Abstract
Emotional speech has some dependency on language or within a language itself, there are certain variations due to accents. The presence of accents degrades the performance of the speech emotion recognition (SER) system. A pre-trained accent identification system (AID) could effectively capture the characteristics of accent variations in emotional speech which is an important factor to develop a more reliable SER system. In this work, we investigate the dependencies between accent identification and emotion recognition to enhance the performance of SER. This paper proposes a novel transfer learning-based approach utilizing accent identification knowledge for SER. In the proposed method, the deep neural network (DNN) is used to model the accent identification system, which uses statistical aggregation functions (mean, std, median, etc.,) of spectral subband centroid (SSC) features and Mel-frequency discrete wavelet coefficients (MFDWC). To build the SER, the deep convolutional recurrent autoencoder produces the attention-based latent representation, and the acoustic features are extracted by the openSMILE toolkit. A separate DNN model is used to learn the map** between attention features and acoustic features for SER. In addition, the a priori knowledge of accent can lead the SER to effect the improvement which is possible through transfer learning (TL). The performance of the proposed method is assessed using the accented emotional speech utterances of the Crema-D dataset and also compared with state-of-the-art techniques. The experimental results show that transferring AID learning improves the recognition rate of the SER and results in around 8% relative improvement in accuracy as compared to the existing SER techniques.






Similar content being viewed by others
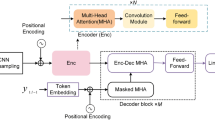
Data Availability
The dataset used in the current study is publically available open-source dataset.
References
G. Agarwal, H. Om, Performance of deer hunting optimization based deep learning algorithm for speech emotion recognition. Multimed. Tools Appl. 80, 9961–9992 (2021)
E.M. Albornoz, D.H. Milone, Emotion recognition in never-seen languages using a novel ensemble method with emotion profiles. IEEE Trans. Affect. Comput. 8, 43–53 (2017)
K. Amino, T. Osanai, Native vs. non-native accent identification using Japanese spoken telephone numbers. Speech Commun. 56, 70–81 (2014)
A. Burmania, C. Busso, A stepwise analysis of aggregated crowdsourced labels describing multimodal emotional behaviors. Proc. Interspeech 08, 152–156 (2017). https://doi.org/10.21437/Interspeech.2017-1278
H. Cao, D.G. Cooper, M.K. Keutmann, R.C. Gur, A. Nenkova, R. Verma, CREMA-D: crowd-sourced emotional multimodal actors dataset. IEEE Trans. Affect. Comput. 5, 377–390 (2014)
M. Chen, Z. Yang, H. Zheng, W. Liu, Improving native accent identification using deep neural networks, in Proceedings of the Annual Conference of the International Speech Communication Association. INTERSPEECH, pp. 2170–2174 (2014)
M. Chen, Z. Yang, J. Liang, Y. Li, W. Liu, Improving deep neural networks based multi-accent Mandarin speech recognition using i-vectors and accent-specific top layer (INTERSPEECH, 2015)
Y. Chen, Z.J. Yang, C.F. Yeh, Aipnet: generative adversarial pre-training of accent-invariant networks for end-to-end speech recognition, in IEEE International Conference on Acoustics, Speech and Signal Processing(ICASSP), pp. 6979–6983 (2020)
S. Chu, S. Narayanan, C.J. Kuo, Environmental sound recognition with time-frequency audio features. IEEE Trans. Audio Speech Lang. Process. 17, 1142–1158 (2009)
G.E. Dahl, D. Yu, L. Deng, A. Acero, Context-dependent pre-trained deep neural networks for large-vocabulary speech recognition. IEEE Trans. Audio Speech Lang. Process. 20, 30–42 (2012)
S. Das, N. Nadine Lønfeldt, A. Katrine Pagsberg, L.H. Clemmensen, Towards transferable speech emotion representation: on loss functions for cross-lingual latent representations, in ICASSP, pp. 6452–6456 (2022)
