Abstract
Manipulating intracellular signals by interaction with transmembranal G-protein-coupled receptors (GPCRs) is the way of action of more than 30% of available medicines. Designing molecules against GPCRs is most challenging due to their flexible binding orthosteric and allosteric pockets, a property that lead to different mode and extent of activation of intracellular mediators. Here, in the current study we aimed to design N-substituted tetrahydro-beta-carbolines (THβC’s) targeting Mu Opioid Receptors (MORs). We performed ligand docking study for reference and designed compounds against active and inactive states of MOR, as well as the active state bound to intracellular mediator of Gi. The reference compounds include 40 known agonists and antagonists, while the designed compounds include 25,227 N-substituted THβC analogues. Out of the designed compounds, 15 compounds were comparatively having better extra precision (XP) Gscore and were analyzed for absorption, distribution, metabolism, and excretion-toxicity (ADMET) properties, drug-likness, and molecular dynamic (MD) simulation. The results showed that N-substituted tetrahydro-beta-carbolines with and without C6-methoxy group substitutions (THBC/6MTHBC) analogues of A1/B1 and A9/B9 have relatively acceptable affinity and within pocket-stability toward MOR compared to the reference compounds of morphine (agonist) and naloxone (antagonist). Moreover, the designed analogues interact with key residue within the binding pocket of Asp 147 that is reported to be involved in receptor activation. In conclusion, the designed THBC analogues represent a good starting point for designing opioid receptor ligands other than morphinan scaffold, that have good synthetic accessibility which promotes feasible structural manipulation to tailor pharmacological effects with minimal side effects.
Graphical abstract

Workflow rational in the discovery of potential Mu Opioid Receptor ligands.









Similar content being viewed by others
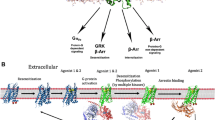
References
Mahmod Al-Qattan MN, Mordi MN (2019) Molecular basis of modulating adenosine receptors activities. Curr Pharm Des. https://doi.org/10.2174/1381612825666190304122624
Galligan JJSC (2016) Insights into the role of opioid receptors in the GI tract: experimental evidence and therapeutic relevance. Handb Exp Pharmacol 239:363–378. https://doi.org/10.1007/164_2016_116
Kaserer T, Steinacher T, Kainhofer R et al (2020) Identification and characterization of plant-derived alkaloids, corydine and corydaline, as novel mu opioid receptor agonists. Sci Rep 10:1–12. https://doi.org/10.1038/s41598-020-70493-1
Law PY, Reggio PH, Loh HH (2013) Opioid receptors: toward separation of analgesic from undesirable effects. Trends Biochem Sci 38:275–282. https://doi.org/10.1016/j.tibs.2013.03.003
Huang W, Manglik A, Venkatakrishnan AJ et al (2015) Structural insights into μ-opioid receptor activation. Nature 524:315–321. https://doi.org/10.1038/nature14886
Manglik A, Kruse AC, Kobilka TS et al (2012) Crystal structure of the μ-opioid receptor bound to a morphinan antagonist. Nature. https://doi.org/10.1038/nature10954
Koehl A, Hu H, Maeda S et al (2018) Structure of the μ-opioid receptor-Gi protein complex. Nature. https://doi.org/10.1038/s41586-018-0219-7
Mafi A, Kim S, Goddard WA (2020) Mechanism of β-arrestin recruitment by the μ-opioid G protein-coupled receptor. Proc Natl Acad Sci USA 117:16346–16355. https://doi.org/10.1073/pnas.1918264117
