Abstract
Early Earthquake Warning (EEW) systems alarm about ongoing earthquakes to reduce their devastating human and financial damages. In complicated tasks like earthquake forecasting, Artificial Intelligence (AI) solutions show promising results. The goal of this review is to investigate the AI-based EEW systems. Web of Science, Scopus, Embase, and PubMed databases were systematically searched from its beginning until April 18, 2023. Studies that used AI algorithms to develop EEWs and forecast earthquake magnitude were qualified. The quality assessment was conducted using the Mixed Methods Assessment Tool version 2018. Detailed analysis was performed on 26 of 2604 retrieved articles. Researchers predict earthquakes most often using neural network family models (21 studies). Among eight categorized groups of parameters for earthquake forecasting, it was often predicted utilizing seismic wave characteristics (65.38%) and seismic activity data (61.54%). AI models most often predicted earthquake magnitude (32.69%) and depth (15.38%). Logistic Model Tree and Bayesian Network had the highest sensitivity, accuracy, and F-measure efficiency (99.9%). Findings showed that AI algorithms can forecast earthquakes. However, additional study is needed to determine the efficacy of more data-driven AI algorithms in mining seismic data using more input variables. This review is helpful for seismologists and researchers develo** EEW systems using AI.
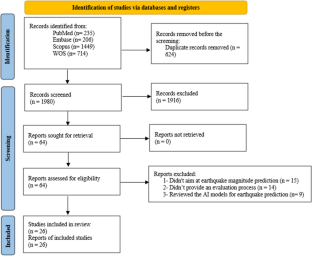






Similar content being viewed by others
Data availability
No datasets were generated or analysed during the current study.
References
Abdalzaher MS, Krichen M, Yiltas-Kaplan D, Ben Dhaou I, Adoni WYH (2023) Early detection of earthquakes using IoT and cloud infrastructure: a survey. Sustainability 15(15):11713. https://doi.org/10.3390/su151511713
Al Banna MH, Taher KA, Kaiser MS, Mahmud M, Rahman MS, Hosen AS, Cho GH (2020) Application of artificial intelligence in predicting earthquakes: state-of-the-art and future challenges. IEEE Access 8:192880–192923. https://doi.org/10.1109/ACCESS.2020.3029859
Allen CR (1976) Responsibilities in earthquake prediction: to the seismological society of America, delivered in Edmonton, Alberta, May 12, 1976. Bull Seismol Soc Am 66(6):2069–2074
Allen RM, Melgar D (2019) Earthquake early warning: advances, scientific challenges, and societal needs. Annual Rev Earth Planet Sci 47:361–388. https://doi.org/10.1146/annurev-earth-053018-060457
Anders A (2013) Real-time decision support in the face of emerging natural hazard events [Doctoral dissertation, Technical University of Denmark]. Official research database of the Technical University of Denmark. https://orbit.dtu.dk/en/publications/real-time-decision-support-in-the-face-of-emerging-natural-hazard
Asim K, Martínez-Álvarez F, Basit A, Iqbal T (2017) Earthquake magnitude prediction in Hindukush region using machine learning techniques. Nat Hazards 85:471–486. https://doi.org/10.1007/s11069-016-2579-3
Bejani MM, Ghatee M (2021) A systematic review on overfitting control in shallow and deep neural networks. Artif Intell Rev 54:6391–6438. https://doi.org/10.1007/s10462-021-09975-1
Berhich A, Belouadha F-Z, Kabbaj MI (2021) LSTM-based earthquake prediction: enhanced time feature and data representation. Int J High Perform Syst Archit 10(1):1–11. https://doi.org/10.1504/IJHPSA.2021.115499
Biau G, Scornet E (2016) A random forest guided tour. TEST 25:197–227. https://doi.org/10.1007/s11749-016-0481-7
Bilal MA, Ji Y, Wang Y, Akhter MP, Yaqub M (2022a) Early earthquake detection using batch normalization graph convolutional neural network (BNGCNN). Appl Sci 12(15):7548. https://doi.org/10.3390/app12157548
Bilal MA, Ji Y, Wang Y, Akhter MP, Yaqub M (2022b) An early warning system for earthquake prediction from seismic data using batch normalized graph convolutional neural network with attention mechanism (BNGCNNATT). Sensors 22(17):6482. https://doi.org/10.3390/s22176482
