Abstract
There is enormous scope and prospective of crop yield prediction at finer scale for both farm-level crop management as well as for crop insurance settlement at gram panchayat (GP) level in India. Now with the advent of satellite sensors like the MSI from Sentilnel-2 with fine spatial resolution, the possibility of generating frequent information on crop condition at field scale is increasing. This study demonstrated the combined use of high-resolution data from Sentinel-2 (10 m and 20 m); moderate-resolution data from MODIS (500 m) and coarser-resolution radiation data from INSAT-3D (4 km) for estimating yield of three major crops of India at GP and taluka level using a semi-physical model based on crop-specific radiation use efficiency. The novelty of this study lies in the data fusion approach using parameters from multiple spatial resolution of Geostationary and Lower Earth Orbiting satellites within the basic semi-physical model framework. The methodology has been demonstrated in Cuttack district of Odisha for rice; Rajkot district of Gujarat for cotton; and Indore district of MP and Fatehabad district of Haryana for wheat. We validated our result at GP, taluka and district level. At GP level, the root mean square error (RMSE) was found to be 16.5% for rice and 5.8% for wheat in Indore district. At taluka level, the RMSE was found to be 15%, 5.7%, 4.4% and 7.4% for rice, wheat in Indore district, wheat in Fatehabad district and cotton, respectively. The study concluded that high resolution remote sensing data would be of immense use for finer scale yield estimation, which can be aggregated at GP and taluka level with satisfactory accuracy (p = 95%).











Similar content being viewed by others
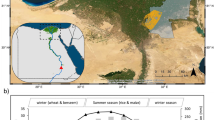
References
Aggarwal, P. K., Kalra, N., Chander, S., & Pathak, H. (2006a). InfoCrop: A dynamic simulation model for the assessment of crop yields, losses due to pests, and environmental impact of agro-ecosystems in tropical environments I. Model description. Agricultural Systems, 89, 1–25.
Aggarwal, P. K., Banerjee, B., Daryaei, M. G., Bhatia, A., Bala, A., Rani, S., Chander, S., Pathak, H., & Kalra, N. (2006b). InfoCrop: A dynamic simulation model for the assessment of crop yields, losses due to pests, and environmental impact of agro-ecosystems in tropical environments. II Performance of the model. Agricultural Systems, 89, 47–67.
Azzari, G., Jain, M., & Lobell, D. B. (2017). Towards fine resolution global maps of crop yields: testing multiple methods and satellites in three countries. Remote Sensing of Environment. https://doi.org/10.1016/j.rse.2017.04.014.
Bairagi, G. D., Chaudhari, K. N., Patidar, Manoj, Tripathy, R., Sharma, R., & Bhattacharya, B. K. (2018). Wheat yield simulation using modified Monteith model and geospatial data: A case study. Journal of Agrometeorology, 20(Special issue), 69–75.
Battude, M., Al Bitar, A., Morin, D., Cros, J., Huc, M., MaraisSicre, C., LeDantec, V., & Demarez, V. (2016). Estimating maize biomass and yield over large areas using high spatial and temporal resolution Sentinel-2 like remote sensing data. Remote Sensing of Environment, 184, 668–681. https://doi.org/10.1016/j.rse.2016.07.030.
Chaudhari, K. N. et al. (2019). Final report on Development of Standard Operationalized Procedure using Remote Sensing Technology for Optimization of CCEs.,/BPSG/PMFBY-02/201819.
CSO. (2008). Manual on area and crop production statistics. CSO-M-AG-, 01, 111p.
Directorate of Wheat Development, Ministry Of Agriculture (2016). Status paper on Wheat, Department of Agriculture and Cooperation, C.G.O. COMPLEX-I, 3 Floor, KAMLA NEHRU NAGAR GHAZIABAD- 201 002 (U.P.).
Dwivedi, M., Saxena, NS., & Ray, S. S. (2019). Assessment of rice biomass production and yield using semi- physical approach and remotely sensed data. The international archives of the photogrammetry, remote sensing and spatial information sciences, Volume XLII-3/W6, 2019 ISPRS-GEOGLAM-ISRS Joint Int. Workshop on “Earth Observations for Agricultural Monitoring”, 18–20 February 2019, New Delhi, India. https://doi.org/10.5194/isprs-archives-XLII-3-W6-217-2019.
