Abstract
The determination of roll extrema distribution for ships and offshore structures is a matter of great concern. In order to take into account the nonlinearities of roll motion, a lot of effort is devoted to the evaluation of the linear and the nonlinear dam** coefficients. However, it should be noted that the way to use these coefficients in the overall design loop is equally important, and that inaccurate post-processing could spoil the effort invested in the evaluation of the linear and nonlinear roll dam**. The objective of this paper is to investigate different methods to assess roll extrema distribution under nonlinear roll dam**.
In this study, the original model to represent the roll response consists in an ordinary differential equation with a nonlinear (quadratic) dam**. All the other terms, such as the restoring force, are assumed linear through the whole paper. The time domain solution of this differential equation is the reference solution for the rest of the paper. Unfortunately, this resolution becomes CPU time consuming when long term roll value is required. Classically, a method of equivalent linearization is applied. This technique is well suited to frequency domain but unfortunately has a strong tendency to overestimate roll motions. To circumvent these issues, two different approaches (the Linearize & Match method introduced by Duthoit [3] and the equivalent design wave method) are presented, applied to two cases (a simple one degree of freedom illustrative case and a 6 degree of freedom realistic application case) and proved to give very good results for the estimation of the roll extrema distribution at almost no calculation cost.
Access this chapter
Tax calculation will be finalised at checkout
Purchases are for personal use only
Similar content being viewed by others
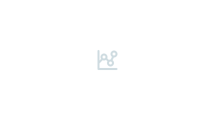
References
Armand, J.-L., Duthoit, C.: Distribution of maxima of nonlinear ship rolling. In: Price, W.G., Temarel, P., Keane, A.J. (ed.) Dynamics of Marine Vehicles and Structures in Waves. Elsevier Science Publishers (1991)
Cummins, W.E.: The Impulse Response Function and Ship Motions. Schiffstecnik
Duthoit, C.: Nonlinear Stochastic Response of Marine Vehicles. Ph.d. thesis of University of California, USA (1987)
Duthoit, C., Armand, J.-L.: Nonlinear dynamic behavior of moored platforms driven by stochastic seas. In: 6th OMAE Conference, Houston, Texas, USA (1987)
Isserlis, L.: On a formula for the product-moment coefficient of any order of a normal frequency distribution in any number of variables. Biometrika 12, 134–139 (1918)
Jensen, J.J.: Load and Global Response of Ships. Elsevier Ocean Engineering, vol. 4 (2001)
Mendoume Minko, I.D., Prevosto, M., Le Boulluec, M.: Distribution of maxima of non-linear rolling in case of coupled sway and roll motions of a floating body in irregular waves. In: 27th OMAE Conference, Estoril, Portugal (2008)
Prevosto, M.: Distribution of maxima of non-linear barge rolling with medium dam**. In: 11th ISOPE Conference, Stavanger, Norway (2001)
Rice, S.O.: Mathematical Analysis of Random Noice. Bell Systems Technical Journal
Tromans, P.S., Anaturk, A., Hagemerijer, P.: A new model for the kinematics of large ocean waves – application as a design wave. In: 1st ISOPE Conference, vol. III, Edinburgh, UK
Volterra, V.: Theory of Functionals and of Integral and Integro-Differential Equations. Dover Books on Mathematics (1930)
Explicit Runge-Kutta method of order (4) 5 due to Dormand and Prince (with stepsize control and dense output). https://docs.scipy.org/doc/scipy/reference/generated/scipy.integrate.ode.html#rcd9e153b6bcf-hnw93
Author information
Authors and Affiliations
Corresponding author
Editor information
Editors and Affiliations
7 Appendix 1
7 Appendix 1
The objective of this section is to show how the coefficients \( \beta_{n} \) are calculated.
1.1 7.1 Isserlis Theorem
If \( \left( {X_{1} , \ldots ,X_{2n} } \right) \) is a zero-mean multivariate normal random vector then:
where the notation ∑∏ means summing over all distinct ways of partitioning \( X_{1} X_{2} \ldots X_{2n} \) into pairs \( X_{i} X_{j} \) and each summand is the products of n pairs. This yields \( \frac{{\left( {2n} \right)!}}{{\left( {2^{n} n!} \right)}} = \left( {2n - 1} \right)!! \) terms in the sum.