Burnett JW, Mothorpe C (2021) Human-induced earthquakes, risk salience, and housing values. Resour Energy Econ 63:101212. https://doi.org/10.1016/j.reseneeco.2020.101212
Chelidze T, Melikadze G, Kiria T, Jimsheladze T, Kobzev G (2020) Statistical and non-linear dynamics methods of Earthquake Forecast: application in the Caucasus. Front Earth Sci 8:194. https://doi.org/10.3389/feart.2020.00194
Cheng Z, Peng C, Chen M (2023) Real-time seismic intensity measurements prediction for earthquake early warning: a systematic literature review. Sensors 23(11):5052. https://doi.org/10.3390/s23115052
Chiang Y-J, Chin T-L, Chen D-Y (2022) Neural network-based strong motion prediction for on-site earthquake early warning. Sensors 22(3):704. https://doi.org/10.3390/s22030704
Chin T-L, Huang C-Y, Shen S-H, Tsai Y-C, Hu YH, Wu Y-M (2019) Learn to detect: improving the accuracy of earthquake detection. IEEE Trans Geoscience Remote Sens 57(11):8867–8878. https://doi.org/10.1109/TGRS.2019.2923453
Cochran ES, Kohler MD, Given DD, Guiwits S, Andrews J, Meier MA, Ahmad M, Henson I, Hartog R, Smith D (2018) Earthquake early warning ShakeAlert system: testing and certification platform. Seismol Res Lett 89(1):108–117. https://doi.org/10.1785/0220170138
Cremen G, Galasso C (2020) Earthquake early warning: recent advances and perspectives. Earth Sci Rev 205:103184. https://doi.org/10.1016/j.earscirev.2020.103184
Cremen G, Zuccolo E, Galasso C (2021) Accuracy and uncertainty analysis of selected methodological approaches to earthquake early warning in Europe. Seismological Soc Am 92(4):2321–2332
Dallo I, Marti M, Clinton J, Böse M, Massin F, Zaugg S (2022) Earthquake early warning in countries where damaging earthquakes only occur every 50 to 150 years–the societal perspective. Int J Disaster Risk Reduct 83:103441. https://doi.org/10.1016/j.ijdrr.2022.103441
Debnath P, Chittora P, Chakrabarti T, Chakrabarti P, Leonowicz Z, Jasinski M, Gono R, Jasińska E (2021) Analysis of earthquake forecasting in India using supervised machine learning classifiers. Sustainability 13(2):971. https://doi.org/10.3390/su13020971
Department SR (2023) Number of earthquakes worldwide 2000–2021. Available at https://www.statista.com/statistics/263105/development-of-the-number-of-earthquakes-worldwide-since-2000/. Accessed 13-09-2023
Dike HU, Zhou Y, Deveerasetty KK, Wu Q (2018) Unsupervised learning based on artificial neural network: a review. In: 2018 IEEE International Conference on Cyborg and Bionic Systems (CBS), IEEE
Dimililer K, Dindar H, Al-Turjman F (2021) Deep learning, machine learning and internet of things in geophysical engineering applications: an overview. Microprocessors Microsystems 80:103613. https://doi.org/10.1016/j.micpro.2020.103613
Essam Y, Kumar P, Ahmed AN, Murti MA, El-Shafie A (2021) Exploring the reliability of different artificial intelligence techniques in predicting earthquake for Malaysia. Soil Dyn Earthq Eng 147:106826. https://doi.org/10.1016/j.soildyn.2021.106826
Feng H, Miao Z, Hu Q (2022) Study on the uncertainty of machine learning model for earthquake-induced landslide susceptibility assessment. Remote Sens 14(13):2968. https://doi.org/10.3390/rs14132968
Galasso C, Zuccolo E, Aljawhari K, Cremen G, Melis NS (2023) Assessing the potential implementation of earthquake early warning for schools in the Patras region, Greece. Int J Disaster Risk Reduct 90:103610. https://doi.org/10.1016/j.ijdrr.2023.103610
Galkina A, Grafeeva N (2019) Machine learning methods for earthquake prediction: a survey. In: Proceedings of the Fourth Conference on Software Engineering and Information Management (SEIM-2019), Saint Petersburg, Russia
Gupta HK (2023) If a Magnitude∼ 8 earthquake occurs in India Today…. J Geol Soc India 99(3):299–302. https://doi.org/10.1007/s12594-023-2310-4
Gürsoy G, Varol A, Nasab A (2023) Importance of machine learning and deep learning algorithms in earthquake prediction: a review. In: 2023 11th International Symposium on Digital Forensics and Security (ISDFS), IEEE