El-Mohsen, A. A. A., & Amein, M. M. (2016). Study the relationships between seed cotton yield and yield component traits by different statistical techniques. International Journal of Agronomy and Agricultural Research, 8(5), 88–104.
Grassini, P., van Bussel, L. G. J., Van Wart, J., Wolf, J., Claessens, L., Yang, H., Boogaard, H., deGroot, H., vanIttersum, M. K., & Cassman, K. G. (2015). How good is good enough? Data requirements for reliable crop yield simulations and yield-gap analysis. Field Crop Research, 177, 49–63.
Gulati, A., Tewary, P., Hussain, S. (2018). Crop INSURANCE in India: Key Issues and Way Forward; Working paper no. 352; Indian Council for Research on International Economic Relations: New Delhi, India.
Hunt, M. L., Blackburn, G. A., Carrasco, L., & Redhead, J. W. (2019). High resolution wheat yield map** using Sentinel-2. Remote Sensing of Environment, 233, 111410. https://doi.org/10.1016/j.rse.2019.111410.
**, X., Kumar, L., Li, Z., Feng, H., Xu, X., Yang, G., & Wang, J. (2018). A review of data assimilation of remote sensing and crop models. European Journal of Agronomy, 92, 141–152. https://doi.org/10.1016/j.eja.2017.11.002.
**, Z., Azzari, G., & Lobell, D. B. (2017). Improving the accuracy of satellite-based high-resolution yield estimation: a test of multiple scalable approaches. Agricultural and Forest Meteorology, 247, 207–220. https://doi.org/10.1016/j.agrformet.2017.08.001.
**, Z., Azzaria, G., Youa, C., Tommasoa, S. D., Astonb, S., Burkea, M., & Lobell, D. B. (2019). Smallholder maize area and yield map** at national scales with Google Earth Engine. Remote Sensing of Environment, 228, 115–128. https://doi.org/10.1016/j.rse.2019.04.016.
Johnson, D. M. (2014). An assessment of pre- and within-season remotely sensed variables for forecasting corn and soybean yields in the United States. Remote Sensing of Environment, 141, 116–128. https://doi.org/10.1016/j.rse.2013.10.027.
Kanga, Y., & Özdoğanb, M. (2019). Field-level crop yield map** with Landsat using a hierarchical data assimilation approach. Remote Sensing of Environment, 228, 144–163. https://doi.org/10.1016/j.rse.2019.04.005.
Kiniry, J. R., Jones, C. A., O’toole, J. C., Blanchet, R., Cabelguenne, M., & Spanel, D. A. (1989). Radiation-use efficiency in biomass accumulation prior to grain-filling for five grain-crop species. Field Crops Research, 20(1), 51–64.
Kumar, S. N., Aggarwal, P. K., Saxena, R., Rani, S., Jain, S., & Chauhan, N. (2013). An assessment of regional vulnerability of rice to climate change in India. Climatic Change, 118, 683–699.
Lambert, M. J., Blaes, X., Traore, P. S., Defourny, P. (2017). Estimate yield at parcel level from S2 time series in sub-Saharan small holder farming systems. In 2017 9th international workshop on the analysis of work. Multitemporal Remote Sensing Images, Multi Temp 2017. https://doi.org/10.1109/Multi-Temp.2017.8035204.
Liu, F., Liu, X., Zhao, L., Ding, C., Jiang, J., & Wu, L. (2015). The dynamic assessment model for monitoring cadmium stress levels in rice based on the assimilation of remote sensing and the WOFOST model. IEEE JSTAR, 8, 1330–1338.
Lobell, D. B., Thau, D., Seifert, C., Engle, E., & Little, B. (2015). A scalable satellite-based crop yield mapper. Remote Sensing of Environment. https://doi.org/10.1016/j.rse.2015.04.021.
Marshall, M., Tu, K., & Brown, J. (2018). Optimizing a remote sensing production efficiency model for macro-scale GPP and yield estimation in agroecosystems. Remote Sensing of Environment, 217, 258–271. https://doi.org/10.1016/j.rse.2018.08.001.
McCarty, W., & Bowman, R. K. (2012). Cotton Yield Estimation (Un-published). https://extension.msstate.edu/sites/default/files/topic-files/cotton/estimating_yield.pdf.
