Abstract
Objectives
Glioblastoma (GB) without peritumoral fluid-attenuated inversion recovery (FLAIR) hyperintensity is atypical and its characteristics are barely known. The aim of this study was to explore the differences in pathological and MRI-based intrinsic features (including morphologic and first-order features) between GBs with peritumoral FLAIR hyperintensity (PFH-bearing GBs) and GBs without peritumoral FLAIR hyperintensity (PFH-free GBs).
Methods
In total, 155 patients with pathologically diagnosed GBs were retrospectively collected, which included 110 PFH-bearing GBs and 45 PFH-free GBs. The pathological and imaging data were collected. The Visually AcceSAble Rembrandt Images (VASARI) features were carefully evaluated. The first-order radiomics features from the tumor region were extracted from FLAIR, apparent diffusion coefficient (ADC), and T1CE (T1-contrast enhanced) images. All parameters were compared between the two groups of GBs.
Results
The pathological data showed more alpha thalassemia/mental retardation syndrome X-linked (ATRX)-loss in PFH-free GBs compared to PFH-bearing ones (p < 0.001). Based on VASARI evaluation, PFH-free GBs had larger intra-tumoral enhancing proportion and smaller necrotic proportion (both, p < 0.001), more common non-enhancing tumor (p < 0.001), mild/minimal enhancement (p = 0.003), expansive T1/FLAIR ratio (p < 0.001) and solid enhancement (p = 0.009), and less pial invasion (p = 0.010). Moreover, multiple ADC- and T1CE-based first-order radiomics features demonstrated differences, especially the lower intensity heterogeneity in PFH-free GBs (for all, adjusted p < 0.05).
Conclusions
Compared to PFH-bearing GBs, PFH-free ones demonstrated less immature neovascularization and lower intra-tumoral heterogeneity, which would be helpful in clinical treatment stratification.
Clinical relevance statement
Glioblastomas without peritumoral FLAIR hyperintensity show less immature neovascularization and lower heterogeneity leading to potential higher treatment benefits due to less drug resistance and treatment failure.
Key Points
• The study explored the differences between glioblastomas with and without peritumoral FLAIR hyperintensity.
• Glioblastomas without peritumoral FLAIR hyperintensity showed less necrosis and contrast enhancement and lower intensity heterogeneity.
• Glioblastomas without peritumoral FLAIR hyperintensity had less immature neovascularization and lower tumor heterogeneity.





Similar content being viewed by others
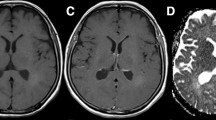
Abbreviations
- ADC:
-
Apparent diffusion coefficient
- ATRX:
-
Alpha thalassemia/mental retardation syndrome X-linked
- ECM:
-
Extracellular matrix
- FDR:
-
False discovery rate
- FLAIR:
-
Fluid-attenuated inversion recovery
- GB:
-
Glioblastoma
- ICC:
-
Intraclass correlation coefficient
- MAD:
-
Mean absolute deviation
- nCET:
-
Non-contrast enhancing tumor
- PFH:
-
Peritumoral FLAIR hyperintensity
- rMAD:
-
Robust mean absolute deviation
- ROC:
-
Receiver operator characteristics
- SNR:
-
Signal-to-noise ratio
- T1CE:
-
T1-contrast enhanced
- T1WI:
-
T1-weighted imaging
- T2WI:
-
T2-weighted imaging
- VASARI:
-
Visually AcceSAble Rembrandt Images
- VEGF:
-
Vascular endothelial growth factor
- VOI:
-
Volume of interest
References
Tate MC, Aghi MK (2009) Biology of angiogenesis and invasion in glioma. Neurotherapeutics 6:447–457
Carlson MR, Pope WB, Horvath S et al (2007) Relationship between survival and edema in malignant gliomas: role of vascular endothelial growth factor and neuronal pentraxin 2. Clin Cancer Res 13:2592–2598
Gerstner ER, Duda DG, di Tomaso E et al (2009) VEGF inhibitors in the treatment of cerebral edema in patients with brain cancer. Nat Rev Clin Oncol 6:229–236
Jain RK, Tong RT, Munn LL (2007) Effect of vascular normalization by antiangiogenic therapy on interstitial hypertension, peritumor edema, and lymphatic metastasis: insights from a mathematical model. Cancer Res 67:2729–2735
Lemée JM, Clavreul A, Menei P (2015) Intratumoral heterogeneity in glioblastoma: don’t forget the peritumoral brain zone. Neuro Oncol 17:1322–1332
Miroshnikova YA, Mouw JK, Barnes JM et al (2016) Tissue mechanics promote IDH1-dependent HIF1α-tenascin C feedback to regulate glioblastoma aggression. Nat Cell Biol 18:1336–1345
