Abstract
Water mains which suffered from corrosive environment and various loads/effects. This leads to the simultaneous occurrence of the decrease of the pipe capacity and the appearance of significant bending moments within the pipe. Various investigation in literature focused on the stress of defected pipe due to burst pressure for oil and gas pipe under the high internal pressure, rather than the bended water mains. Primitive studies on this problem are at the observation step without providing an applicable model for practice. Since finding analytical solution for corrosion pipe is a challenging task because of the localized of the defects, the Finite Element Analysis (FEA) is an effective alternative. The critical drawback of FEA is that the problem needs to analysis separately with computational expense and required highly skilled experts. To ease these difficulties, a data-driven model is developed based on the database generated from FEAs and labeled by their results. The application of machine learning techniques improves the accuracy of the conventionally statistical regression models such as linear regression due to the flexible of the model and thus a trained data-driven model is a practical approach to solve the problem.
Access this chapter
Tax calculation will be finalised at checkout
Purchases are for personal use only
Similar content being viewed by others
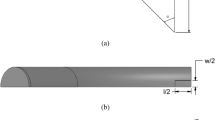
References
Bai, Y., Hauch, S.: Collapse capacity of corroded pipes under combined pressure, longitudinal force and bending. Int. J. Offshore Polar Eng. 11(01) (2001)
Balkaya, M., Moore, I.D., Sağlamer, A.: Study of nonuniform bedding support because of erosion under cast iron water distribution pipes. J. Geotech. Geoenviron. Eng. 138(10), 1247–1256 (2012)
Boser, B.E., Guyon, I.M., Vapnik, V.N.: A training algorithm for optimal margin classifiers. In: Proceedings of the Fifth Annual Workshop on Computational Learning Theory, pp. 144–152 (1992)
Breiman, L., Friedman, J., Olshen, R., Stone, C.: Classification and regression trees. Wadsworth & Brooks. Cole Statistics/Probability Series. (1984)
Carreira-Perpinan, M.A., Hinton, G.E.: On contrastive divergence learning. In: Aistats. Citeseer, pp. 33–40 (2005)
Chandra Mondal, B., Sutra Dhar, A.: Finite-element evaluation of burst pressure models for corroded pipelines. J. Press. Vessel Technol. 139(2) (2017)
Chen, Y.-F., Li, X., Zhou, J.: Ultimate bending capacity of pipe with arbitrary corrosion defects. Eng. Mech. 22(2), 43–49 (2009)
Chen, Y., Li, X., Chai, Y., Zhou, J.: Assessment of the flexural capacity of corroded steel pipes. Int. J. Press. Vessels Pip. 87(2–3), 100–110 (2010)
Cortes, C., Vapnik, V.: Support-vector networks. Mach. Learn. 20(3), 273–297 (1995)
Diniz, J., Vieira, R., Castro, J., Benjamin, A., Freire, J.: Stress and strain analysis of pipelines with localized metal loss. Exp. Mech. 46(6), 765–775 (2006)
Duong, H.T., Phan, H.C., Bui, N.D., Le, T.-T.: Optimization design of rectangular concrete-filled steel tube short columns with balancing composite motion optimization and data-driven model. Structures (2020) Accepted
Folkman, S.: Water main break rates in the USA and Canada: a comprehensive study (2018)
Friedman, J.H.: Greedy function approximation: a gradient boosting machine. Ann. Stat. 1189–1232 (2001)
Géron, A.: Hands-On Machine Learning with Scikit-Learn, Keras, and TensorFlow: Concepts, Tools, and Techniques to Build Intelligent Systems. O’Reilly Media (2019)
Hinton, G.E., Osindero, S., Teh, Y.-W.: A fast learning algorithm for deep belief nets. Neural Comput. 18(7), 1527–1554 (2006)
Jang, J.-S.: ANFIS: adaptive-network-based fuzzy inference system. IEEE Trans. Syst. Man Cybern. 23(3), 665–685 (1993)
Koy, C., Yune, C.-Y.: Numerical analysis on consolidation of soft clay by sand drain with heat injection. J. Korean Geotech. Soc. 33(11), 45–57 (2017)
LeCun, Y., Bengio, Y.: Convolutional networks for images, speech, and time series. Handb. Brain Theory Neural Netw. 3361(10), 1995 (1995)
Li, X., Bai, Y., Su, C., Li, M.: Effect of interaction between corrosion defects on failure pressure of thin wall steel pipeline. Int. J. Press. Vessels Pip. 138, 8–18 (2016)
Liu, J., Chauhan, V., Ng, P., Wheat, S., Hughes, C.: Remaining strength of corroded pipe under secondary (biaxial) loading, GL Industrial Services UK Ltd. (2009)
Liyanage, K.T., Dhar, A.S.: Effects of corrosion pits on wall stresses in cast-iron water mains. J. Pipeline Syst. Eng. Pract. 8(4), 04017023 (2017)