J. Deng, F. Jun, Z. Sascha, B. Schuller, Recognizing emotions from whispered speech based on acoustic feature transfer learning. IEEE Access 5, 5235–5246 (2017)
J. Deng, X. Xu, Z. Zhang, S. Frühholz, B. Schuller, Semisupervised autoencoders for speech emotion recognition. IEEE/ACM Trans. Audio Speech Lang. Process. 26, 31–43 (2018)
F. Eyben, M. Wollmer, B. Schuller, openSMILE: The Munich versatile and fast open-source audio feature extractor, in Proceedings of the 18th ACM International Conference on Multimedia, pp. 1459–1462 (2010)
H. Feng , S. Ueno, T. Kawahara, End-to-end speech emotion recognition combined with acoustic-to-word ASR model, in Proceedings of the Interspeech, pp. 501–505 (2020)
B. Gajic, K.K. Paliwal, Robust speech recognition in noisy environments based on subband spectral centroid histograms. IEEE Trans. Audio Speech Lang. Process. 14, 600–608 (2006)
J.N. Gowdy, Z. Tufekci, Mel-scaled discrete wavelet coefficients for speech recognition, in IEEE International Conference on Acoustics Speech and Signal Processing (ICASSP), pp. 1351–1354 (2000)
A. Hanani, M. Russell, M.J. Carey, Speech-based identification of social groups in a single accent of British English by humans and computers, in IEEE International Conference on Acoustics, Speech and Signal Processing (ICASSP), pp. 4876–4879 (2011)
Y.J. Hu, Z.H. Ling, Extracting spectral features using deep autoencoders with binary distributed hidden units for statistical parametric speech synthesis. IEEE/ACM Trans. Audio Speech Lang. Process. 26, 713–724 (2018)
R. Huang, J.H.L. Hansen, P. Angkititrakul, Dialect/accent classification using unrestricted audio. IEEE Trans. Audio Speech Lang. Process. 15, 453–464 (2007)
Z. Huang, S.M. Siniscalchi, C.H. Lee, A unified approach to transfer learning of deep neural networks with applications to speaker adaptation in automatic speech recognition. Neurocomputing 218, 448–459 (2016)
A. Ikeno, J. Hansen, The role of prosody in the perception of US native English accents. INTERSPEECH (2006)
S.G. Koolagudi, A. Barthwal, S. Devliyal, K.S. Rao, Real life emotion classification using spectral features and gaussian mixture models. Procedia Eng. 38, 3892–3899 (2012)
J.M.K. Kua, T. Thiruvaran, H. Nosrati, A.E., J. Epps, Investigation of spectral centroid magnitude and frequency for speaker recognition, in Odyssey: The Speaker and Language Recognition Workshop (2010)
W. Lin, K. Sridhar, C. Busso, Deepemocluster: a semi-supervised framework for latent cluster representation of speech emotions, in IEEE International Conference on Acoustics, Speech and Signal Processing (ICASSP), pp. 7263–7267 (2021)
L. Lu, D. Liu, H.J. Zhang, Automatic mood detection and tracking of music audio signals. IEEE Trans. Audio Speech Lang. Process. 14, 5–18 (2006)
H. Luo, J. Han, Nonnegative matrix factorization based transfer subspace learning for cross-corpus speech emotion recognition. IEEE/ACM Trans. Audio Speech Lang. Process. 28, 2047–2060 (2020)
X. Ma, Z. Wu, J. Jia, M. Xu, H.M. Meng, L. Cai, Emotion recognition from variable-length speech segments using deep learning on spectrograms, in INTERSPEECH (2018)
H. Ma, Z. Wang, X. Zhou, G. Zhou, Q. Zhou, Emotion recognition with conversational generation transfer. ACM Trans. Asian Low-Resour. Lang. Inf. Process. 21, 1–17 (2022)
K. Manohar, E. Logashanmugam, Hybrid deep learning with optimal feature selection for speech emotion recognition using improved meta-heuristic algorithm. Knowl. Based Syst. 246, 108659 (2022)
Q. Mao, M. Dong, Z. Huang, Y. Zhan, Learning salient features for speech emotion recognition using convolutional neural networks. IEEE Trans. Multimed. 16, 2203–2213 (2014)
M. Mauchand, M.D. Pell, Listen to my feelings! How prosody and accent drive the empathic relevance of complaining speech. Neuropsychologia 175, 108356 (2022)