Shiroishi MS, Boxerman JL, Pope WB (2016) Physiologic MRI for assessment of response to therapy and prognosis in glioblastoma. Neuro Oncol 18:467–478
Qin X, Liu R, Akter F et al (2021) Peri-tumoral brain edema associated with glioblastoma correlates with tumor recurrence. J Cancer 12:2073–2082
Jain RK, di Tomaso E, Duda DG, Loeffler JS, Sorensen AG, Batchelor TT (2007) Angiogenesis in brain tumours. Nat Rev Neurosci 8:610–622
Michaelsen SR, Urup T, Olsen LR, Broholm H, Lassen U, Poulsen HS (2018) Molecular profiling of short-term and long-term surviving patients identifies CD34 mRNA level as prognostic for glioblastoma survival. J Neurooncol 137:533–542
Chen WJ, He DS, Tang RX, Ren FH, Chen G (2015) Ki-67 is a valuable prognostic factor in gliomas: evidence from a systematic review and meta-analysis. Asian Pac J Cancer Prev 16:411–420
Chaurasia A, Park SH, Seo JW, Park CK (2016) Immunohistochemical analysis of ATRX, IDH1 and p53 in glioblastoma and their correlations with patient survival. J Korean Med Sci 31:1208–1214
Wiestler B, Capper D, Holland-Letz T et al (2013) ATRX loss refines the classification of anaplastic gliomas and identifies a subgroup of IDH mutant astrocytic tumors with better prognosis. Acta Neuropathol 126:443–451
Mazurowski MA, Desjardins A, Malof JM (2013) Imaging descriptors improve the predictive power of survival models for glioblastoma patients. Neuro Oncol 15:1389–1394
Zhou M, Scott J, Chaudhury B et al (2018) Radiomics in brain tumor: image assessment, quantitative feature descriptors, and machine-learning approaches. AJNR Am J Neuroradiol 39:208–216
Kickingereder P, Burth S, Wick A et al (2016) Radiomic profiling of glioblastoma: identifying an imaging predictor of patient survival with improved performance over established clinical and radiologic risk models. Radiology 280:880–889
Gourtsoyianni S, Doumou G, Prezzi D et al (2017) Primary rectal cancer: repeatability of global and local-regional MR imaging texture features. Radiology 284:552–561
O’Connor JP, Rose CJ, Waterton JC, Carano RA, Parker GJ, Jackson A (2015) Imaging intratumor heterogeneity: role in therapy response, resistance, and clinical outcome. Clin Cancer Res 21:249–257
Parikh J, Selmi M, Charles-Edwards G et al (2014) Changes in primary breast cancer heterogeneity may augment midtreatment MR imaging assessment of response to neoadjuvant chemotherapy. Radiology 272:100–112
Dvorak HF (2003) Rous-Whipple award lecture. How tumors make bad blood vessels and stroma. Am J Pathol 162:1747–1757
Bellail AC, Hunter SB, Brat DJ, Tan C, Van Meir EG (2004) Microregional extracellular matrix heterogeneity in brain modulates glioma cell invasion. Int J Biochem Cell Biol 36:1046–1069
Mazaheri Y, Vargas HA, Nyman G, Shukla-Dave A, Akin O, Hricak H (2013) Diffusion-weighted MRI of the prostate at 3.0 T: comparison of endorectal coil (ERC) MRI and phased-array coil (PAC) MRI-The impact of SNR on ADC measurement. Eur J Radiol 82:e515-520
van Griethuysen JJM, Fedorov A, Parmar C et al (2017) Computational radiomics system to decode the radiographic phenotype. Cancer Res 77:e104–e107
Schoenegger K, Oberndorfer S, Wuschitz B et al (2009) Peritumoral edema on MRI at initial diagnosis: an independent prognostic factor for glioblastoma? Eur J Neurol 16:874–878
Liu XY, Gerges N, Korshunov A et al (2012) Frequent ATRX mutations and loss of expression in adult diffuse astrocytic tumors carrying IDH1/IDH2 and TP53 mutations. Acta Neuropathol 124:615–625
Wang K, Wang Y, Fan X et al (2016) Radiological features combined with IDH1 status for predicting the survival outcome of glioblastoma patients. Neuro Oncol 18:589–597
Stadlbauer A, Mouridsen K, Doerfler A et al (2018) Recurrence of glioblastoma is associated with elevated microvascular transit time heterogeneity and increased hypoxia. J Cereb Blood Flow Metab 38:422–432
Evans SM, Judy KD, Dunphy I et al (2004) Hypoxia is important in the biology and aggression of human glial brain tumors. Clin Cancer Res 10:8177–8184
Badr CE, Silver DJ, Siebzehnrubl FA, Deleyrolle LP (2020) Metabolic heterogeneity and adaptability in brain tumors. Cell Mol Life Sci 77:5101–5119
Patel AP, Tirosh I, Trombetta JJ et al (2014) Single-cell RNA-seq highlights intratumoral heterogeneity in primary glioblastoma. Science 344:1396–1401
Davnall F, Yip CS, Ljungqvist G et al (2012) Assessment of tumor heterogeneity: an emerging imaging tool for clinical practice? Insights Imaging 3:573–589