Han Y, Zang Y, Meng L, Wang Y, Deng S, Ma Y, **e M (2022) A summary of seismic activities in and around China in 2021. Earthq Res Adv 2(3):100157. https://doi.org/10.1016/j.eqrea.2022.100157
He C, Huang Q, Bai X, Robinson DT, Shi P, Dou Y, Zhao B, Yan J, Zhang Q, Xu F (2021) A global analysis of the relationship between urbanization and fatalities in earthquake-prone areas. Int J Disaster risk Sci 12:805–820. https://doi.org/10.1007/s13753-021-00385-z
Heaton TH (1985) A model for a seismic computerized alert network. Science 228(4702):987–990. https://doi.org/10.1126/science.228.4702.987
Hong QN, Fàbregues S, Bartlett G et al (2018) The mixed methods appraisal tool (MMAT) version 2018 for information professionals and researchers. Educ Inf 34(4):285–291. https://doi.org/10.3233/EFI-180221
Jiao P, Alavi AH (2020) Artificial intelligence in seismology: advent, performance and future trends. Geosci Front 11(3):739–744. https://doi.org/10.1016/j.gsf.2019.10.004
Joshi A, Vishnu C, Mohan CK (2022) Early detection of earthquake magnitude based on stacked ensemble model. J Asian Earth Sciences: X 8:100122. https://doi.org/10.1016/j.jaesx.2022.100122
Kaftan I, Şalk M, Şenol Y (2017) Processing of earthquake catalog data of Western Turkey with artificial neural networks and adaptive neuro-fuzzy inference system. Arab J Geosci 10:1–9. https://doi.org/10.1007/s12517-017-3021-1
Kilb D, Bunn J, Saunders J, Cochran E, Minson S, Baltay A, O’Rourke C, Hoshiba M, Kodera Y (2021) The PLUM earthquake early warning algorithm: a retrospective case study of West Coast, USA, data. J Geophys Res: Solid Earth 126(7):e2020JB021053. https://doi.org/10.1029/2020JB021053
Liu Y, Yong S, He C, Wang X, Bao Z, **e J, Zhang X (2022) An earthquake Forecast Model based on Multi-station PCA Algorithm. Appl Sci 12(7):3311. https://doi.org/10.3390/app12073311
Mahtta R, Fragkias M, Güneralp B, Mahendra A, Reba M, Wentz EA, Seto KC (2022) Urban land expansion: the role of population and economic growth for 300 + cities. Npj Urban Sustain 2(1):5. https://doi.org/10.1038/s42949-022-00048-y
Marhain S, Ahmed AN, Murti MA, Kumar P, El-Shafie A (2021) Investigating the application of artificial intelligence for earthquake prediction in Terengganu. Nat Hazards 108:977–999. https://doi.org/10.1007/s11069-021-04716-7
Meier MA, Kodera Y, Böse M, Chung A, Hoshiba M, Cochran E, Minson S, Hauksson E, Heaton T (2020) How often can earthquake early warning systems alert sites with high-intensity ground motion? J Geophys Res: Solid Earth 125(2):e2019JB017718. https://doi.org/10.1029/2019JB017718
Mignan A, Broccardo M (2020) Neural network applications in earthquake prediction (1994–2019): Meta-analytic and statistical insights on their limitations. Seismol Res Lett 91(4):2330–2342. https://doi.org/10.1785/0220200021
Mousavi SM, Beroza GC (2020) Bayesian-deep-learning estimation of Earthquake Location from single-station observations. IEEE Trans Geosci Remote Sens 58(11):8211–8224. https://doi.org/10.1109/TGRS.2020.2988770
Mousavi SM, Sheng Y, Zhu W, Beroza GC (2019a) STanford EArthquake dataset (STEAD): a global data set of seismic signals for AI. IEEE Access 7:179464–179476. https://doi.org/10.1109/ACCESS.2019.2947848
Mousavi SM, Zhu W, Ellsworth W, Beroza G (2019b) Unsupervised clustering of seismic signals using deep convolutional autoencoders. IEEE Geosci Remote Sens Lett 16(11):1693–1697. https://doi.org/10.1109/LGRS.2019.2909218
Murwantara IM, Yugopuspito P, Hermawan R (2020) Comparison of machine learning performance for earthquake prediction in Indonesia using 30 years historical data. TELKOMNIKA: Telecommunication, Computing, Electronics and Control 18(3):1331–1342. https://doi.org/10.12928/TELKOMNIKA.v18i3.14756
Nakano M, Sugiyama D, Hori T, Kuwatani T, Tsuboi S (2019) Discrimination of seismic signals from earthquakes and tectonic tremor by applying a convolutional neural network to running spectral images. Seismol Res Lett 90(2A):530–538. https://doi.org/10.1785/0220180279
Patterson J, Gibson A (2017) Deep learning: a practitioner’s approach. O’Reilly Media Inc.
