Abstract
English speech incorporates numerous features associated with the speaker’s emotions, offering valuable cues for emotion recognition. This paper begins by briefly outlining preprocessing approaches for English speech signals. Subsequently, the Mel-frequency cepstral coefficient (MFCC), energy, and short-time zero-crossing rate were chosen as features, and their statistical properties were computed. The resulting 250-dimensional feature fusion was employed as input. A novel approach that combined gated recurrent unit (GRU) and a convolutional neural network (CNN) was designed for emotion recognition. The bidirectional GRU (BiGRU) method was enhanced through jump-joining to create a CNN-Skip-BiGRU model as an emotion recognition method for English speech. Experimental evaluations were conducted using the IEMOCAP dataset. The findings indicated that the fusion features exhibited superior performance in emotion recognition, achieving an unweighted accuracy rate of 70.31% and a weighted accuracy rate of 70.88%. In contrast to models like CNN-long short-term memory (LSTM), the CNN-Skip-BiGRU model demonstrated enhanced discriminative capabilities for different emotions. Moreover, it stood favorably against several existing emotion recognition methods. These results underscore the efficacy of the improved method in English speech emotion identification, suggesting its potential practical applications.
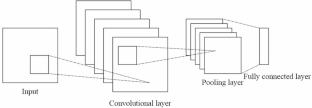



Similar content being viewed by others
Data availability
The data in this paper are available from the corresponding author.
References
Ahmed, M. R., Islam, S., Islam, A. M., & Shatabda, S. (2023). An ensemble 1D-CNN-LSTM-GRU model with data augmentation for speech emotion recognition. Expert Systems with Applications, 218, 119633.
Ayadi, S., & Lachiri, Z. (2022). Visual emotion sensing using convolutional neural network. Przeglad Elektrotechniczny, 98(3), 89–92.
Chattopadhyay, S., Dey, A., Singh, P. K., Ahmadian, A., & Sarkar, R. (2023). A feature selection model for speech emotion recognition using clustering-based population generation with hybrid of equilibrium optimizer and atom search optimization algorithm. Multimedia Tools and Applications, 82(7), 9693–9726.
Chen, Y., Liu, G., Huang, X., Chen, K., Hou, J., & Zhou, J. (2021). Development of a surrogate method of groundwater modeling using gated recurrent unit to improve the efficiency of parameter auto-calibration and global sensitivity analysis. Journal of Hydrology, 598(3), 1–16.
Guo, L., Wang, L., Dang, J., Chng, E. S., & Nakagawa, S. (2022). Learning affective representations based on magnitude and dynamic relative phase information for speech emotion recognition - ScienceDirect. Speech Communication, 136, 118–127.
Hansen, L., Zhang, Y. P., Wolf, D., Sechidis, K., Ladegaard, N., & Fusaroli, R. (2021). A generalizable speech emotion recognition model reveals depression and remission. Acta Psychiatrica Scandinavica, 145(2), 186–199.
Hu, D., Chen, C., Zhang, P., Li, J., Yan, Y., & Zhao, Q. (2021). A two-stage attention based modality fusion framework for multi-modal speech emotion recognition. IEICE Transactions on Information and Systems, E104.D(8), 1391–1394.
Hu, Z., Wang, L., Luo, Y., **a, Y., & **ao, H. (2022). Speech emotion recognition model based on attention CNN Bi-GRU fusing visual information. Engineering Letters, 30(2).
Hyder, H. (2021). The pedagogy of English language teaching using CBSE methodologies for schools. Advances in Social Sciences Research Journal, 8, 188–193.
Li, Z., Wang, S. H., Fan, R. R., Cao, G., Zhang, Y. D., & Guo, T. (2019). Teeth category classification via seven-layer deep convolutional neural network with max pooling and global average pooling. International Journal of Imaging Systems and Technology, 29(4), 577–583.
Liu, L. Y., Liu, W. Z., Zhou, J., Deng, H. Y., & Feng, L. (2022). ATDA: Attentional temporal dynamic activation for speech emotion recognition. Knowledge-based Systems, 243(May 11), 1–11.
Nfissi, A., Bouachir, W., Bouguila, N., & Mishara, B. L. (2022). CNN-n-GRU: End-to-end speech emotion recognition from raw waveform signal using CNNs and gated recurrent unit networks. In 21st IEEE international conference on machine learning and applications (ICMLA), (pp. 699–702).