Trescot AM, Datta S, Lee M, Hans H (2008) Opioid pharmacology. Pain Physician 11:133–154. https://doi.org/10.1097/ajp.0000000000000710
Sum CS, Murphy BJ, Li Z et al (2019) Pharmacological characterization of GPCR agonists, antagonists, allosteric modulators and biased ligands from HTS hits to lead optimization. In: Markossian S, Sittampalam G, Grossman A (eds) Assay guidance manual. Eli Lilly & Company and the National Center for Advancing Translational Sciences, Bristol-Myers Squibb Company, NJ, pp 1–27
Wu Z, Hruby VJ (2019) Toward a universal μ-agonist template for template-based alignment modeling of opioid ligands. ACS Omega 4:17457–17476. https://doi.org/10.1021/acsomega.9b02244
Váradi A, Marrone GF, Palmer TC et al (2016) Mitragynine/corynantheidine pseudoindoxyls as opioid analgesics with mu agonism and delta antagonism, which do not recruit β-arrestin-2. J Med Chem 59:8381–8397. https://doi.org/10.1021/acs.jmedchem.6b00748
Wang J, Gong F, Liang T et al (2021) A review of synthetic bioactive tetrahydro-β-carbolines: a medicinal chemistry perspective. Eur J Med Chem 225:113815. https://doi.org/10.1016/j.ejmech.2021.113815
Kruegel AC, Gassaway MM, Kapoor A et al (2016) Synthetic and receptor signaling explorations of the mitragyna alkaloids: mitragynine as an atypical molecular framework for opioid receptor modulators. J Am Chem Soc 138:6754–6764. https://doi.org/10.1021/jacs.6b00360
Chakraborty S, Uprety R, Daibani AE et al (2021) Kratom alkaloids as probes for opioid receptor function: pharmacological characterization of minor indole and oxindole alkaloids from Kratom. ACS Chem Neurosci 12:2661–2678. https://doi.org/10.1021/acschemneuro.1c00149
Maity P, Adhikari D, Jana AK (2019) An overview on synthetic entries to tetrahydro-β-carbolines. Tetrahedron 75:965–1028. https://doi.org/10.1016/j.tet.2019.01.004
Jiang XL, Shen HW, Yu AM (2009) Pinoline may be used as a probe for CYP2D6 activity. Drug Metab Dispos 37:443–446. https://doi.org/10.1124/dmd.108.025056
Mohamad Arshad AS, Chear NJY, Ezzat MO et al (2020) Synthesis, characterization and crystal structure of new tetrahydro-β-carboline as acetylcholinesterase inhibitor. J Mol Struct. https://doi.org/10.1016/j.molstruc.2019.127070
Kalso E, Edwards JE, Moore RA, McQuay HJ (2004) Opioids in chronic non-cancer pain: systematic review of efficacy and safety. Pain 112:372–380. https://doi.org/10.1016/j.pain.2004.09.019
Morris GML-WM (2006) Molecular docking. In: Kukol AK (ed) Methods in molecular biology. Molecular modeling of proteins, vol 443. Human Press, Totowa, pp 365–382
Berman HM, Westbrook J, Feng Z et al (2000) The Protein Data Bank (www.rcsb.org). Nucleic Acids Res. https://doi.org/10.1093/nar/28.1.235
Banks JL, Beard HS, Cao Y et al (2005) Integrated modeling program, applied chemical theory (IMPACT). J Comput Chem 26:1752–1780. https://doi.org/10.1002/jcc.20292
Ellis CR, Kruhlak NL, Kim MT et al (2018) Predicting opioid receptor binding affinity of pharmacologically unclassified designer substances using molecular docking. PLoS ONE 13:1–18. https://doi.org/10.1371/journal.pone.0197734
Sterling T, Irwin JJ (2015) ZINC 15—ligand discovery for everyone. J Chem Inf Model. https://doi.org/10.1021/acs.jcim.5b00559
Molecular Operating Environment (MOE), 2019.0102 Chemical Computing Group ULC. Montreal
Friesner RA, Murphy RB, Repasky MP et al (2006) Extra precision glide: docking and scoring incorporating a model of hydrophobic enclosure for protein–ligand complexes. J Med Chem 49:6177–6196. https://doi.org/10.1021/jm051256o