M. Najafian, M. Russell, Automatic accent identification as an analytical tool for accent robust automatic speech recognition. Speech Commun. 122, 44–55 (2020)
T.L. Nwe, S.W. Foo, L.C.D. Silva, Speech emotion recognition using Hidden Markov Models. Speech Commun. 41, 603–623 (2003)
S. Niu, Y. Liu, J. Wang, H. Song, A decade survey of transfer learning (2010–2020). IEEE Trans. Artif. Intell. 1, 151–166 (2020). https://doi.org/10.1109/TAI.2021.3054609
J. Oliveira, I. Praça, On the usage of pre-trained speech recognition deep layers to detect emotions. IEEE Access 9, 9699–9705 (2021)
K.K. Paliwal, Spectral subband centroid features for speech recognition, in IEEE International Conference on Acoustics, Speech, and Signal Processing (ICASSP), pp. 617–620 (1998)
S.J. Pan, Q. Yang, A survey on transfer learning. IEEE Trans. Knowl. Data Eng. 22, 1345–1359 (2010). https://doi.org/10.1109/TKDE.2009.191
R. Pappagari, T. Wang, J. Villalba, N. Chen, N. Dehak, X-vectors meet emotions: a study on dependencies between emotion and speaker recognition, in IEEE International Conference on Acoustics, Speech and Signal Processing (ICASSP), pp. 7169–7173 (2020)
R. Pappagari, J. Villalba, P. Zelasko, M. Velazquez, D. Najim, Copypaste: an augmentation method for speech emotion recognition, in IEEE International Conference on Acoustics, Speech and Signal Processing (ICASSP), pp. 6324–6328 (2021)
A. Radoi, A. Birhala, N. Ristea, L. Dutu, An end-to-end emotion recognition framework based on temporal aggregation of multimodal information. IEEE Access 9, 135559–135570 (2021)
R. Rajoo, C.C. Aun, Influences of languages in speech emotion recognition: a comparative study using Malay, English and Mandarin languages. IEEE Symposium on Computer Applications & Industrial Electronics (ISCAIE), pp. 35–39 (2016)
V.M. Ramos, H.A.K. Hernandez-Diaz, M.E.H. Huici, H. Martens, G.V. Nuffelen, M.D. Bodt, Acoustic features to characterize sentence accent production in dysarthric speech. Biomed. Signal Process. Control 57, 101750 (2020)
K.S. Rao, Accent classification from an emotional speech in clean and noisy environments. Multimed. Tools Appl. 82, 3485–3508 (2023)
S. Saleem, F. Subhan, N. Naseer, A. Bais, A. Imtiaz, Forensic speaker recognition: a new method based on extracting accent and language information from short utterances. Forensic Sci. Int. Digit. Investig. 34, 300982 (2020)
B. Schuller, S. Steidl, A. Batliner, F. Burkhardt, L. Devillers, C. Müller, S. Narayanan, The INTERSPEECH 2010 paralinguistic challenge, in Proceedings of the 11th Annual Conference of the International Speech Communication Association, INTERSPEECH, pp. 2794–2797 (2010)
D. Seppi, A. Batliner, S. Steidl, B. Schuller, E. Nöth, Word accent and emotion, in Proceedings of the Speech Prosody, 053 (2010). https://doi.org/10.21437/SpeechProsody.2010-131
I. Shahin, N. Hindawi, A.B. Nassif, A. Alhudhaif, K. Polat, Novel dual-channel long short-term memory compressed capsule networks for emotion recognition. Expert Syst. Appl. 188, 116080 (2022)
G. Sharma, K. Umapathy, S. Krishnan, Trends in audio signal feature extraction methods. Appl. Acoust. 158, 107020 (2020)
J. Shen, R. Pang, R. J. Weiss, M. Schuster, N. Jaitly, Z. Yang, Z. Chen, Y. Zhang, Y. Wang, R. Skerrv-Ryan, R. A. Saurous, Y. Agiomvrgiannakis, Y. Wu, Natural TTS synthesis by conditioning wavenet on MEL spectrogram predictions, in IEEE International Conference on Acoustics, Speech and Signal Processing (ICASSP), pp. 4779–4783 (2018)
P.G. Shivakumar, P. Georgiou, Transfer learning from adult to children for speech recognition: evaluation, analysis and recommendations. Comput. Speech Lang. 63, 101077 (2020)
D. Snyder, D. Garcia-Romero, G. Sell, D. Povey, S. Khudanpur, X-Vectors: Robust DNN embeddings for speaker recognition, in IEEE International Conference on Acoustics, Speech and Signal Processing (ICASSP), pp. 5329–5333 (2018)
P. Song, W. Zheng, Feature selection based transfer subspace learning for speech emotion recognition. IEEE Trans. Affect. Comput. 11, 373–382 (2020)