Ma, B., Shuai, J., Liu, D., Xu, K.: Assessment on failure pressure of high strength pipeline with corrosion defects. Eng. Fail. Anal. 32, 209–219 (2013)
Makar, J., Rogge, R., McDonald, S., Tesfamariam, S.: The effect of corrosion pitting on circumferential failures in grey iron pipes. American Water Works Association, Denver (2005)
Maraveas, C., Wang, Y., Swailes, T., Sotiriadis, G.: An experimental investigation of mechanical properties of structural cast iron at elevated temperatures and after cooling down. Fire Saf. J. 71, 340–352 (2015)
Matin, S., Farahzadi, L., Makaremi, S., Chelgani, S.C., Sattari, G.: Variable selection and prediction of uniaxial compressive strength and modulus of elasticity by random forest. Appl. Soft Comput. 70, 980–987 (2018)
McCulloch, W.S., Pitts, W.: A logical calculus of the ideas immanent in nervous activity. Bull. Math. Biophys. 5(4), 115–133 (1943)
Mondal, B.C., Dhar, A.S.: Burst pressure of corroded pipelines considering combined axial forces and bending moments. Eng. Struct. 186, 43–51 (2019)
Montáns, F.J., Chinesta, F., Gómez-Bombarelli, R., Kutz, J.N.: Data-driven modeling and learning in science and engineering. C. R. Méc. 347(11), 845–855 (2019)
Ni, P., Mangalathu, S., Yi, Y.: Fragility analysis of continuous pipelines subjected to transverse permanent ground deformation. Soils Found. 58(6), 1400–1413 (2018)
Oh, C.-K., Kim, Y.-J., Baek, J.-H., Kim, Y.-P., Kim, W.-S.: Ductile failure analysis of API X65 pipes with notch-type defects using a local fracture criterion. Int. J. Press. Vessels Pip. 84(8), 512–525 (2007)
Pham, T.D., Bui, N.D., Nguyen, T.T., Phan, H.C.: Predicting the reduction of embankment pressure on the surface of the soft ground reinforced by sand drain with random forest regression. In: IOP Conference Series: Materials Science and Engineering, pp. 072027. IOP Publishing (2020)
Ranzato, M.A., Huang, F.J., Boureau, Y.-L., LeCun, Y.: Unsupervised learning of invariant feature hierarchies with applications to object recognition. In: 2007 IEEE Conference on Computer Vision and Pattern Recognition, pp. 1–8. IEEE (2007)
Roy, S., Grigory, S., Smith, M., Kanninen, M., Anderson, M.: Numerical simulations of full-scale corroded pipe tests with combined loading (1997)
Sun, J., Shi, H., Jukes, P.: Upheaval buckling analysis of partially buried pipeline subjected to high pressure and high temperature. In: International Conference on Offshore Mechanics and Arctic Engineering, pp. 487–494 (2011)
Torii, A.J., Lopez, R.H.: Reliability analysis of water distribution networks using the adaptive response surface approach. J. Hydraul. Eng. 138(3), 227–236 (2012)
Trickey, S.A., Moore, I.D., Balkaya, M.: Parametric study of frost-induced bending moments in buried cast iron water pipes. Tunn. Undergr. Space Technol. 51, 291–300 (2016)
Vapnik, V.: Statistical Learning Theory. John Wiley&Sons. Inc., New York (1998)
Vazouras, P., Karamanos, S.A., Dakoulas, P.: Mechanical behavior of buried steel pipes crossing active strike-slip faults. Soil Dyn. Earthq. Eng. 41, 164–180 (2012)
Yu, W., Vargas, P.M., Karr, D.G.: Bending capacity analyses of corroded pipeline. J. Offshore Mech. Arct. Eng. 134(2) (2012)
Zheng, M., Luo, J., Zhao, X., Zhou, G., Li, H.: Modified expression for estimating the limit bending moment of local corroded pipeline. Int. J. Press. Vessels Pip. 81(9), 725–729 (2004)
Zhou, J., Shi, X., Du, K., Qiu, X., Li, X., Mitri, H.S.: Feasibility of random-forest approach for prediction of ground settlements induced by the construction of a shield-driven tunnel. Int. J. Geomech. 17(6), 04016129 (2017)
Acknowledgements
This research is funded by Vietnam National Foundation for Science and Technology Development (NAFOSTED) under grant number: 107.02-2020.04.
Author information
Authors and Affiliations
Corresponding author
Editor information
Editors and Affiliations
Rights and permissions
Copyright information
© 2022 The Author(s), under exclusive license to Springer Nature Singapore Pte Ltd.
About this paper
Cite this paper
Phan, H.C., Bui, N.D., Pham, T.D., Duong, H.T. (2022). Predicting Capacity of Defected Pipe Under Bending Moment with Data-Driven Model. In: Tien Khiem, N., Van Lien, T., Xuan Hung, N. (eds) Modern Mechanics and Applications. Lecture Notes in Mechanical Engineering. Springer, Singapore. https://doi.org/10.1007/978-981-16-3239-6_64
Download citation
DOI: https://doi.org/10.1007/978-981-16-3239-6_64
Published:
Publisher Name: Springer, Singapore
Print ISBN: 978-981-16-3238-9
Online ISBN: 978-981-16-3239-6
eBook Packages: EngineeringEngineering (R0)