Abstract
As one of China’s national urban agglomerations, the middle reaches of the Yangtze River urban agglomeration (MRYRA) has suffered from serious PM2.5 pollution for a considerable period. Using deep learning methods to fit the quantitative relationship between aerosol optical depth (AOD) and PM2.5 (AOD-PM2.5) has received extensive attention. In this study, based on the correlation between AOD and PM2.5 data, a new network model BiCNN is proposed by using the satellite-ground synergy method, aiming to obtain the spatial distribution of PM2.5 concentration in the MRYRA. Data from MRYRA 2019 is used for model training and testing. The results show that BiCNN exhibits the best estimation on the annual scale, with a fit coefficient R2 of 0.836 and the smallest error, RMSE of 6.746 and MAPE of 12.497 compared to the benchmark model. The model effectively improves the relationship between AOD-PM2.5. The spatial distribution of PM2.5 in the MRYRA from 2019 to 2022 by applying the BiCNN model inversion shows that the PM2.5 concentration is higher in 2020, but the overall air quality improves year by year. Seasonal evaluation results show that BiCNN has a significant advantage in PM2.5 estimation in autumn, with R2 reaching 0.883. The spatial distribution of PM2.5 shows a spatial pattern of higher in spring and winter, lower in summer and autumn, and transition in autumn. The BiCNN model integrates the advantages of Convolutional Neural Network (CNN) and Bidirectional Long Short-Term Memory Neural Network (BiLSTM), makes full use of historical and future data, and can effectively capture the temporal and spatial characteristics between cofactors and PM2.5 data. It has achieved ideal results in the PM2.5 estimation of the MRYRA and provides an effective scientific method for air pollution control in urban agglomerations.





Similar content being viewed by others
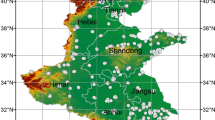
Data availability
All datasets, raw or preprocessed, are available upon request from the corresponding author.
References
Bai L, Jiang L, Chen Z (2018) Spatio-temporal characteristics of PM2.5 and its influencing factors of the urban agglomeration in the middle reaches of the Yangtze. Resour Environ Yangtze Basin 27(05):960–968
Beloconi A, Kamarianakis Y, Chrysoulakis N (2016) Estimating urban PM10 and PM2.5 concentrations, based on synergistic MERIS/AATSR aerosol observations, land cover and morphology data. Remote Sens Environ 172:148–164
Bengio Y, Simard P, Frasconi P (1994) Learning long-term dependencies with gradient descent is difficult. IEEE Trans Neural Netw 5(2):157–166
Buoli M, Grassi S, Caldiroli A, Carnevali GS, Mucci F, Iodice S et al (2018) Is there a link between air pollution and mental disorders? Environ Int 118:154–168
Dai H, Huang G, Wang J, Zeng H (2023) VAR-tree model based spatio-temporal characterization and prediction of O3 concentration in China. Ecotoxicol Environ Saf 257:114960
Dai H, Huang G, Zeng H, Yu R (2022) Haze risk assessment based on improved PCA-MEE and ISPO-LightGBM model. Systems 10:263
Engel-Cox J, Oanh NTK, van Donkelaar A, Martin RV, Zell E (2013) Toward the next generation of air quality monitoring: particulate matter. Atmos Environ 80:584–590
Fang C (2014) Progress and the future direction of research into urban agglomeration in China. Acta Geographica Sinica 69(8):1130–1144
Fang X, Zou B, Liu X, Sternberg T, Zhai L (2016) Satellite-based ground PM2.5 estimation using timely structure adaptive modeling. Remote Sens Environ 186:152–163
Gers FA, Schmidhuber J, Cummins F (2000) Learning to forget: continual prediction with LSTM. Neural Comput 12(10):2451–2471
Green M, Kondragunta S, Ciren P, Xu C (2009) Comparison of GOES and MODIS aerosol optical depth (AOD) to aerosol robotic network (AERONET) AOD and IMPROVE PM2.5 mass at Bondville, Illinois. J Air Waste Manage Assoc 59(9):1082–1091
Gu J, Wang Z, Kuen J, Ma L, Shahroudy A, Shuai B et al (2018) Recent advances in convolutional neural networks. Pattern Recogn 77:354–377
Gupta P, Christopher SA (2009) Particulate matter air quality assessment using integrated surface, satellite, and meteorological products: multiple regression approach. J Geophys Res Atmos 114(D14)
