 355:1913–1930. https://doi.org/10.1016/j.jfranklin.2017.07.038
Yang X, Xue B, Jia L, Zhang H (2017) Quantitative analysis of pit defects in an automobile engine cylinder cavity using the radial basis function neural network-genetic algorithm model. Struct Health Monit 16:696–710. https://doi.org/10.1177/1475921716680591
Yang M, Sang YF, Liu C, Wang Z (2016) Discussion on the choice of decomposition level for wavelet based hydrological time series modeling. Water. https://doi.org/10.3390/w8050197
Nourani V, Komasi M, Mano A (2009) A multivariate ANN-wavelet approach for rainfall-runoff modeling. Water Resour Manag 23:2877. https://doi.org/10.1007/s11269-009-9414-5
Aussem A, Campbell J, Murtagh F (1998) Wavelet-based feature extraction and decomposition for financial forecasting. J Comput Intell Financ 3:5–12
Kaplun D, Voznesenskiy A, Romanov S, Nepomuceno E, Butusov D (2019) Optimal estimation of wavelet decomposition level for a matching pursuit algorithm. Entropy 21:843. https://doi.org/10.3390/e21090843
He H, Starzyk JA (2006) A self-organizing learning array system for power quality classification based on wavelet transform. IEEE Trans Power Deliv 21:286–295. https://doi.org/10.1109/tpwrd.2005.852392
Liu D, **ao Z, Hu X, Zhang C, Malik OP (2019) Feature extraction of rotor fault based on EEMD and curve code. Meas J Int Meas Confed 135:712–724. https://doi.org/10.1016/j.measurement.2018.12.009
Shukla S, Yadav RN, Sharma J, Khare S (2015) Analysis of statistical features for fault detection in ball bearing. In: 2015 IEEE international conference on computational intelligence and computing research (ICCIC), pp 1–7
Altinors A, Yol F, Yaman O (2021) A sound based method for fault detection with statistical feature extraction in UAV Motors. Appl Acoust 183:108325. https://doi.org/10.1016/j.apacoust.2021.108325
Refaeilzadeh P, Tang L, Liu H (2009) Cross-validation. Springer, US
Yi H, Jiang Q, Yan X, Wang B (2021) Imbalanced classification based on minority clustering synthetic minority oversampling technique with wind turbine fault detection application. IEEE Trans Ind Inform 17:5867–5875. https://doi.org/10.1109/TII.2020.3046566
Sharif I, Khare S (2014) Comparative analysis of Haar and daubechies wavelet for hyper spectral image classification. International Society for Photogrammetry and Remote Sensing, vol XL-8, pp 937–941
Lee BY, Tarng YS (1999) Application of the discrete wavelet transform to the monitoring of tool failure in end milling using the spindle motor current. Int J Adv Manuf Technol 15:238–243
Sharma P, Saxena A (2017) Critical investigations on performance of ANN and wavelet fault classifiers. Cogent Eng. https://doi.org/10.1080/23311916.2017.1286730
Chazal PD, Celler BG, Reilly RB (2000) Using wavelet coefficients for the classification of the electrocardiogram. In: Annual international conference of the IEEE engineering in medicine and biology-proceedings, vol 1, pp 64–67. https://doi.org/10.1109/IEMBS.2000.900669
Author information
Authors and Affiliations
Corresponding author
Additional information
Technical Editor: Samuel da Silva.
Publisher's Note
Springer Nature remains neutral with regard to jurisdictional claims in published maps and institutional affiliations.
Rights and permissions
Springer Nature or its licensor (e.g. a society or other partner) holds exclusive rights to this article under a publishing agreement with the author(s) or other rightsholder(s); author self-archiving of the accepted manuscript version of this article is solely governed by the terms of such publishing agreement and applicable law.
About this article
Cite this article
Das, O., Bagci Das, D. Smart machine fault diagnostics based on fault specified discrete wavelet transform. J Braz. Soc. Mech. Sci. Eng. 45, 55 (2023). https://doi.org/10.1007/s40430-022-03975-0
Received:
Accepted:
Published:
DOI: https://doi.org/10.1007/s40430-022-03975-0