Ding Y, Fang Y, Moreno J et al (2016) Assessing the similarity of ligand binding conformations with the Contact Mode Score. Comput Biol Chem 64:403–413. https://doi.org/10.1016/j.compbiolchem.2016.08.007
Daina A, Michielin O, Zoete V (2014) iLOGP: a simple, robust, and efficient description of n-octanol/water partition coefficient for drug design using the GB/SA approach. J Chem Inf Model 25:3284–3301. https://doi.org/10.1021/ci500467k
Daina A, Michielin O, Zoete V (2017) SwissADME: a free web tool to evaluate pharmacokinetics, drug-likeness and medicinal chemistry friendliness of small molecules. Sci Rep 7:1–13. https://doi.org/10.1038/srep42717
**ong G, Wu Z, Yi J et al (2021) ADMETlab 2.0: an integrated online platform for accurate and comprehensive predictions of ADMET properties. Nucleic Acids Res 49:W5–W14. https://doi.org/10.1093/nar/gkab255
Banerjee P, Eckert AO, Schrey AK, Preissner R (2018) ProTox-II: a webserver for the prediction of toxicity of chemicals. Nucleic Acids Res 46:W257–W263. https://doi.org/10.1093/nar/gky318
Bowers KJ, Chow E, Xu H et al (2006) Scalable algorithms for molecular dynamics simulations on commodity clusters. In: SC ’06: proceedings of the 2006 ACM/IEEE conference on supercomputing. IEEE, Tampa, pp 43–43
Lyman E, Higgs C, Kim B et al (2009) A role for a specific cholesterol interaction in stabilizing the Apo configuration of the human A2A adenosine receptor. Structure 17:1660–1668. https://doi.org/10.1016/j.str.2009.10.010
Mark P, Nilsson L (2001) Structure and dynamics of the TIP3P, SPC, and SPC/E water models at 298 K. J Phys Chem A 105:9954–9960. https://doi.org/10.1021/jp003020w
Genheden S, Ryde U (2015) The MM/PBSA and MM/GBSA methods to estimate ligand-binding affinities. Expert Opin Drug Discov 10:449–461. https://doi.org/10.1517/17460441.2015.1032936
Hauser AS, Kooistra AJ, Munk C et al (2021) GPCR activation mechanisms across classes and macro/microscales. Nat Struct Mol Biol 28:879–888. https://doi.org/10.1038/s41594-021-00674-7
Zou Y, Ewalt J, Ng HL (2019) Recent insights from molecular dynamics simulations for G protein-coupled receptor drug discovery. Int J Mol Sci. https://doi.org/10.3390/ijms20174237
Shahraki A, Işbilir A, Dogan B et al (2021) Structural and functional characterization of allatostatin receptor type-C of Thaumetopoea pityocampa, a potential target for next-generation pest control agents. J Chem Inf Model 61:715–728. https://doi.org/10.1021/acs.jcim.0c00985
Zhao Z, Huang T, Li J (2020) Molecular dynamics simulations to investigate how PZM21 affects the conformational state of the μ-opioid receptor upon activation. Int J Mol Sci 21:1–17. https://doi.org/10.3390/ijms21134699
Coimbra JRM, Baptista SJ, Dinis TCP et al (2020) Combining virtual screening protocol and in vitro evaluation towards the discovery of BACE1 inhibitors. Biomolecules. https://doi.org/10.3390/biom10040535
Lopez-Vallejo F, Caulfield T, Martinez-Mayorga K et al (2011) Integrating virtual screening and combinatorial chemistry for accelerated drug discovery. Comb Chem High Throughput Screen 14:475–487. https://doi.org/10.2174/138620711795767866
Jorgensen WL, Duffy EM (2002) Prediction of drug solubility from structure. Adv Drug Rev 54:355–366. https://doi.org/10.1016/S0169-409X(02)00008-X
Ghose AK, Viswanadhan VN, Wendoloski JJ (1999) A knowledge-based approach in designing combinatorial or medicinal chemistry libraries for drug discovery. 1. A qualitative and quantitative characterization of known drug databases. J Comb Chem 1:55–68. https://doi.org/10.1021/cc9800071