Monteith, J. L. (1972). Solar radiation and productivity in tropical ecosystems. Journal of Applied Ecology, 9, 747–766.
Monteith, J. L., & Moss, C. J. (1977). Climate and the efficiency of crop production in Britain [and discussion]. Philosophical Transactions of the Royal Society of London B: Biological Sciences, 281(980), 277–294.
Moulin, S., Bondeau, A., & Delecolle, R. (1998). Combining agricultural crop models and satellite observations: from field to regional scales. International Journal of Remote Sensing, 19, 1021–1036. https://doi.org/10.1080/014311698215586.
Nagori, R., & Chaudhari, K. N. (2020). Development of high spatial resolution weather data using daily meteorological observations over Indian region. Mausam, 71(4), 605–616.
Patel, N. R., Bhattacharjee, B., Mohammed, A. J., Priya, T., & Saha, S. K. (2006). Remote sensing of regional yield assessment of wheat in Haryana, India. International Journal of Remote Sensing, 27(19), 4071–4090.
Patel, N. R., Mohammed, A. J., & Rakesh, D. (2005). Modeling of Regional wheat yields in western UP Using Multi-temporal Terra/MODIS satellite data. Geocarto International, 21(1), 43–50.
Patel, N. R., Saha, S. K., & Dadhwal, V. K. (2010). Evaluation of MODIS data Potential to infer water stress for wheat NPP estimation. Journal of International Society of Tropical Ecology, 51(1), 93–105.
Pathak, H., Parameswaran, C., Subudhi, H. N., Prabhukarthikeyan, S. R., Pradhan, S.K., & Anandan, A., et al. (2019). Rice varieties of NRRI: yield, quality, special traits and tolerance to biotic and abiotic stresses. NRRI Research Bulletin No.20, ICAR-National Rice Research Institute Indian Council of Agricultural Research Cuttack, Odisha 753006, (pp. 68).
Pinter, P. J., Kimball, B. A., Mauney, J. R., Hendrey, G. R., Lewin, K. F., & Nagy, J. (1994). Effects of free-air carbon dioxide enrichment on PAR absorption and conversion efficiency in cotton. Agricultural and Forest Meteorology, 70(1–4), 209–230. https://doi.org/10.1016/0168-1923(94)90059-0.
Prostko, E., Lemon, R., Cothren, J. T. (1998). Field estimation of cotton yields. SCS-1998–20, Texas Agril. Ext. Service, The Texas and A&M University System. http://publications.tamu.edu/COTTON/ PUB_cottonField%20Estimation%20of%20 Cotton%20Yields.pdf.
Raich, J. W., Rastetter, E. B., Melillo, J. M., Kicklighter, D. W., Steudler, P. A., Peterson, B. J., et al. (1991). Potential net primary productivity in South-America—application of a global-model. Ecological Applications, 1, 399–429.
Segarra, J., Buchaillot, M. L., Araus, J. L., & Kefauver, S. C. (2020). Remote sensing for precision agriculture: Sentinel-2 improved features and applications. Agronomy, 10, 641.
Shirsath, P. B., Sehgal, V. K., & Aggarwal, P. K. (2020). Agriculture, 10, 58. https://doi.org/10.3390/agriculture10030058.
Sibley, A. M., Grassini, P., Thomas, N. E., Cassman, K. G., & Lobell, D. B. (2014). Testingremote sensing approaches for assessing yield variability among maize fields. Agronomy Journal, 106, 24–32. https://doi.org/10.2134/agronj2013.0314.
Singh, A. K., Tripathy, R., & Chopra, U. K. (2008). Evaluation of CERES-Wheat and CropSyst models for water-nitrogen interactions in wheat crop. Agricultural Water Management, 95, 776–786.
Singh, P., & Kairon, M. S. (2019). Cotton varieties and hybrids. CICR Technical bulletin no: 13. Central Institute for Cotton Research Nagpur. https://www.cicr.org.in/pdf/cotton_varieties_hybrids.pdf
Singh, P. K., Singh, K. K., Bhan, S. C., Baxla, A. K., Singh, S., Rathore, L. S., & Gupta, A. (2017). Impact of projected climate change on rice (Oryza sativa L.) yield using CERES-rice model in different agroclimatic zones of India. Current Science, 112, 108–115.
