AI-based prediction of protein–ligand binding affinity and discovery of potential natural product inhibitors against ERK2
Authors (first, second and last of 5)
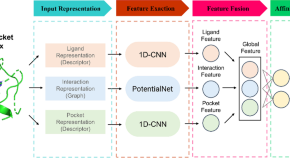
Collection
Dr. Marcus Tullius Scotti is an Associate Professor in the Chemistry Department of the Federal University of ParaÃba, since 2009. He received his Master's and Ph.D. degree in Organic Chemistry at the Chemistry Institute of the University of São Paulo. His research interests are cheminformatics applied to natural product databases, virtual screening, QSAR, and chemotaxonomy. He has published 308 papers, authored 19 book chapters, and edited two books.
Dr Renjith Thomas is the Head of the Department of Chemistry, and Director of the Centre for Theoretical and Computational Chemistry at St Berchmans College, Mahatma Gandhi University, India. He has contributed to theoretical and computational chemist research but his recent interest is in the evaluation of the nature of interaction between drug molecules and solvents and the development of a protocol to model the nature of drug-drug weak interactions. He was listed in the World’s top 2% scientists (2021-23) in Chemical Physics by Stanford University and Elsevier. He is elected as a fellow of the Royal Chemical Society, London.