Abstract
Objectives
To develop and compare radiomics model and fusion model based on multiple MR parameters for staging liver fibrosis in patients with chronic liver disease.
Materials and methods
Patients with chronic liver disease who underwent multiparametric abdominal MRI were included in this retrospective study. Multiparametric MR images were imported into 3D-Slicer software for drawing bounding boxes on MR images. By using a 3D-Slicer extension of SlicerRadiomics, radiomics features were extracted from these MR images. The z-score normalization method was used for post-processing radiomics features. The least absolute shrinkage and selection operator method (LASSO) was performed for selecting significant radiomics features. The logistic regression analysis was used for building the radiomics model. A fusion model was built by integrating serum fibrosis biomarkers of aspartate transaminase-to-platelet ratio index (APRI) and the fibrosis-4 index (FIB-4) with radiomics signatures.
Results
In the training cohort, AUCs of radiomics and fusion model were 0.707–0.842 and 0.718–0.854 for differentiating different groups. In the testing cohort, AUCs were 0.514–0.724 and 0.609–0.728. For the training cohort, there was no significant difference of AUCs between radiomics and fusion model (p > 0.05). For the testing cohort, AUCs of fusion model were higher than those of radiomics model in differentiating F1-3 vs. F4 and F1-2 vs. F4 (p = 0.011 & 0.042).
Conclusions
Radiomics model and fusion model based on multiparametric MRI exhibited the feasibility for staging liver fibrosis in patients with CLD, and APRI and FIB-4 could improve the diagnostic performance of radiomics model in differentiating F1-3 vs. F4 and F1-2 vs. F4.



Similar content being viewed by others
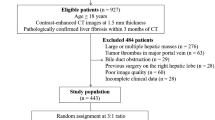
Abbreviations
- ECM:
-
Extracellular matrix
- MRE:
-
Magnetic resonance elastography
- RFI:
-
Radiomics fibrosis index
- AUC:
-
Area under the curves
- PACS:
-
Picture archiving and communication system
- TACE:
-
Transcatheter arterial chemoebolization
- APRI:
-
Aspartate transaminase-to-platelet ratio index
- FIB-4:
-
Fibrosis-4 index
- ROI:
-
Region of interest
- GLCM:
-
Gray level cooccurrence matrix
- GLDM:
-
Gray level dependence matrix
- GLRLM:
-
Gray level run length matrix
- GLSZM:
-
Gray level size zone matrix
- NGTDM:
-
Neighbouring gray tone difference matrix
- ICC:
-
Intraclass correlation coefficient
- LASSO:
-
Least absolute shrinkage and selection operator method
- CI:
-
Confidence interval
- CLD:
-
Chronic liver disease
References
Roehlen N, Crouchet E, Baumert TF (2020). Liver Fibrosis: Mechanistic Concepts and Therapeutic Perspectives. Cells 9:875
Caligiuri A, Gentilini A, Pastore M, et al (2021). Cellular and Molecular Mechanisms Underlying Liver Fibrosis Regression. Cells 10:2759
Rockey DC, Caldwell SH, Goodman ZD, et al (2009). Liver biopsy. Hepatology 49:1017–1044
Standish RA, Cholongitas E, Dhillon A, et al (2006). An appraisal of the histopathological assessment of liver fibrosis. Gut 55:569–578
Fu J, Wu B, Wu H, et al (2020). Accuracy of real-time shear wave elastography in staging hepatic fibrosis: a meta-analysis. BMC Med Imaging 20:16
Jiang W, Huang S, Teng H, et al (2018). Diagnostic accuracy of point shear wave elastography and transient elastography for staging hepatic fibrosis in patients with non-alcoholic fatty liver disease: a meta-analysis. BMJ Open 8:e021787
Ozturk A, Olson MC, Samir AE, Venkatesh SK (2022). Liver fibrosis assessment: MR and US elastography. Abdom Radiol (NY) 47:3037–3050
Kim YS, Jang YN, Song JS (2018). Comparison of gradient-recalled echo and spin-echo echo-planar imaging MR elastography in staging liver fibrosis: a meta-analysis. Eur Radiol 28:1709–1718