Perol T, Gharbi M, Denolle M (2018) Convolutional neural network for earthquake detection and location. Sci Adv 4(2):e1700578. https://doi.org/10.1126/sciadv.1700578
Pirmagomedov R, Blinnikov M, Amelyanovich A, Glushakov R, Loskutov S, Koucheryavy A, Kirichek R, Bobrikova E (2018) IoT based earthquake prediction technology. In: Internet of Things, Smart Spaces, and Next Generation Networks and Systems: 18th International Conference, NEW2AN 2018, and 11th Conference, ruSMART 2018, St. Petersburg, Russia, August 27–29, 2018, Proceedings 18 Springer
Rahmani AM, Azhir E, Ali S, Mohammadi M, Ahmed OH, Ghafour MY, Ahmed SH, Hosseinzadeh M (2021) Artificial intelligence approaches and mechanisms for big data analytics: a systematic study. PeerJ Comput Sci 7:e488. https://doi.org/10.7717/peerj-cs.488
Rajasekaran S, Pai GV (2017) Neural networks, fuzzy systems, and evolutionary algorithms: Synthesis and applications. PHI Learning Pvt. Ltd, New Delhi, pp 1–442
Rigatti SJ (2017) Random forest. J Insur Med 47(1):31–39. https://doi.org/10.17849/insm-47-01-31-39.1
Roelofs R, Shankar V, Recht B, Fridovich-Keil S, Hardt M, Miller J, Schmidt L (2019) A meta-analysis of overfitting in machine learning. In: Proceedings of the 33rd conference on neural information processing systems (NeurIPS 2019), pp 1–10. Vancouver. Retrieved from https://proceedings.neurips.cc/paper_files/paper/2019/file/ee39e503b6bedf0c98c388b7e8589aca-Paper.pdf
Ross ZE, Meier MA, Hauksson E, Heaton TH (2018) Generalized seismic phase detection with deep learning. Bull Seismol Soc Am 108(5A):2894–2901. https://doi.org/10.1785/0120180080
Ross ZE, Yue Y, Meier MA, Hauksson E, Heaton TH (2019) PhaseLink: a deep learning approach to seismic phase association. J Geophys Res: Solid Earth 124(1):856–869. https://doi.org/10.1029/2018JB016674
Sadhukhan B, Chakraborty S, Mukherjee S (2023) Predicting the magnitude of an impending earthquake using deep learning techniques. Earth Sci Inf 16(1):803–823. https://doi.org/10.1007/s12145-022-00916-2
Salam MA, Ibrahim L, Abdelminaam DS (2021) Earthquake prediction using hybrid machine learning techniques. Int J Adv Comput Sci Appl 12(5):654–665. https://doi.org/10.14569/IJACSA.2021.0120578
Samui P, Kim D (2014) Applicability of artificial intelligence to reservoir induced earthquakes. Acta Geophys 62(3):608–619. https://doi.org/10.2478/s11600-014-0201-1
Satriano C, Wu Y-M, Zollo A, Kanamori H (2011) Earthquake early warning: concepts, methods and physical grounds. Soil Dyn Earthq Eng 31(2):106–118. https://doi.org/10.1016/j.soildyn.2010.07.007
Seto KC, Fragkias M, Güneralp B, Reilly MK (2011) A meta-analysis of global urban land expansion. PLoS ONE 6(8):e23777. https://doi.org/10.1371/journal.pone.0023777
Thakur A, Konde A (2021) Fundamentals of neural networks. Int J Res Appl Sci Eng Technol 9:407–426
Thomas AM, Inbal A, Searcy J, Shelly DR, Bürgmann R (2021) Identification of low-frequency earthquakes on the San Andreas Fault with Deep Learning. Geophys Res Lett 48(13):e2021GL093157. https://doi.org/10.1029/2021GL093157
Vasti M, Dev A (2020) Classification and analysis of real-world earthquake data using various machine learning algorithms. In: Advances in Data Sciences, Security and Applications: Proceedings of ICDSSA 2019, Springer
Veroutsos E (2022) The World’s 10 Most Earthquake Prone Countries. Available at https://www.worldatlas.com/articles/the-world-s-10-most-earthquake-prone-countries.html. Accessed 17-09-2023