1.2 7.2 Volterra Expansion
As mentioned in the Sect. 3.3, we introduce the Volterra expansion (which is essentially the convolution integral extended to nonlinear systems [11]):
where \( h_{1} , h_{3} \) denote the so-called Volterra kernels. The function \( C\left( t \right) \) represents the roll exciting moment (1). Due to the roll symmetries, the components associated with even Volterra kernels are equal to zero. In the following, we will not explicitly use the higher order Volterra kernel so the following notation will be enough:
Using this Volterra expansion, one gets the moment of order 2n:
In particular, one notices that in order to get an approximation to the order \( \varepsilon^{2n + 2} \), it is sufficient to determine \( {\mathbb{E}}\left[ {\theta_{1}^{2n - 1} \theta_{3} } \right] \).
1.3 7.3 Cubic System
We recall the form of the cubic nonlinear system to be solved:
By introducing the Volterra expansion, one gets:
The main idea of the following calculations is to isolate the expectation terms which contain the roll derivative. This is achieved by using the Isserlis theorem (7) for the developments down to \( {\mathbb{E}}\left[ {\theta^{2n - 4} } \right] \). In the following, we omit the variables (t) and (t-\( \tau \)) for \( \theta_{1} \) and \( \dot{\theta }_{1} \) in order to simplify the notation. Following Isserlis decomposition:
The term \( {\mathbb{E}}\left[ {\dot{\theta }\theta^{2n - 1} } \right] \) is studied hereafter:
The term \( {\mathbb{E}}\left[ {\dot{\theta }\dot{\theta }\theta^{2n - 2} } \right] \) is studied hereafter:
Finally, we have demonstrated following identity:
1.4 7.4 Equivalent Linear System (Sn) for the General Case N > 2
We recall the form of the linear system to be solved:
Note that here n > 2 (for the moment of order 2n).
By introducing the Volterra expansion, one gets:
Using the identity (12) which was demonstrated using Isserlis theorem [5], one gets the following expression:
1.5 7.5 Expressions Matching
The functions \( \theta_{1} \) and \( \theta_{n1} \) are equal since they are solutions of the same linear ODE. By identification of Eqs. (15) and (20), one obtains that:
1.6 7.6 Expression of βn Coefficients
Finally, the coefficients \( \beta_{n} \left( {n \ge 3} \right) \) can be written as follows:
where \( I_{2} , J_{2} \) and \( (\sigma_{\theta linear}^{2} , \sigma_{{\dot{\theta }linear}}^{2} ) \) are defined as follows:
Which corresponds to the linear transfer function of the cubic model with the cubic dam** equal to zero. It is to be noted that \( {\mathbb{E}}\left[ {{\dot{\theta }}^{2} } \right] \) corresponds to the solution of equivalent linear system (8). We have also:
As we have just mentioned above, it is to be noted that \( {\mathbb{E}}\left[ {{\dot{\theta }}^{2} } \right] \) corresponds to the solution of equivalent linear system (Eq. 2).
Rights and permissions
Copyright information
© 2021 Springer Nature Singapore Pte Ltd.
About this paper
Cite this paper
Diebold, L., de Hauteclocque, G. (2021). Investigation of Methods to Assess Roll Extrema Distribution Under Nonlinear Roll Dam**. In: Okada, T., Suzuki, K., Kawamura, Y. (eds) Practical Design of Ships and Other Floating Structures. PRADS 2019. Lecture Notes in Civil Engineering, vol 63. Springer, Singapore. https://doi.org/10.1007/978-981-15-4624-2_44
Download citation
DOI: https://doi.org/10.1007/978-981-15-4624-2_44
Published:
Publisher Name: Springer, Singapore
Print ISBN: 978-981-15-4623-5
Online ISBN: 978-981-15-4624-2
eBook Packages: EngineeringEngineering (R0)