Niu, D., Yu, M., Sun, L., Gao, T., & Wang, K. (2022). Short-term multi-energy load forecasting for integrated energy systems based on CNN-BiGRU optimized by attention mechanism. Applied Energy, 313, 1–17.
Ocquaye, E. N. N., Mao, Q., Xue, Y., & Song, H. (2021). Cross lingual speech emotion recognition via triple attentive asymmetric convolutional neural network. International Journal of Intelligent Systems, 36(1), 53–71.
Pandey, S. K., Shekhawat, H. S., & Prasanna, S. R. M. (2022). Attention gated tensor neural network architectures for speech emotion recognition. Biomedical Signal Processing and Control, 71(2), 1–16.
Peng, Z., Zhu, Z., Unoki, M., Dang, J., Akagi, M. (2018). Auditory-inspired end-to-end speech emotion recognition using 3D convolutional recurrent neural networks based on spectral-temporal representation. In 2018 IEEE international conference on, multimedia, & expo. (ICME) (pp. 1–6), San Diego, CA, USA.
Ponmalar, A., & Dhanakoti, V. (2022). Hybrid whale tabu algorithm optimized convolutional neural network architecture for intrusion detection in big data. Concurrency and Computation: Practice and Experience, 34(19), 1–15.
Qiao, D., Chen, Z. J., Deng, L., & Tu, C. L. (2022). Method for Chinese speech emotion recognition based on improved speech-processing convolutional neural network. Computer Engineering, 48(2), 281–290.
Requardt, A. F., Ihme, K., Wilbrink, M., & Wendemuth, A. (2020). Towards affect-aware vehicles for increasing safety and comfort: Recognising driver emotions from audio recordings in a realistic driving study. IET Intelligent Transport Systems, 14(10), 1265–1277.
Tan, M., Wang, C., Yuan, H., Bai, J., & An, L. (2020). FDA-MIMO Beampattern synthesis with Hamming window weighted linear frequency increments. International Journal of Aerospace Engineering, 2020(2), 1–8.
Tanko, D., Dogan, S., Demir, F. B., Baygin, M., Sahin, S. E., & Tuncer, T. (2022). Shoelace pattern-based speech emotion recognition of the lecturers in distance education: ShoePat23. Applied Acoustics, 190, 1–9.
Wibawa, I. D. G. Y. A., & Darmawan, I. D. M. B. A. (2021). Implementation of audio recognition using mel frequency cepstrum coefficient and dynamic time war** in wirama praharsini. Journal of Physics: Conference Series, 1722, 1–8.
Zhao, Z., Zheng, Y., Zhang, Z., Wang, H., Zhao, Y., & Li, C. (2018). Exploring spatio-temporal representations by integrating attention-based bidirectional-LSTM-RNNs and FCNs for speech emotion recognition. In Annual conference of the international speech communication association, (pp. 272–276).
Zhao, Z., Bao, Z., Zhao, Y., Zhang, Z., Cummins, N., Ren, Z., & Schuller, B. (2019). Exploring deep spectrum representations via attention-based recurrent and convolutional neural networks for Speech emotion recognition. IEEE Access: Practical Innovations, Open Solutions, 7, 97515–97525.
Zhu, M., Cheng, J., & Zhang, Z. (2021). Quality control of microseismic P-phase arrival picks in coal mine based on machine learning. Computers & Geosciences, 156, 1–12.
Funding
Not applicable.
Author information
Authors and Affiliations
Contributions
YYY conceived the idea for the study, did the analyses, and wrote the paper.
Corresponding author
Ethics declarations
Conflict of interest
The authors declare no conflict of interest.
Additional information
Publisher’s Note
Springer Nature remains neutral with regard to jurisdictional claims in published maps and institutional affiliations.
Rights and permissions
Springer Nature or its licensor (e.g. a society or other partner) holds exclusive rights to this article under a publishing agreement with the author(s) or other rightsholder(s); author self-archiving of the accepted manuscript version of this article is solely governed by the terms of such publishing agreement and applicable law.
About this article
Cite this article
Yang, Y. Feature fusion: research on emotion recognition in English speech. Int J Speech Technol (2024). https://doi.org/10.1007/s10772-024-10107-7
Received:
Accepted:
Published:
DOI: https://doi.org/10.1007/s10772-024-10107-7