Skakun, S., Vermote, E., Roger, J. C., & Franch, B. (2017). Combined use of landsat-8 and sentinel-2A images for winter crop map** and winter wheat yield assessment at regional scale. AIMS Geoscience, 3, 163–186. https://doi.org/10.3934/geosci.2017.2.163.
Stöckle, C., Martin, S., & Cambell, G. (1992). A model to assess environmental impact of crop** systems. American Society of Agricultural Enggneering, 92, 2041.
Thakur, M. R. (2020). Square formation, boll retention, yield and quality parameters of Bt and non-Bt cotton in relation to plant density and NPK levels. International Journal of Chemical Studies, 8(1), 2741–2753.
Timsina, J., Godwin, D., Humphreys, E., Kukal, S. S., & Smith, D. (2008). Evaluation of options for increasing yield and water productivity of wheat in Punjab, India using the DSSAT-CSM-CERES-Wheat model. Agricultural Water Management, 95, 1099–1110.
Tripathy, R., Chaudhari, K. N., Mukherjee, J., Ray, S. S., Patel, N., Panigrahy, S., & Parihar, J. S. (2013). Forecasting wheat yield in Punjab state of India by combining crop simulation model WOFOST and remotely sensed inputs. Remote Sensing. Letters, 4, 19–28.
Tripathy, R., Chaudhary, K. N., Nigam, R., Manjunath, K. R., Chauhan, P., Ray, S. S., & Parihar, J. S. (2014). Operational semi-physical spectral-spatial wheat yield model development. In The international archives of the photogrammetry, remote sensing and spatial information sciences, volume XL-8, 2014, ISPRS technical commission VIII symposium, 09–12 December 2014, Hyderabad, India (pp. 977–982).
Tripathy, R., Chaudhari, K. N., Ray, S. S. Nigam, R., & Bhattacharya, B. K. (2017). Forecasting wheat and mustard yield using GEO-LEO satellite sensor data. A Compendium of Research at SAC Jan 2015 to June 2017 (pp. 1030–1034).
Tsubo, M., & Walker, S. (2005). Relationships between photosynthetically active radiation and clearness index at Bloemfontein, South Africa. Theoritical and Applied Climatoly, 80, 17–25. https://doi.org/10.1007/s00704-004-0080-5.
Wilmot, C. J. (1982). Some comments on the evaluation of model performance. Bulletin of the American Meteorological Society, 64, 1309–1313.
**ao, X., Boles, S., Liu, J. Y., Zhuang, D. F., & Liu, M. L. (2002). Characterization of forest types in North eastern China, using multitemporal SPOT-4 VEGETATION sensor data. Remote Sensing of Environment, 82, 335–348.
Yadav, S., Li, T., Humphreys, E., Gill, G., & Kukal, S. S. (2011). Evaluation and application of ORYZA2000 for irrigation scheduling of puddled transplanted rice in north west India. Field Crops Research, 122, 104–117.
Acknowledgement
We are grateful to Director, Space Applications Centre, for his encouragement and keen interest in this study. Authors are grateful to Dr. Raj Kumar, former DD, EPSA, SAC for his support in this study. We acknowledge MNCFC, New Delhi for providing the rice crop mask at finer resolution, NASA (lpdaac.usgs.gov) for MODIS data, ESA (Copernicus Open Access Hub) for Sentinel-2A data.
Funding
The present research was carried out in the SUFALAM project at Space Applications Centre, Ahmedabad, India funded by Indian Space Research Organization, Bangalore, India.
Author information
Authors and Affiliations
Corresponding author
Ethics declarations
Conflict of interest
All authors declare no conflicts of interest in this paper.
Additional information
Publisher's Note
Springer Nature remains neutral with regard to jurisdictional claims in published maps and institutional affiliations.
About this article
Cite this article
Tripathy, R., Chaudhari, K.N., Bairagi, G.D. et al. Towards Fine-Scale Yield Prediction of Three Major Crops of India Using Data from Multiple Satellite. J Indian Soc Remote Sens 50, 271–284 (2022). https://doi.org/10.1007/s12524-021-01361-2
Received:
Accepted:
Published:
Issue Date:
DOI: https://doi.org/10.1007/s12524-021-01361-2