Han M, Jia S, Zhang C (2022) Estimation of high-resolution PM2.5 concentrations based on gap-filling aerosol optical depth using gradient boosting model. Air Qual Atmos Health 15(4):619–631
Han Y, Lam JC, Li VO, Reiner D (2021) A Bayesian LSTM model to evaluate the effects of air pollution control regulations in Bei**g, China. Environ Sci Policy 115:26–34
Hao Y, Gao Q (2020) Predicting the trend of stock market index using the hybrid neural network based on multiple time scale feature learning. Appl Sci 10(11):3961
Jia S, Han M, Zhang C (2022) Long short-term memory network model to estimate PM2.5 concentrations with missing-filled satellite data in Bei**g. Stoch Environ Res Risk Assess 36(12):4175–4184
Jiang S, Kong S, Zheng H, Zeng X, Chen N, Deng S (2022) Real-time source apportionment of PM2.5 and potential geographic origins of each source during winter in Wuhan. Environmental. Science 43(01):61–73. https://doi.org/10.13227/j.hjkx.202105171
Kloog I, Chudnovsky AA, Just AC, Nordio F, Koutrakis P, Coull BA et al (2014) A new hybrid spatio-temporal model for estimating daily multi-year PM2.5 concentrations across northeastern USA using high resolution aerosol optical depth data. Atmos Environ 95:581–590
Kow PY, Wang YS, Zhou Y, Kao IF, Issermann M, Chang LC, Chang FJ (2020) Seamless integration of convolutional and back-propagation neural networks for regional multi-step-ahead PM2.5 forecasting. J Clean Prod 261:121285
Li Y, Hao Z, Lei H (2016) Survey of convolutional neural network. J Comput Appl 36(9):2508
Li T, Shen H, Yuan Q, Zhang X, Zhang L (2017a) Estimating ground-level PM2.5 by fusing satellite and station observations: a geo-intelligent deep learning approach. Geophys Res Lett 44(23):11–985
Li T, Shen H, Zeng C, Yuan Q, Zhang L (2017b) Point-surface fusion of station measurements and satellite observations for map** PM2.5 distribution in China: methods and assessment. Atmos Environ 152:477–489
Liang CS, Duan FK, He KB, Ma YL (2016) Review on recent progress in observations, source identifications and countermeasures of PM2.5. Environ Int 86:150–170
Liu C, Deng X, Zhu B, Duan C (2018) Characteristics of GSR of China’s three major economic regions in the past 10 years and its relationship with 03 and PM2.5. Environmental. Science 38(08):2820–2829
Liu L, Zhang Y, Li Y et al (2020) PM2.5 inversion using remote sensing data in eastern China based on deep learning. Environmental. Science 41(04):1513–1519. https://doi.org/10.13227/j.hjkx.201909209
Lv B, Hu Y, Chang HH, Russell AG, Bai Y (2016) Improving the accuracy of daily PM2.5 distributions derived from the fusion of ground-level measurements with aerosol optical depth observations, a case study in North China. Environ Sci Technol 50(9):4752–4759
Ma YR, Ji Q, Fan Y (2016) Spatial linkage analysis of the impact of regional economic activities on PM2.5 pollution in China. J Clean Prod 139:1157–1167
Ma Z, Hu X, Huang L, Bi J, Liu Y (2014) Estimating ground-level PM2. 5 in China using satellite remote sensing. Environ Sci Technol 48(13):7436–7444
Maji KJ, Ye WF, Arora M, Nagendra SS (2018) PM2.5-related health and economic loss assessment for 338 Chinese cities. Environ Int 121:392–403
Miao L, Tang S, Ren Y, Kwan MP, Zhang K (2022) Estimation of daily ground-level PM2.5 concentrations over the Pearl River Delta using 1 km resolution MODIS AOD based on multi-feature BiLSTM. Atmos Environ 290:119362
Ni X, Cao C, Zhou Y, Cui X, Singh PR (2018) Spatio-temporal pattern estimation of PM2.5 in Bei**g-Tian**-Hebei Region based on MODIS AOD and meteorological data using the back propagation neural network. Atmosphere 9(3):105
Peng J, Chen S, Lü H, Liu Y, Wu J (2016) Spatiotemporal patterns of remotely sensed PM2.5 concentration in China from 1999 to 2011. Remote Sens Environ 174:109–121
Shan L, Liu Y, Tang M, Yang M, Bai X (2021) CNN-BiLSTM hybrid neural networks with attention mechanism for well log prediction. J Petrol Sci Eng 205:108838
Shao Y, Lunetta RS (2012) Comparison of support vector machine, neural network, and CART algorithms for the land-cover classification using limited training data points. ISPRS J Photogramm Remote Sens 70:78–87
Tan R, Liu Y, Liu Y, He Q, Ming L, Tang S (2014) Urban growth and its determinants across the Wuhan urban agglomeration, central China. Habitat Int 44:268–281