Wald DJ (2020) Practical limitations of earthquake early warning. Earthq Spectra 36(3):1412–1447. https://doi.org/10.1177/8755293020911388
Wang Q, Guo Y, Yu L, Li P (2020) Earthquakeprediction based on spatio-temporal data mining: an LSTM network approach. IEEE Trans Emerg Top Comput 8(1):148–158. https://doi.org/10.1109/TETC.2017.2699169
Wang Y, Li X, Wang Z, Liu J (2023) Deep learning for magnitude prediction in earthquake early warning. Gondwana Res 123:164–173
Yousefzadeh M, Hosseini SA, Farnaghi M (2021) Spatiotemporally explicit earthquake prediction using deep neural network. Soil Dyn Earthq Eng 144:106663. https://doi.org/10.1016/j.soildyn.2021.106663
Zhang X, Zhang M, Tian X (2021) Real-time earthquake early warning with deep learning: application to the 2016 M 6.0 Central Apennines, Italy earthquake. Geophys Res Lett 48(5):2020GL089394. https://doi.org/10.1029/2020GL089394
Zhang Y, Fung JF, Johnson KJ, Sattar S (2022) Review of seismic risk mitigation policies in earthquake-prone countries: lessons for earthquake resilience in the United States. J Earthquake Eng 26(12):6208–6235. https://doi.org/10.1080/13632469.2021.1911889
Zhao M, **ao Z, Chen S, Fang L (2023) DiTing: a large-scale Chinese seismic benchmark dataset for artificial intelligence in seismology. Earthq Sci 36(2):84–94. https://doi.org/10.1016/j.eqs.2022.01.022
Zhu W, Mousavi SM, Beroza GC (2019) Seismic signal denoising and decomposition using deep neural networks. IEEE Trans Geosci Remote Sens 57(11):9476–9488. https://doi.org/10.1109/TGRS.2019.2926772
Zhu J, Li S, Song J (2022) Magnitude estimation for earthquake early warning with multiple parameter inputs and a support vector machine. Seismol Res Lett 93(1):126–136. https://doi.org/10.1785/0220210144
Zuccolo E, Cremen G, Galasso C (2021) Comparing the performance of regional earthquake early warning algorithms in Europe. Front Earth Sci 9:686272. https://doi.org/10.3389/feart.2021.686272
Funding
This study has been funded and supported by Iranian Red Crescent Society.
Author information
Authors and Affiliations
Contributions
Seyed Mohammad Ayyoubzadeh conceptualized the research. Pirhossein Kolivand, Sharareh Rostam Niakan Kalhori and Peyman Saberian supervised the manuscript. Mozhgan Tanhapour, Fereshteh Karimi, Zohreh Javanmard, Soroush Heydari, Seyed Saeid Hoseini Talari, Seyed Mohsen Laal Mousavi, and Maryam Alidadi wrote the original draft. Mahnaz Ahmadi reviewed the manuscript.
Corresponding author
Ethics declarations
Competing interests
The authors declare no competing interests.
Additional information
Communicated by: H. Babaie
Publisher’s Note
Springer Nature remains neutral with regard to jurisdictional claims in published maps and institutional affiliations.
Appendix
Appendix
Rights and permissions
Springer Nature or its licensor (e.g. a society or other partner) holds exclusive rights to this article under a publishing agreement with the author(s) or other rightsholder(s); author self-archiving of the accepted manuscript version of this article is solely governed by the terms of such publishing agreement and applicable law.
About this article
Cite this article
Kolivand, P., Saberian, P., Tanhapour, M. et al. A systematic review of Earthquake Early Warning (EEW) systems based on Artificial Intelligence. Earth Sci Inform 17, 957–984 (2024). https://doi.org/10.1007/s12145-024-01253-2
Received:
Accepted:
Published:
Issue Date:
DOI: https://doi.org/10.1007/s12145-024-01253-2