Sanchez-Covarrubias L, Slosky LM, Thompson BJ et al (2014) P-glycoprotein modulates morphine uptake into the CNS: a role for the non-steroidal anti-inflammatory drug diclofenac. PLoS ONE 9:1–11. https://doi.org/10.1371/journal.pone.0088516
Mahar Doan KM, Humphreys JE, Webster LO et al (2002) Passive permeability and P-glycoprotein-mediated efflux differentiate central nervous system (CNS) and non-CNS marketed drugs. J Pharmacol Exp Ther 303:1029–1037. https://doi.org/10.1124/jpet.102.039255
Lipinski CA (2000) Drug-like properties and the causes of poor solubility and poor permeability. J Pharmacol Toxicol Methods. https://doi.org/10.1016/S1056-8719(00)00107-6
Gudin J (2012) Opioid therapies and cytochrome P450 interactions. J Pain Symptom Manag 44:S4–S14. https://doi.org/10.1016/j.jpainsymman.2012.08.013
Overholser BR, Foster DR (2011) Opioid pharmacokinetic drug–drug interactions. Am J Manag Care 17:276–287
AlRabiah H, Ahad A, Mostafa GAE, Al-Jenoobi FI (2018) Effect of naltrexone hydrochloride on cytochrome P450 1A2, 2C9, 2D6, and 3A4 activity in human liver microsomes. Eur J Drug Metab Pharmacokinet 43:707–713. https://doi.org/10.1007/s13318-018-0482-x
Holmquist GL (2009) Opioid metabolism and effects of cytochrome P450. Pain Med 10:20–29. https://doi.org/10.1111/j.1526-4637.2009.00596.x
Raies AB, Bajic VB (2016) In silico toxicology: computational methods for the prediction of chemical toxicity. Wiley Interdiscip Rev Comput Mol Sci 6:147–172. https://doi.org/10.1002/wcms.1240
Zhang M, Park E-J, Kondratyuk TP et al (2018) Synthesis and structure-activity relationships of tetrahydro-β-carboline derivatives as anticancer and cancer-chemopreventive agents. Anticancer Res 38:4425–4433. https://doi.org/10.21873/anticanres.12744
Nie C, Li Q, Qiao Y et al (2020) Study on chemical modification and analgesic activity of N-(4-tert-butylphenyl)-4-(3-chloropyridin-2-yl) piperazine-1-carboxamide. Eur J Med Chem 194:112236. https://doi.org/10.1016/j.ejmech.2020.112236
Jaromin A, Gryzło B, Jamrozik M et al (2021) Synthesis, molecular docking and antiplasmodial activities of new tetrahydro-β-carbolines. Int J Mol Sci. https://doi.org/10.3390/ijms222413569Academic
Santos LHS, Ferreira RS, Caffarena ER (2019) Integrating molecular docking and molecular dynamics simulations. In: Methods in molecular biology. Humana, New York, pp 13–34
Podlewska S, Bugno R, Kudla L et al (2020) Molecular modeling of µ opioid receptor ligands patterns confronted with experimental data. Mol Pharmacol. https://doi.org/10.3390/molecules25204636
Ricarte A, Dalton JAR, Giraldo J (2021) Structural assessment of agonist efficacy in the μ-opioid receptor: morphine and fentanyl elicit different activation patterns. J Chem Inf Model 61:1251–1274. https://doi.org/10.1021/acs.jcim.0c00890
Liao S, Tan K, Floyd C et al (2021) Probing biased activation of mu-opioid receptor by the biased agonist PZM21 using all atom molecular dynamics simulation. Life Sci 269:119026. https://doi.org/10.1016/j.lfs.2021.119026
Ronsisvalle S, Panarello F, Spadaro A et al (2020) Pharmacological properties and biochemical mechanisms of μ-opioid receptor ligands might be due to different binding poses: MD studies. Future Med Chem 12:2001–2018. https://doi.org/10.4155/fmc-2020-0249
Li J, Chen C, Yin J et al (1999) ASP147 in the third transmembrane helix of the rat mu opioid receptor forms ion-pairing with morphine and naltrexone. Life Sci 65:175–185. https://doi.org/10.1016/s0024-3205(99)00234-9
