Abstract
The output power of the fuel cell depends on the operating conditions, such as the temperature and membrane water content. Therefore, a robust maximum power point tracking (MPPT) approach is highly required to ensure the operation of the proton exchange membrane fuel cell (PEMFC) at the maximum power point. A new hybrid perturb & observe and type-2 fuzzy-based adaptive step size MPPT for PEMFC system is proposed to enhance the dynamic response and eliminates the oscillations around maximum power point at steady state. The type-2 fuzzy approach has been used to scale the variable step size of conventional perturb & observe algorithm to extract the maximum available power of the PEMFC system. The proposed hybrid controller has been tested and validated using MATLAB software via modeling of the fuel cell power system composed of 7 kW PEMFC powering a resistive load through a DC-DC boost converter. Comparative simulation results prove that our proposed hybrid P&O type-2 fuzzy-based MPPT reduces the response time between 14.62 and 84.72% compared to the P&O type-1 fuzzy-based MPPT. The response time is also reduced between 43.99 and 88.04% compared to the conventional P&O fixed step size MPPT. In addition, the proposed controller decreases the steady-state oscillation to an interval of 66.67% to 70.97% compared to the P&O type-1 fuzzy-based MPPT and an interval of 72.73% to 84.85% compared to the conventional P&O fixed step size MPPT.
















Similar content being viewed by others
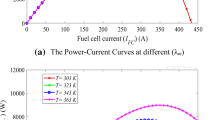
Abbreviations
- FC:
-
Fuel cell
- FLC:
-
Fuzzy logic controller
- IC:
-
Incremental conductance
- MPP:
-
Maximum power point
- MPPT:
-
Maximum power point tracking
- PSO:
-
Particle swarm optimization
- P&O:
-
Perturbation and observation
- PID:
-
Proportional integral derivative
- PEM:
-
Proton exchange membrane
- SMC:
-
Sliding mode control
- μ act :
-
Activation voltage
- C :
-
Capacitance
- C O2 :
-
Concentration of dissolved oxygen
- μ con :
-
Concentration voltage
- I :
-
Current
- D :
-
Duty cycle
- F :
-
Faraday’s constant
- I FC :
-
Fuel cell current
- υ FC :
-
Fuel cell voltage
- P H2 :
-
Hydrogen pressure
- L :
-
Inductance
- ΔI L :
-
Inductor current ripple
- I max :
-
Maximum current density
- A :
-
Membrane active area
- R M :
-
Membrane resistance
- Φ:
-
Membrane water content
- E nernst :
-
Nernst voltage
- κ:
-
Number of electrons
- μ ohm :
-
Ohmic voltage
- ΔυO:
-
Output voltage ripple
- P O2 :
-
Oxygen pressure
- ξ i = 1 – 4 :
-
Parametric coefficients
- P :
-
Power
- R c :
-
Equivalent resistance of electron
- Fs:
-
Switching frequency
- T :
-
Temperature
- λ :
-
Membrane thickness
- R :
-
Universal gas constant
- dI :
-
Variation in current
- dP :
-
Variation in power
- dV :
-
Variation in voltage
- E :
-
Error
- ΔE :
-
Change in error
References
Qi A (2014) Proton exchange membrane fuel cells. CRC Press, Boca Raton
Sarvi M, Barati MM (2010) Voltage and current based MPPT of fuel cells under variable temperature conditions. In: 45th international universities power engineering conference UPEC2010. IEEE, pp 1–4
Karami N, Outbib R, Moubayed N (2012) Fuel flow control of a PEM Fuel Cell with MPPT. In: 2012 IEEE international symposium on intelligent control. IEEE, pp 289–294
Becherif M, Hissel D (2010) MPPT of a PEMFC based on air supply control of the motocompressor group. Int J Hydrogen Energy 35(22):12521–12530
Venkateshkumar M, Sarathkumar G, Britto S (2013) Intelligent control based MPPT method for fuel cell power system. In: International conference on renewable energy and sustainable energy ICRESE. IEEE, pp 253–257
Li D, Yu Y, ** Q, Gao Z (2014) Maximum power efficiency operation and generalized predictive control of PEM (proton exchange membrane) fuel cell. Energy 68:210–217
Ríos R, Ramos-Paja CA, Espinosa JJ (2016) A control system for reducing the hydrogen consumption of PEM fuel cells under parametric uncertainties. TecnoLógicas 19(37):45–59