Kang Y, Choi SH, Kim YJ et al (2011) Gliomas: histogram analysis of apparent diffusion coefficient maps with standard- or high-b-value diffusion-weighted MR imaging–correlation with tumor grade. Radiology 261:882–890
Abdel Wahab C, Jannot AS, Bonaffini PA et al (2020) Diagnostic algorithm to differentiate benign atypical leiomyomas from malignant uterine sarcomas with diffusion-weighted MRI. Radiology 297:361–371
Roy S, Whitehead TD, Quirk JD et al (2020) Optimal co-clinical radiomics: sensitivity of radiomic features to tumour volume, image noise and resolution in co-clinical T1-weighted and T2-weighted magnetic resonance imaging. EBioMedicine 59:102963
Mahesparan R, Read TA, Lund-Johansen M, Skaftnesmo KO, Bjerkvig R, Engebraaten O (2003) Expression of extracellular matrix components in a highly infiltrative in vivo glioma model. Acta Neuropathol 105:49–57
Pope WB, Mirsadraei L, Lai A et al (2012) Differential gene expression in glioblastoma defined by ADC histogram analysis: relationship to extracellular matrix molecules and survival. AJNR Am J Neuroradiol 33:1059–1064
Patel KS, Yao J, Raymond C et al (2020) Decorin expression is associated with predictive diffusion MR phenotypes of anti-VEGF efficacy in glioblastoma. Sci Rep 10:14819
Yang L, Qiu M, **a C et al (2019) Value of high-resolution DWI in combination with texture analysis for the evaluation of tumor response after preoperative chemoradiotherapy for locally advanced rectal cancer. AJR Am J Roentgenol 212: 1279–1286
Parekh VS, Jacobs MA (2017) Integrated radiomic framework for breast cancer and tumor biology using advanced machine learning and multiparametric MRI. NPJ Breast Cancer 3:43
PyRadiomics documentation.; Available from: https://pyradiomics.readthedocs.io/en/latest/features.html#module-adiomics.firstorder. Accessed 18 Mar 2022 – 18 Nov 2022
Dubois LG, Campanati L, Righy C et al (2014) Gliomas and the vascular fragility of the blood brain barrier. Front Cell Neurosci 8:418
Acknowledgments
We thank all authors who contributed to the present study.
Funding
This project was supported by Shanghai Municipal Science and Technology Major Project (No. 2018SHZDZX01) and ZJ Lab, Shanghai Center for Brain-Inspired Technology, Medical Engineering Fund of Fudan University (yg2021-029), Shanghai Sailing Program (Grant No. 21YF1404800), Youth Program of Special Project for Clinical Research of Shanghai Municipal Health Commission Health industry (Grant No. 20204Y0423) and Youth Medical Talents –Medical Imaging Practitioner Program (No.3030256001).
Author information
Authors and Affiliations
Corresponding authors
Ethics declarations
Guarantor
The scientific guarantor of this publication is Bo Yin.
Conflict of interest
The authors of this manuscript declare no relationships with any companies, whose products or services may be related to the subject matter of the article.
Statistics and biometry
No complex statistical methods were necessary for this paper.
Informed consent
This was a retrospective study and informed consent was waived from all subjects.
Ethical approval
This retrospective study was approved by the local ethics committee.
Study subjects or cohorts overlap
No study subjects or cohorts have been previously reported.
Methodology
• Retrospective
• case-control study
• performed at one institution
Additional information
Publisher's note
Springer Nature remains neutral with regard to jurisdictional claims in published maps and institutional affiliations.
Supplementary Information
Below is the link to the electronic supplementary material.
Rights and permissions
Springer Nature or its licensor (e.g. a society or other partner) holds exclusive rights to this article under a publishing agreement with the author(s) or other rightsholder(s); author self-archiving of the accepted manuscript version of this article is solely governed by the terms of such publishing agreement and applicable law.
About this article
Cite this article
Han, Q., Lu, Y., Wang, D. et al. Glioblastomas with and without peritumoral fluid-attenuated inversion recovery (FLAIR) hyperintensity present morphological and microstructural differences on conventional MR images. Eur Radiol 33, 9139–9151 (2023). https://doi.org/10.1007/s00330-023-09924-2
Received:
Revised:
Accepted:
Published:
Issue Date:
DOI: https://doi.org/10.1007/s00330-023-09924-2