Manduca A, Bayly PJ, Ehman RL, et al (2021). MR elastography: Principles, guidelines, and terminology. Magn Reson Med 85:2377–2390
Lafata KJ, Wang Y, Konkel B, et al (2022). Radiomics: a primer on high-throughput image phenoty**. Abdom Radiol (NY) 47:2986–3002
Park HJ, Lee SS, Park B, et al (2019). Radiomics Analysis of Gadoxetic Acid-enhanced MRI for Staging Liver Fibrosis. Radiology 290:380–387
Zheng W, Guo W, **ong M, et al (2023). Clinic-radiological features and radiomics signatures based on Gd-BOPTA-enhanced MRI for predicting advanced liver fibrosis. Eur Radiol 33:633–644
Zhang D, Cao Y, Sun Y, et al (2023). Radiomics nomograms based on R2* map** and clinical biomarkers for staging of liver fibrosis in patients with chronic hepatitis B: a single-center retrospective study. Eur Radiol 33:1653–1667
Wei H, Shao Z, Fu F, et al (2023). Value of multimodal MRI radiomics and machine learning in predicting staging liver fibrosis and grading inflammatory activity. Br J Radiol 96:20220512
Bedossa P, Bioulacsage P, Callard P, et al (1994). Intraobserver and interobserver variations in liver biopsy interpretation in patients with chronic hepatitis C. Hepatology 20:15–20
Goodman ZD (2007). Grading and staging systems for inflammation and fibrosis in chronic liver diseases. J Hepatol 47:598–607
Wai CT, Greenson Jk, Fontana RJ, et al (2003). A simple noninvasive index can predict both significant fibrosis and cirrhosis in patients with chronic hepatitis C. Hepatology 38:518–526
Sterling RK, Lissen E, Clumeck N, et al (2006). Development of a simple noninvasive index to predict significant fibrosis in patients with HIV/HCV coinfection. Hepatology 43:1317–1325
Koo TK, Li MY (2016). A Guideline of Selecting and Reporting Intraclass Correlation Coefficients for Reliability Research. J Chiropr Med 15:155–163
**ao G, Yang J, Yan L (2015). Comparison of diagnostic accuracy of aspartate aminotransferase to platelet ratio index and fibrosis-4 index for detecting liver fibrosis in adult patients with chronic hepatitis B virus infection: a systemic review and meta-analysis. Hepatology 61:292–302
Kim WR, Berg T, Asselah T, et al (2016). Evaluation of APRI and FIB-4 scoring systems for non-invasive assessment of hepatic fibrosis in chronic hepatitis B patients. J Hepatol 64:773–780
Orlhac F, Lecler A, Savatovski J, et al (2021). How can we combat multicenter variability in MR radiomics? Validation of a correction procedure. Eur Radiol 31:2272–2280
Carre A, Battistella E, Niyoteka S, et al (2022). AutoComBat: a generic method for harmonizing MRI-based radiomic features. Sci Rep 12:12762
Acknowledgements
None.
Author information
Authors and Affiliations
Corresponding authors
Ethics declarations
Conflict of interest
The authors declare that the research was conducted in the absence of any commercial or financial relationships that could be construed as a potential conflict of interest.
Additional information
Publisher's Note
Springer Nature remains neutral with regard to jurisdictional claims in published maps and institutional affiliations.
Rights and permissions
Springer Nature or its licensor (e.g. a society or other partner) holds exclusive rights to this article under a publishing agreement with the author(s) or other rightsholder(s); author self-archiving of the accepted manuscript version of this article is solely governed by the terms of such publishing agreement and applicable law.
About this article
Cite this article
**ao, L., Zhao, H., Liu, S. et al. Staging liver fibrosis: comparison of radiomics model and fusion model based on multiparametric MRI in patients with chronic liver disease. Abdom Radiol 49, 1165–1174 (2024). https://doi.org/10.1007/s00261-023-04142-2
Received:
Revised:
Accepted:
Published:
Issue Date:
DOI: https://doi.org/10.1007/s00261-023-04142-2