Chen FX, Yu Y, Chen JX (2017) Control system design of power tracking for PEM fuel cell automotive application. Fuel Cells 17(5):671–681
Karami N, ElKhoury L, Khoury G, Moubayed N (2014) Comparative study between P&O and incremental conductance for fuel cell MPPT. In: International conference on renewable energies for develo** countries 2014. IEEE, pp 17–22
Fathabadi H (2016) Novel highly accurate universal maximum power point tacker for maximum power extraction from hybrid fuel cell/photovoltaic/wind power generation system. Energy 116:402–416. https://doi.org/10.1016/j.energy.2016.09.095
Habib M, Khoucha F, Harrag A (2017) GA-based robust LQR controller for interleaved boost DC-DC converter improving fuel cell voltage regulation. Electr Power Syst Res 152:438
Fathabadi H (2017) Novel fast and high accuracy maximum power point tracking method for hybrid photovoltaic/fuel cell energy conversion systems. Renew Energy 106:232–242. https://doi.org/10.1016/j.renene.2017.01.028
Harrag A, Messalti S (2017) Variable step size IC MPPT controller for PEMFC power system improving static and dynamic performances. Fuel Cells 17(6):816–824
Harrag A, Bahri H (2017) Novel neural network IC-based variable step size fuel cell MPPT controller: performance, efficiency and lifetime improvement. Int J Hydrogen Energy 42(5):3549–3563
Reddy KJ, Sudhakar N (2018) High voltage gain interleaved boost converter with neural network based MPPT controller for fuel cell based electric vehicle applications. IEEE Access 6:3899–3908
Reddy KJ, Sudhakar N (2018) A new RBFN based MPPT controller for grid-connected PEMFC system with high step-up three-phase IBC. Int J Hydrogen Energy 43(37):17835–17848
Harrag A, Messalti S (2018) How fuzzy logic can improve PEM fuel cell MPPT performances? Int J Hydrogen Energy 43(1):537–550
Aliasghary M (2018) Control of PEM fuel cell systems using interval type-2 fuzzy PID approach. Fuel Cells 18(4):449–456. https://doi.org/10.1002/fuce.201700157
Harrag A, Bahri H (2019) A novel single sensor variable step size maximum power point tracking for proton exchange membrane fuel cell power system. Fuel Cells 19(2):177–189. https://doi.org/10.1002/fuce.201800122
Luta DN, Raji AK (2019) Comparing fuzzy rule-based MPPT techniques for fuel cell stack applications. Energy Procedia 156:177–182. https://doi.org/10.1016/j.egypro.2018.11.124
Khan MJ, Mathew L (2019) Fuzzy logic controller-based MPPT for hybrid photo-voltaic/wind/fuel cell power system. Neural Comput Appl 31:6331–6344. https://doi.org/10.1007/s00521-018-3456-7
Gheisarnejad M, Boudjadar J, Khooban MH (2019) A new adaptive type-II fuzzy-based deep reinforcement learning control: fuel cell air-feed sensors control. IEEE Sens J 19(20):9081–9089. https://doi.org/10.1109/JSEN.2019.2924726
Silaa MY, Derbeli M, Barambones O, Cheknane A (2020) Design and implementation of high order sliding mode control for PEMFC power system. Energies 13:4317–4331. https://doi.org/10.3390/en13174317
Derbedli M, Barambones O, Farhat M, Ramos-Hernanz JA, Sbita L (2020) Robust high order sliding mode control for performance improvement of PEM fuel cell power systems. Int J Hydrogen Energy 45(53):29222–29234. https://doi.org/10.1016/j.ijhydene.2020.07.172
Derbedli M, Barambones O, Silaa MY, Napole C (2020) Real-time implementation of a new MPPT control method for a DC-DC boost converter used in a PEM fuel cell power system. Actuators 9:105–126. https://doi.org/10.3390/act9040105
Li Q, Yang W, Yin L, Chen W (2020) Real-time implementation of maximum net power strategy based on sliding mode variable structure control for proton-exchange membrane fuel cell system. IEEE Trans Transp Electrif 6(1):288–297. https://doi.org/10.1109/TTE.2020.2970835
Ghousiya Begum K, Balasubramanian G (2020) Robust analytical proportional-integral-derivative tuning rules for regulation of air pressure in supply manifold of proton exchange membrane fuel cell. Asia-Pac J Chem Eng 2020:e2569. https://doi.org/10.1002/apj.2569