S. Sun, C.F. Yeh, M.Y. Hwang, M. Ostendorf, L. **e, Domain adversarial training for accented speech recognition, in IEEE International Conference on Acoustics, Speech and Signal Processing (ICASSP), pp. 4854–4858 (2018)
Y. Sun, W. Sommer, W. Li, How accentuation influences the processing of emotional words in spoken language: an ERP study. Neuropsychologia 166, 108144 (2022)
N. Tabassum, T. Tabassum, F. Saad, T. Safa, H. Mahmud, M.K. Hasan, Exploring the English accent-independent features for speech emotion recognition using filter and wrapper-based methods for feature selection, in INTERSPEECH, pp. 3217–3221 (2023)
A. Vaswani, N. Shazeer, N. Parmar, J. Uszkoreit, L. Jones, A.N. Gomez, L. Kaiser, I. Polosukhin, Attention is all you need, in Proceedings of the 31st International Conference on Neural Information Processing Systems, pp. 6000–6010 (2017)
N. Vryzas, L. Vrysis, R. Kotsakis, C. Dimoulas, A web crowdsourcing framework for transfer learning and personalized speech emotion recognition. Mach. Learn. Appl. 6, 100132 (2021). https://doi.org/10.1016/j.mlwa.2021.100132
S. Waldekar, G. Saha, Classification of audio scenes with novel features in a fused system framework. Digit. Signal Process. 75, 71–82 (2018)
S. Waldekar, G. Saha, Wavelet transform based mel-scaled features for acoustic scene classification, in INTERSPEECH, pp. 3323–3327 (2018)
D. Wang, T.F. Zheng, Transfer learning for speech and language processing, in Asia-Pacific Signal and Information Processing Association Annual Summit and Conference (APSIPA), pp. 1225–1237 (2015)
Y. **a, L.W. Chen, A. Rudnicky, R. Stern, Temporal context in speech emotion recognition, in INTERSPEECH, pp. 3370–3374 (2021)
Z. Yao, Z. Wang, W. Liu, Y. Liu, J. Pan, Speech emotion recognition using fusion of three multi-task learning-based classifiers: {HSF-DNN}, {MS-CNN} and {LLD-RNN}. Speech Commun. 120, 11–19 (2020)
C. Yin, S. Zhang, J. Wang, N.N. **ong, Anomaly detection based on convolutional recurrent autoencoder for iot time series. IEEE Trans. Syst. Man Cybern. Syst. 52, 112–122 (2022)
C. Zhang, L. Xue, Autoencoder with emotion embedding for speech emotion recognition. IEEE Access 9, 51231–51241 (2021)
H. Zhang, Expression-EEG based collaborative multimodal emotion recognition using deep AutoEncoder. IEEE Access 8, 164130–164143 (2020)
Z. Zhao, Z. Bao, Y. Zhao, Z. Zhang, N. Cummins, Z. Ren, B. Schuller, Exploring deep spectrum representations via attention-based recurrent and convolutional neural networks for speech emotion recognition. IEEE Access 7, 97515–97525 (2019)
Z. Zhao, Q. Li, Z. Zhang, N. Cummins, H. Wang, J. Tao, B.W. Schuller, Combining a parallel 2D CNN with a self-attention Dilated Residual Network for CTC-based discrete speech emotion recognition. Neural Netw. 141, 52–60 (2021)
J. Zhong, P. Zhang, X. Li, Adaptive recognition of different accents conversations based on convolutional neural network. Multimed. Tools Appl. 78, 30749–30767 (2019)
Author information
Authors and Affiliations
Corresponding author
Ethics declarations
Conflict of interest
The authors declare that they have no conflict of interest.
Additional information
Publisher's Note
Springer Nature remains neutral with regard to jurisdictional claims in published maps and institutional affiliations.
Rights and permissions
Springer Nature or its licensor (e.g. a society or other partner) holds exclusive rights to this article under a publishing agreement with the author(s) or other rightsholder(s); author self-archiving of the accepted manuscript version of this article is solely governed by the terms of such publishing agreement and applicable law.
About this article
Cite this article
Priya Dharshini, G., Sreenivasa Rao, K. Transfer Accent Identification Learning for Enhancing Speech Emotion Recognition. Circuits Syst Signal Process (2024). https://doi.org/10.1007/s00034-024-02687-1
Received:
Revised:
Accepted:
Published:
DOI: https://doi.org/10.1007/s00034-024-02687-1