Tecer LH, Alagha O, Karaca F, Tuncel G, Eldes N (2008) Particulate matter (PM2.5, PM10-2.5, and PM10) and children’s hospital admissions for asthma and respiratory diseases: a bidirectional case-crossover study. J Toxicol Environ Health A 71(8):512–520
Wen C, Liu S, Yao X, Peng L, Li X, Hu Y, Chi T (2019) A novel spatiotemporal convolutional long short-term neural network for air pollution prediction. Sci Total Environ 654:1091–1099
Wu S, Li H (2022) Prediction of PM2. 5 concentration in urban agglomeration of China by hybrid network model. J Clean Prod 374:133968
**a C, Zhang A, Wang H, Zhang B, Zhang Y (2019) Bidirectional urban flows in rapidly urbanizing metropolitan areas and their macro and micro impacts on urban growth: a case study of the Yangtze River middle reaches megalopolis, China. Land Use Policy 82:158–168
Xue T, Zheng Y, Tong D, Zheng B, Li X, Zhu T, Zhang Q (2019) Spatiotemporal continuous estimates of PM2.5 concentrations in China, 2000–2016: a machine learning method with inputs from satellites, chemical transport model, and ground observations. Environ Int 123:345–357
Zhang H, Hoff RM, Engel-Cox JA (2009) The relation between Moderate Resolution Imaging Spectroradiometer (MODIS) aerosol optical depth and PM2.5 over the United States: a geographical comparison by US Environmental Protection Agency regions. J Air Waste Manage Assoc 59(11):1358–1369
Zhang T, He W, Zheng H, Cui Y, Song H, Fu S (2021) Satellite-based ground PM2.5 estimation using a gradient boosting decision tree. Chemosphere 268:128801
Zhang M, Tan S, Zhang Y, He J, Ni Q (2022) Does land transfer promote the development of new-type urbanization? New evidence from urban agglomerations in the middle reaches of the Yangtze River. Ecol Indic 136:108705
Zhao R, Wang D, Yan R, Mao K, Shen F, Wang J (2017) Machine health monitoring using local feature-based gated recurrent unit networks. IEEE Trans Ind Electron 65(2):1539–1548
Zheng H, Kong S, Chen N, Yan Y, Liu D, Zhu B et al (2020) Significant changes in the chemical compositions and sources of PM2.5 in Wuhan since the city lockdown as COVID-19. Sci Total Environ 739:140000
Zhong J, Zhang X, Gui K, Liao J, Fei Y, Jiang L et al (2022) Reconstructing 6-hourly PM 2.5 datasets from 1960 to 2020 in China. Earth Syst Sci Data 14(7):3197–3211
Zhou D, Zhang S, Yan J, Wu Y, Wu Q, Tao F (2022) Spatiotemporal distribution characteristics of PM2.5 and its influencing factors of the three urbanagglomerations in the Yangtze River Economic Belt. Resour Environ Yangtze Basin 31(04):878–889
Funding
This work is supported by Key Project of Natural Science Foundation of Jiangxi Province (20232ACB203025), Ministry of Education Project (202102245015), and Special Fund for Graduate Innovation (No. YC2022-S637).
Author information
Authors and Affiliations
Contributions
Study conception and design: SW and YH; data collection and processing: SW; methodology: SW, YH and YBZ; analysis of results and discussion: SW, YH, and HL; and draft manuscript preparation: SW, YH, HL, and YZ. All authors have read and agreed to the published version of the manuscript.
Corresponding authors
Ethics declarations
Competing interests
The authors declare that they have no known competing financial interests or personal relationships that could have appeared to influence the work reported in this paper.
Additional information
Publisher’s Note
Springer Nature remains neutral with regard to jurisdictional claims in published maps and institutional affiliations.
Rights and permissions
Springer Nature or its licensor (e.g. a society or other partner) holds exclusive rights to this article under a publishing agreement with the author(s) or other rightsholder(s); author self-archiving of the accepted manuscript version of this article is solely governed by the terms of such publishing agreement and applicable law.
About this article
Cite this article
Wu, S., Li, H., Zhou, Y. et al. PM2.5 estimation and analysis of BiCNN model considering spatiotemporal characteristics: a case study of the middle reaches of the Yangtze River urban agglomeration. Theor Appl Climatol 155, 2787–2799 (2024). https://doi.org/10.1007/s00704-023-04775-9
Received:
Accepted:
Published:
Issue Date:
DOI: https://doi.org/10.1007/s00704-023-04775-9