Marmolejo-Valencia AF, Madariaga-Mazón A, Martinez-Mayorga K (2021) Bias-inducing allosteric binding site in mu-opioid receptor signaling. SN Appl Sci 3:1–16. https://doi.org/10.1007/s42452-021-04505-8
Manglik A (2020) Molecular basis of opioid action: from structures to new leads. Biol Psychiatry 87:6–14. https://doi.org/10.1016/j.biopsych.2019.08.028
Wang H, Hetzer F, Huang W et al (2022) Structure-based evolution of G protein-biased μ-opioid receptor agonists. Angew Chem Int Ed. https://doi.org/10.1002/anie.202200269
Kongsted J, Ryde U (2009) An improved method to predict the entropy term with the MM/PBSA approach. J Comput Aided Mol Des 23:63–71. https://doi.org/10.1007/s10822-008-9238-z
Porter SJ, Somogyi AA, White JM (2002) In vivo and in vitro potency studies of 6β-naltrexol, the major human metabolite of naltrexone. Addict Biol 7:219–225. https://doi.org/10.1080/135562102200120442
Mannelli P, Peindl KS, Wu L-T (2011) Pharmacological enhancement of naltrexone treatment for opioid dependence: a review. Subst Abuse Rehabil. https://doi.org/10.2147/SAR.S15853
Hou T, Wang J, Li Y, Wang W (2011) Assessing the performance of the molecular mechanics/Poisson Boltzmann surface area and molecular mechanics/generalized born surface area methods. II. The accuracy of ranking poses generated from docking. J Comput Chem 32:866–877. https://doi.org/10.1002/jcc.21666
Tuccinardi T (2021) What is the current value of MM/PBSA and MM/GBSA methods in drug discovery? Expert Opin Drug Discov 16:1233–1237. https://doi.org/10.1080/17460441.2021.1942836
Zhang X, Perez-sanchez H, Lightstone FC (2017) A comprehensive docking and MM/GBSA rescoring study of ligand recognition upon binding antithrombin. Curr Top Med Chem. https://doi.org/10.2174/1568026616666161117112604
Acknowledgements
We acknowledge the Schrodinger Licensing support team (licensing@schrodinger.com) for providing the thermal_mmgbsa.py and MOLSIS Inc. (www.molsis.co.jp) for providing Molecular Operating Environment (MOE, 2019.0102) software of 1-month free trail subscriptions.
Author information
Authors and Affiliations
Contributions
All authors reviewed the manuscript
Corresponding author
Ethics declarations
Conflict of interest
The authors declare no conflict of interest.
Ethical approval
This is a purely computational study and ethics approvals are not applicable.
Consent for publication
All co-authors have read and approved the manuscript.
Additional information
Publisher's Note
Springer Nature remains neutral with regard to jurisdictional claims in published maps and institutional affiliations.
Supplementary Information
Below is the link to the electronic supplementary material.
Rights and permissions
Springer Nature or its licensor (e.g. a society or other partner) holds exclusive rights to this article under a publishing agreement with the author(s) or other rightsholder(s); author self-archiving of the accepted manuscript version of this article is solely governed by the terms of such publishing agreement and applicable law.
About this article
Cite this article
Alananzeh, W.A., Al-qattan, M.N., Ayipo, Y.O. et al. N-substituted tetrahydro-beta-carboline as mu-opioid receptors ligands: in silico study; molecular docking, ADMET and molecular dynamics approach. Mol Divers (2023). https://doi.org/10.1007/s11030-023-10655-1
Received:
Accepted:
Published:
DOI: https://doi.org/10.1007/s11030-023-10655-1