Bettayeb M, Mansouri R (2014) Fractional IMC-PID-filter controllers design for non integer order systems. J Process Control 24(4):261–271. https://doi.org/10.1016/j.jprocont.2014.01.014
Li D, Liu L, ** Q, Hirasawa K (2015) Maximum sensitivity based fractional IMC-PID controller design for non-integer order system with time delay. J Process Control 31:17–29. https://doi.org/10.1016/j.jprocont.2015.04.001
Rezk H, Fathy A (2020) Performance improvement of PEM fuel cell using variable step-size incremental resistance MPPT technique. Sustainability 12:5601–5616. https://doi.org/10.3390/su12145601
Fathy A, Abdelkareem MA, Olabi AG, Rezk H (2020) A novel strategy based on salp swarm algorithm for extracting the maximum power of proton exchange membrane fuel cell. Int J Hydrogen Energy. https://doi.org/10.1016/j.ijhydene.2020.02.165
Aggad F, Allaoui T, Tamer A, Denai M (2020) Modeling, design and energy management of a residential standalone photovoltaic-fuel cell power system. Przeglad Elektrotechniczny 96(8):79–87. https://doi.org/10.15199/48.2020.08.17
Liaqat K, Rehman Z, Ahmad I (2020) Nonlinear controllers for fuel cell, photovoltaic cell and battery based hybrid energy management system. J Energy Storage 32:101796. https://doi.org/10.1016/j.est.2020.101796
Abbaker AMO, Wang H, Tian Y (2020) Robust model-free adaptive interval type-2 fuzzy sliding mode control for PEMFC system using disturbance observer. Int J Fuzzy Syst 22:2188–2203
Wu D, Peng C, Yin C, Tang H (2020) Review of system integration and control of proton exchange membrane fuel cells. Electrochem Energy Rev 3:466–505. https://doi.org/10.1007/s41918-020-00068-1
O’hayre R, Cha S-W, Colella W, Prinz FB (2016) Fuel cell fundamentals. Wiley, New York
Mann RF, Amphlett JC, Hooper MAI, Jensen HM, Peppley BA, Roberge PR (2000) Development and application of a generalized steady-state electrochemical model for a PEM fuel cell. J Power Sources 86(1–2):173–180
Yu D, Yuvarajan S (2004) A novel circuit model for PEM fuel cells. In: Nineteenth annual IEEE applied power electronics conference and exposition, 2004. APEC'04. IEEE, pp 362–366
Lee JH, Lalk TR (1998) Modeling fuel cell stack systems. J Power Sources 73(2):229–241
Avelar HJ, Coelho EAA, Camacho JR, Vieira Júnior JB, Freitas LC, Wu M (2009) PEM fuel cell dynamic model for electronic circuit simulator. In: 2009 IEEE electrical power & energy conference (EPEC). IEEE, pp 1–6
Duman S, Yorukeren N, Altas IH (2018) A novel MPPT algorithm based on optimized artificial neural network by using FPSOGSA for standalone photovoltaic energy systems. Neural Comput Appl 29:257–278. https://doi.org/10.1007/s00521-016-2447-9
Shi K, Wang J, Tang Y, Zhong S (2020) Reliable asynchronous sampled-data filtering of T-S fuzzy uncertain delayed neural networks with stochastic switched topologies. Fuzzy Sets Syst 381:1–25
Luo J, Li M, Liu X, Tian W, Zhong S, Shi K (2020) Stabilization analysis for fuzzy systems with a switched sampled-data control. J Frankl Inst 357(1):39–58
Acknowledgements
The Algerian Ministry of Higher Education and Scientific Research via the DGRSDT supported this research (PRFU Project Code: A01L07UN190120180005).
Author information
Authors and Affiliations
Corresponding author
Ethics declarations
Conflict of interest
On behalf of all authors, the corresponding author states that there is no conflict of interest.
Additional information
Publisher's Note
Springer Nature remains neutral with regard to jurisdictional claims in published maps and institutional affiliations.
Rights and permissions
About this article
Cite this article
Harrag, A., Rezk, H. Indirect P&O type-2 fuzzy-based adaptive step MPPT for proton exchange membrane fuel cell. Neural Comput & Applic 33, 9649–9662 (2021). https://doi.org/10.1007/s00521-021-05729-w
Received:
Accepted:
Published:
Issue Date:
DOI: https://doi.org/10.1007/s00521-021-05729-w